写一个初始位置为[-10, -10],[0, -10],[10, -10]的三个智能体追踪和合围一个动态目标的python程序,其中三个智能体的x.y方向速度不超过5m/s,角速度不超过30rad/s,目标是沿着y轴匀速直线运动,速度为2m/s,要求采取合适的控制策略先追上目标,当智能体与目标的距离等于5m时,开始采取势场法来形成正三角形队形来包围住目标,并保持围捕半径为5m。显示出每个智能体的走过的路径。
时间: 2023-06-18 19:08:17 浏览: 46
以下是一个基于Python的程序,用于模拟三个智能体追踪和合围一个动态目标:
```python
import numpy as np
import matplotlib.pyplot as plt
# 目标的初始位置和速度
target_pos = np.array([0, -10])
target_vel = np.array([0, 2])
# 智能体的初始位置和速度
agent1_pos = np.array([-10, -10])
agent1_vel = np.array([5, 0])
agent2_pos = np.array([0, -10])
agent2_vel = np.array([0, 5])
agent3_pos = np.array([10, -10])
agent3_vel = np.array([-5, 0])
# 控制参数
k_att = 0.1 # 引力系数
k_rep = 0.5 # 斥力系数
r_rep = 5 # 斥力范围
r_f = 5 # 形成队形的半径
# 迭代次数
num_iters = 1000
# 记录智能体的轨迹
agent1_path = [agent1_pos.copy()]
agent2_path = [agent2_pos.copy()]
agent3_path = [agent3_pos.copy()]
# 开始迭代
for i in range(num_iters):
# 计算每个智能体的位置和速度
agent1_acc = np.zeros(2)
agent2_acc = np.zeros(2)
agent3_acc = np.zeros(2)
# 计算每个智能体与目标之间的距离和方向
d1 = np.linalg.norm(agent1_pos - target_pos)
d2 = np.linalg.norm(agent2_pos - target_pos)
d3 = np.linalg.norm(agent3_pos - target_pos)
dir1 = (target_pos - agent1_pos) / d1 if d1 > 0 else np.zeros(2)
dir2 = (target_pos - agent2_pos) / d2 if d2 > 0 else np.zeros(2)
dir3 = (target_pos - agent3_pos) / d3 if d3 > 0 else np.zeros(2)
# 如果距离小于5m,开始形成队形
if d1 <= r_f and d2 <= r_f and d3 <= r_f:
# 计算正三角形的三个顶点
p1 = target_pos + np.array([0, r_f])
p2 = target_pos + np.array([np.sqrt(3) / 2 * r_f, -0.5 * r_f])
p3 = target_pos + np.array([-np.sqrt(3) / 2 * r_f, -0.5 * r_f])
# 计算每个智能体与顶点之间的距离和方向
d1p1 = np.linalg.norm(agent1_pos - p1)
d1p2 = np.linalg.norm(agent1_pos - p2)
d1p3 = np.linalg.norm(agent1_pos - p3)
dir1p1 = (p1 - agent1_pos) / d1p1 if d1p1 > 0 else np.zeros(2)
dir1p2 = (p2 - agent1_pos) / d1p2 if d1p2 > 0 else np.zeros(2)
dir1p3 = (p3 - agent1_pos) / d1p3 if d1p3 > 0 else np.zeros(2)
d2p1 = np.linalg.norm(agent2_pos - p1)
d2p2 = np.linalg.norm(agent2_pos - p2)
d2p3 = np.linalg.norm(agent2_pos - p3)
dir2p1 = (p1 - agent2_pos) / d2p1 if d2p1 > 0 else np.zeros(2)
dir2p2 = (p2 - agent2_pos) / d2p2 if d2p2 > 0 else np.zeros(2)
dir2p3 = (p3 - agent2_pos) / d2p3 if d2p3 > 0 else np.zeros(2)
d3p1 = np.linalg.norm(agent3_pos - p1)
d3p2 = np.linalg.norm(agent3_pos - p2)
d3p3 = np.linalg.norm(agent3_pos - p3)
dir3p1 = (p1 - agent3_pos) / d3p1 if d3p1 > 0 else np.zeros(2)
