FileNotFoundError: train: D:\yolo\datasets\coco128\datasets\coco128\images\train does not exist
时间: 2024-03-15 15:39:59 浏览: 37
FileNotFoundError是一个Python异常,表示文件或目录不存在。在你提供的错误信息中,报告了一个文件不存在的错误,具体是train文件夹在路径D:\yolo\datasets\coco128\datasets\coco128\images\train下不存在。
可能的原因是:
1. 路径错误:请确保路径中的文件夹和文件名正确,并且没有拼写错误。
2. 文件或文件夹被移动或删除:如果你之前有这个文件或文件夹,但现在已经被移动或删除了,那么就会出现该错误。
3. 权限问题:如果你没有足够的权限来访问该文件或文件夹,也会导致该错误。
请检查以上可能的原因,并确保路径和文件名正确,并且文件或文件夹存在。如果问题仍然存在,请提供更多的信息,以便我能够更好地帮助你解决问题。
相关问题
FileNotFoundError: Image Not Found E:\yolo\vocc\train\images\常州 4698.JPG
FileNotFoundError是Python中的一个异常类型,表示文件未找到错误。在你提供的路径中,出现了一个名为"常州 4698.JPG"的图片文件未找到的错误。
可能的原因是:
1. 文件路径错误:请确保路径中的文件夹和文件名拼写正确,并且文件确实存在于指定路径中。
2. 文件不存在:检查一下指定路径中是否存在名为"常州 4698.JPG"的图片文件。如果文件不存在,可以尝试使用其他路径或者确保文件已经被正确地放置在指定路径中。
如果以上两个原因都排除了,还是出现了FileNotFoundError,那可能是其他问题导致的。你可以提供更多的信息,以便我能够更好地帮助你解决问题。
FileNotFoundError: Image Not Found E:\yolo\vocc\train\images\FFRC2238754鳜.jpg
FileNotFoundError是一个Python异常,表示文件未找到错误。在你提供的路径E:\yolo\vocc\train\images\FFRC2238754鳜.jpg中,出现了文件未找到的情况。
可能的原因是:
1. 文件路径错误:请确保路径中的文件夹和文件名正确,并且文件确实存在于该路径下。
2. 文件被移动或删除:如果文件被移动或删除,那么在指定的路径下就无法找到该文件。
请检查以上两个可能的原因,并确保文件存在于指定的路径下。如果文件确实存在,但仍然出现该错误,请提供更多详细信息以便我能够更好地帮助你解决问题。
相关推荐
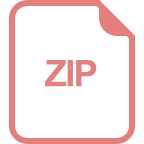












