给我一个基于pytorch的ddpg代码
时间: 2024-01-21 08:04:12 浏览: 17
当然可以!下面是一个基于PyTorch的DDPG(Deep Deterministic Policy Gradient)算法的代码示例:
```python
import torch
import torch.nn as nn
import torch.optim as optim
import numpy as np
# 定义Actor网络
class Actor(nn.Module):
def __init__(self, state_dim, action_dim, hidden_dim):
super(Actor, self).__init__()
self.fc1 = nn.Linear(state_dim, hidden_dim)
self.fc2 = nn.Linear(hidden_dim, hidden_dim)
self.fc3 = nn.Linear(hidden_dim, action_dim)
def forward(self, state):
x = torch.relu(self.fc1(state))
x = torch.relu(self.fc2(x))
action = torch.tanh(self.fc3(x))
return action
# 定义Critic网络
class Critic(nn.Module):
def __init__(self, state_dim, action_dim, hidden_dim):
super(Critic, self).__init__()
self.fc1 = nn.Linear(state_dim + action_dim, hidden_dim)
self.fc2 = nn.Linear(hidden_dim, hidden_dim)
self.fc3 = nn.Linear(hidden_dim, 1)
def forward(self, state, action):
x = torch.relu(self.fc1(torch.cat([state, action], 1)))
x = torch.relu(self.fc2(x))
q_value = self.fc3(x)
return q_value
# 定义DDPG算法类
class DDPG:
def __init__(self, state_dim, action_dim, hidden_dim, lr_actor, lr_critic, gamma):
self.actor = Actor(state_dim, action_dim, hidden_dim)
self.actor_target = Actor(state_dim, action_dim, hidden_dim)
self.critic = Critic(state_dim, action_dim, hidden_dim)
self.critic_target = Critic(state_dim, action_dim, hidden_dim)
self.actor_optim = optim.Adam(self.actor.parameters(), lr=lr_actor)
self.critic_optim = optim.Adam(self.critic.parameters(), lr=lr_critic)
self.gamma = gamma
self.loss = nn.MSELoss()
def select_action(self, state):
state = torch.FloatTensor(state).unsqueeze(0)
action = self.actor(state)
return action.detach().numpy()[0]
def update(self, state, action, reward, next_state, done):
state = torch.FloatTensor(state)
action = torch.FloatTensor(action)
reward = torch.FloatTensor([reward])
next_state = torch.FloatTensor(next_state)
mask = torch.FloatTensor([1 - done])
# 更新Critic网络
q_value = self.critic(state, action)
next_action = self.actor_target(next_state)
target_q_value = reward + self.gamma * self.critic_target(next_state, next_action) * mask
critic_loss = self.loss(q_value, target_q_value.detach())
self.critic_optim.zero_grad()
critic_loss.backward()
self.critic_optim.step()
# 更新Actor网络
actor_loss = -self.critic(state, self.actor(state)).mean()
self.actor_optim.zero_grad()
actor_loss.backward()
self.actor_optim.step()
# 更新目标网络
for target_param, param in zip(self.actor_target.parameters(), self.actor.parameters()):
target_param.data.copy_(param.data)
for target_param, param in zip(self.critic_target.parameters(), self.critic.parameters()):
target_param.data.copy_(param.data)
# 使用示例
state_dim = 4
action_dim = 2
hidden_dim = 32
lr_actor = 0.001
lr_critic = 0.001
gamma = 0.99
ddpg = DDPG(state_dim, action_dim, hidden_dim, lr_actor, lr_critic, gamma)
# 在环境中进行训练
for episode in range(num_episodes):
state = env.reset()
done = False
while not done:
action = ddpg.select_action(state)
next_state, reward, done, _ = env.step(action)
ddpg.update(state, action, reward, next_state, done)
state = next_state
```
这是一个简单的DDPG算法实现,你可以根据自己的需求进行修改和扩展。请确保已经安装了PyTorch和其他所需的依赖库。
相关推荐
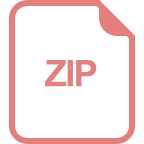
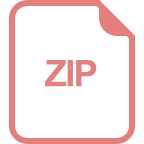
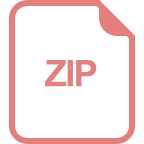














