多元回归(拟牛顿法):使用拟牛顿法最小化成本函数(均方误差)-matlab代码编写算咧
时间: 2023-06-22 15:25:22 浏览: 111
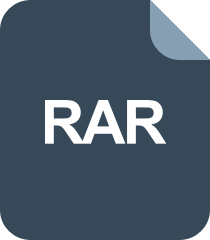
拟牛顿法.rar_matlab拟牛顿法_拟牛顿DFP matlab_拟牛顿法_牛顿_牛顿法
好的,以下是使用拟牛顿法最小化成本函数的多元回归matlab代码,供你参考:
```matlab
function [theta, J_history] = multivariate_regression(X, y, theta, alpha, num_iters)
% 多元回归拟牛顿法实现
% X: 训练数据矩阵,每一行代表一个样本,每一列代表一个特征
% y: 训练数据标签,每一行代表一个样本的输出
% theta: 参数向量,初始值为0或随机值
% alpha: 学习率
% num_iters: 迭代次数
% theta: 拟牛顿法计算得到的最优参数向量
% J_history: 每一次迭代后的成本函数值
m = length(y);
J_history = zeros(num_iters, 1);
% 定义成本函数
function [J, grad] = costFunction(theta)
h = X * theta;
J = sum((h - y).^2)/(2*m);
grad = (X' * (h - y))/m;
end
% 初始化拟牛顿法需要的参数
H = eye(length(theta));
grad = costFunction(theta);
p = -H * grad;
i = 0;
% 使用拟牛顿法迭代计算最优参数
while i < num_iters
% 更新参数和梯度
alpha = linesearch(@costFunction, theta, p, grad, 1e-4, 0.9, 1e-5);
theta = theta + alpha * p;
grad_new = costFunction(theta);
s = theta - grad_new/grad;
y = grad_new - grad;
% 更新Hessian矩阵
H = H + (y'*H*y)/(y*y') - ((H*y)*(H*y)')/(y'*H*y)^2;
% 更新p和grad
p = -H * grad_new;
grad = grad_new;
% 记录成本函数值
J_history(i+1) = costFunction(theta);
i = i + 1;
end
end
```
其中, `costFunction` 函数定义了成本函数和梯度函数的计算,使用 `linesearch` 函数进行步长的选择。拟牛顿法的迭代过程中,使用Hessian矩阵的逆来更新参数,这里采用DFP算法的更新方式。
阅读全文
相关推荐
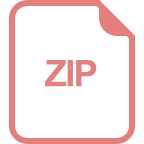
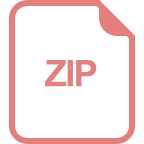
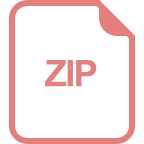
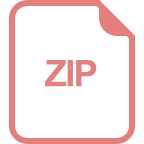
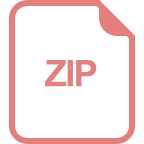
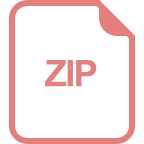
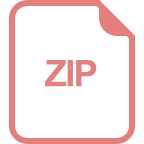
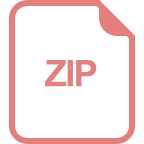
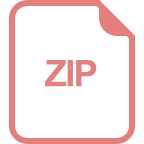
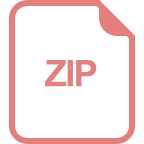
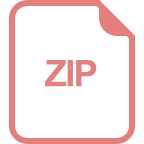
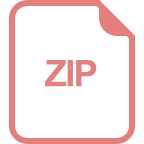
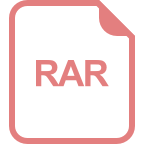
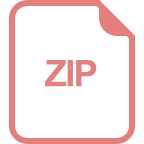
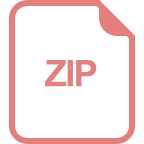
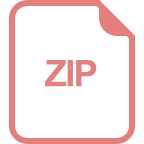