GRU python
时间: 2023-12-27 12:24:48 浏览: 200
GRU(Gated Recurrent Unit)是一种循环神经网络(RNN)的变体,用于处理序列数据。它具有门控机制,可以更好地捕捉序列中的长期依赖关系。下面是一个使用GRU的Python示例:
```python
import tensorflow as tf
from tensorflow import keras
from tensorflow.keras import layers
# 定义输入形状
input_shape = (20, 5)
# 创建输入层
inputs = keras.Input(shape=input_shape)
# 添加GRU层
x = layers.GRU(16, activation='relu', return_sequences=True, kernel_regularizer=keras.regularizers.l2(0.01))(inputs)
# 创建模型
model = keras.Model(inputs=inputs, outputs=x)
# 打印模型结构
model.summary()
```
在这个例子中,我们首先创建了一个输入层,形状为(20, 5)。然后,我们使用`layers.GRU`函数添加了一个GRU层,其中包括16个单元,激活函数为ReLU,并且返回完整的序列。我们还使用了L2正则化来约束模型的权重。最后,我们创建了一个模型,并打印出模型的摘要。
相关问题
gru python
GRU(Gated Recurrent Unit)是一种循环神经网络(RNN)的变体,用于处理序列数据。它是LSTM(Long Short-Term Memory)的简化版本,通过使用门控机制来管理信息的流动。
在Python中,你可以使用库来实现GRU模型,例如TensorFlow或PyTorch。以下是使用PyTorch库实现GRU的示例代码:
```python
import torch
import torch.nn as nn
# 定义GRU模型
class GRUModel(nn.Module):
def __init__(self, input_size, hidden_size, num_layers, output_size):
super(GRUModel, self).__init__()
self.hidden_size = hidden_size
self.num_layers = num_layers
self.gru = nn.GRU(input_size, hidden_size, num_layers, batch_first=True)
self.fc = nn.Linear(hidden_size, output_size)
def forward(self, x):
h0 = torch.zeros(self.num_layers, x.size(0), self.hidden_size).to(device)
out, _ = self.gru(x, h0)
out = self.fc(out[:, -1, :])
return out
# 定义模型参数
input_size = 10
hidden_size = 20
num_layers = 2
output_size = 1
# 创建模型实例
model = GRUModel(input_size, hidden_size, num_layers, output_size)
# 输入数据
input_data = torch.randn(32, 100, input_size) # 输入数据维度为(batch_size, sequence_length, input_size)
# 模型前向传播
output = model(input_data)
```
以上是一个简单的GRU模型的实现例子,你可以根据需要进行修改和扩展。当然,还有其他的方式可以实现GRU模型,这只是其中一种方法。希望对你有所帮助!
GRU python代码
在Python中,你可以使用PyTorch库来创建和训练GRU(Gated Recurrent Unit)。这里有一个简单的例子,展示了如何初始化一个GRU层并使用它在一个循环神经网络(RNN)中:
```python
import torch
import torch.nn as nn
class GRUModel(nn.Module):
def __init__(self, input_size, hidden_size, num_layers=1, dropout=0.5):
super(GRUModel, self).__init__()
self.gru = nn.GRU(input_size, hidden_size, num_layers, dropout=dropout,
bidirectional=True) # 如果是双向GRU,则bidirectional设置为True
self.fc = nn.Linear(hidden_size * 2, output_size) # 输出层
def forward(self, x, h0):
# x: (batch_size, seq_len, input_size)
# h0: (num_layers * num_directions, batch_size, hidden_size)
# RNN期望的输入形状是(batch, seq_len, features),所以我们调整输入形状
x = x.permute(1, 0, 2)
# 运行GRU
out, _ = self.gru(x, h0) # _ 不返回最后一层的隐状态
# 将最后一个时间步的隐状态连接到全连接层
out = out[:, -1, :] # 获取seq_len的最后一项,因为这里是最后一层的隐状态
out = self.fc(out)
return out
# 使用示例
model = GRUModel(input_size=100, hidden_size=64, output_size=10) # 假设我们有100维输入,64隐层,10个输出节点
# 初始化隐状态
h0 = torch.zeros(2, 100, 64) # 如果是双向GRU,层数乘以2
# 假设x是形状(batch_size, seq_len, 100)的数据
input_data = torch.randn(100, 50, 100)
output = model(input_data, h0)
```
这只是一个基础示例,实际应用中可能需要处理更多细节,如添加dropout、包装在训练循环里等。如果你对代码的具体部分有疑问,可以问我相关的
阅读全文
相关推荐
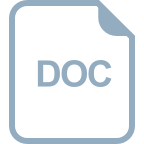
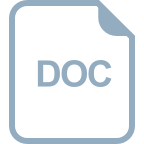
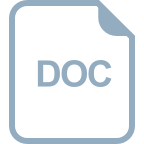












