svr支持向量机代码
时间: 2024-01-09 15:22:44 浏览: 77
SVR(支持向量回归)是一种机器学习算法,用于解决回归问题。它基于支持向量机(SVM)算法,通过寻找一个最优的超平面来拟合数据。
下面是一个简单的SVR代码示例:
```python
# 导入所需的库
from sklearn.svm import SVR
import numpy as np
# 创建训练数据
X = np.sort(5 * np.random.rand(100, 1), axis=0)
y = np.sin(X).ravel()
# 创建SVR模型并进行训练
svr = SVR(kernel='rbf', C=1e3, gamma=0.1)
svr.fit(X, y)
# 创建测试数据
X_test = np.arange(0.0, 5.0, 0.01)[:, np.newaxis]
# 使用SVR进行预测
y_pred = svr.predict(X_test)
# 打印预测结果
print(y_pred)
```
在这个示例中,我们首先导入了需要的库,然后创建了一些随机的训练数据。接下来,我们使用SVR模型进行训练,并创建了一些测试数据。最后,我们使用训练好的模型对测试数据进行预测,并打印出预测结果。
请注意,这只是一个简单的示例代码,实际使用SVR时可能需要根据具体问题进行参数调整和数据处理。
相关问题
svr支持向量机回归代码
以下是Python中使用SVR(支持向量机回归)的代码示例:
```python
# 导入所需的库
import numpy as np
import matplotlib.pyplot as plt
from sklearn.svm import SVR
# 生成数据
X = np.sort(5 * np.random.rand(80, 1), axis=0)
y = np.sin(X).ravel()
# 添加噪声
y[::5] += 3 * (0.5 - np.random.rand(16))
# 训练SVR模型
C = 1e3
svr_rbf = SVR(kernel='rbf', C=C, gamma=0.1)
svr_lin = SVR(kernel='linear', C=C)
svr_poly = SVR(kernel='poly', C=C, degree=3)
y_rbf = svr_rbf.fit(X, y).predict(X)
y_lin = svr_lin.fit(X, y).predict(X)
y_poly = svr_poly.fit(X, y).predict(X)
# 绘制结果
lw = 2
plt.scatter(X, y, color='darkorange', label='data')
plt.plot(X, y_rbf, color='navy', lw=lw, label='RBF model')
plt.plot(X, y_lin, color='c', lw=lw, label='Linear model')
plt.plot(X, y_poly, color='cornflowerblue', lw=lw, label='Polynomial model')
plt.xlabel('data')
plt.ylabel('target')
plt.title('Support Vector Regression')
plt.legend()
plt.show()
```
此代码使用了SVR来拟合一个带有噪声的正弦曲线,并绘制了不同内核的SVR的结果。在代码中,我们定义了三个不同的内核函数:RBF,线性和多项式。然后使用这些内核训练了三个不同的SVR模型,并将它们的预测结果绘制在同一个图表中。
svr支持向量机回归python代码
你好啊!关于支持向量机回归的 Python 代码,以下是一个简单的样例:
```
from sklearn.svm import SVR
# 创建一个支持向量机回归器
regressor = SVR(kernel='linear')
# 训练模型
X_train = [[0, 0], [2, 2]]
y_train = [0.5, 2.5]
regressor.fit(X_train, y_train)
# 使用模型进行预测
X_test = [[1, 1]]
y_pred = regressor.predict(X_test)
print(y_pred)
```
希望对您有所帮助!如果您有其他问题,请继续提问吧!
阅读全文
相关推荐



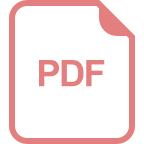
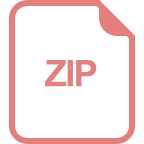
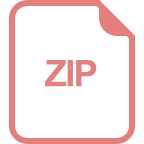
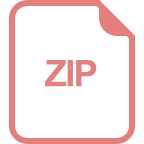
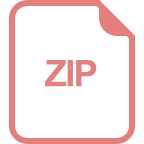
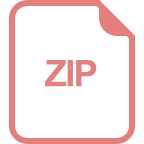
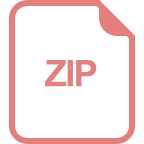
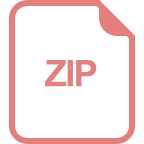