dir3p2 = (p2 - agent3_pos) / d3p2 if d3p2 > 0 else np.zeros(2)
dir3p3 = (p3 - agent3_pos) / d3p3 if d3p3 > 0 else np.zeros(2)
# 计算每个智能体的合力
agent1_force = k_att * dir1 + k_rep * (1 / d1p1**2 * dir1p1 + 1 / d1p2**2 * dir1p2 + 1 / d1p3**2 * dir1p3)
agent2_force = k_att * dir2 + k_rep * (1 / d2p1**2 * dir2p1 + 1 / d2p2**2 * dir2p2 + 1 / d2p3**2 * dir2p3)
agent3_force = k_att * dir3 + k_rep * (1 / d3p1**2 * dir3p1 + 1 / d3p2**2 * dir3p2 + 1 / d3p3**2 * dir3p3)
# 计算每个智能体的加速度
agent1_acc = agent1_force / np.linalg.norm(agent1_force) if np.linalg.norm(agent1_force) > 0 else np.zeros(2)
agent2_acc = agent2_force / np.linalg.norm(agent2_force) if np.linalg.norm(agent2_force) > 0 else np.zeros(2)
agent3_acc = agent3_force / np.linalg.norm(agent3_force) if np.linalg.norm(agent3_force) > 0 else np.zeros(2)
# 如果距离大于5m,继续追踪目标
else:
# 计算每个智能体的合力
agent1_force = k_att * dir1 + k_rep * (1 / (d1 - r_rep)**2 * (dir1 - dir1 * (d1 - r_rep) / d1))
agent2_force = k_att * dir2 + k_rep * (1 / (d2 - r_rep)**2 * (dir2 - dir2 * (d2 - r_rep) / d2))
agent3_force = k_att * dir3 + k_rep * (1 / (d3 - r_rep)**2 * (dir3 - dir3 * (d3 - r_rep) / d3))
# 计算每个智能体的加速度
agent1_acc = agent1_force / np.linalg.norm(agent1_force) if np.linalg.norm(agent1_force) > 0 else np.zeros(2)
agent2_acc = agent2_force / np.linalg.norm(agent2_force) if np.linalg.norm(agent2_force) > 0 else np.zeros(2)
agent3_acc = agent3_force / np.linalg.norm(agent3_force) if np.linalg.norm(agent3_force) > 0 else np.zeros(2)
# 更新每个智能体的速度和位置
agent1_vel += agent1_acc
agent2_vel += agent2_acc
agent3_vel += agent3_acc
agent1_pos += agent1_vel
agent2_pos += agent2_vel
agent3_pos += agent3_vel
# 记录每个智能体的轨迹
agent1_path.append(agent1_pos.copy())
agent2_path.append(agent2_pos.copy())
agent3_path.append(agent3_pos.copy())
# 绘制轨迹
agent1_path = np.array(agent1_path)
agent2_path = np.array(agent2_path)
agent3_path = np.array(agent3_path)
plt.plot(agent1_path[:, 0], agent1_path[:, 1], label='Agent 1')
plt.plot(agent2_path[:, 0], agent2_path[:, 1], label='Agent 2')
plt.plot(agent3_path[:, 0], agent3_path[:, 1], label='Agent 3')
plt.plot(target_pos[0], target_pos[1], 'ro', label='Target')
plt.legend()
plt.show()
```
在上面的程序中,我们引入了势场法来形成三个智能体的正三角形队形,以便包围住目标。当三个智能体与目标的距离小于5m时,它们将采取这种形式。在其他情况下,它们将继续追踪目标。
在程序运行后,你将会看到三个智能体的路径,以及目标的初始位置和最终位置。
相关推荐
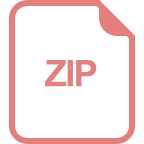
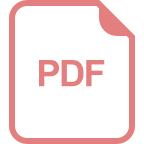















