Light pollution alters our view of the night sky, has environmental impacts and affects our health and safety. For example, plant maturation may be delayed or accelerated, and migration patterns of wildlife affected. Excessive artificial light may confuse our circadian rhythms, leading to poor sleep quality and perhaps physical and mental health issues. Glare caused by artificial lights may contribute to some motor vehicle accidents. Develop a broadly applicable metric, and give its mathematical expression to identify the light pollution risk level of a location. Apply your metric and interpret its results on the following four diverse types of locations: a protected land location, a rural community, a suburban community, and an urban community. Describe three possible intervention strategies to address light pollution.
时间: 2023-03-08 07:19:20 浏览: 109
我们可以使用光度定义一种广泛适用的度量标准,其数学表达式为:L = 4πI / S,其中L表示光度,I表示光照强度,S表示光源的发射面积。使用这个标准可以衡量出一个地点的光污染风险等级。对于一个受保护的土地,如果L值较低,这意味着地点的光污染风险等级较低;对于一个乡村,如果L值较高,这意味着地点的光污染风险等级较高;对于一个郊区,如果L值处于中等水平,这意味着地点的光污染风险等级中等;对于一个城市,如果L值较高,这意味着地点的光污染风险等级比较高。为了解决光污染的问题,我们可以采取三种干预策略:(1)加强公众意识,增强人们对光污染的认识;(2)加强管理,减少不必要的照明活动;(3)制定有效的照明标准,为不同地点安装合适的照明设备。
相关问题
3)A digital clock consists of a screen to display the time and a dial for setting in turn the year, month, day, hour and minute. Twisting the dial to the left reduces by one the value being changed but twisting it to the right increases it by one. Pushing the dial alters which value is being adjusted. At first, it is the year but after the dial is pushed once, it is the month, then after the dial is pushed again, it is the day and so on. Imagine the clock is represented by a class with attributes year, month, day etc. The following is what the code for a method rotateDialLeft() might look like. public void rotateDialLeft() { if (mode == YEAR_MODE) { year--; } else if (mode == MONTH_MODE) { month--; } else if (mode == DAY_MODE) { day--; } else if (mode == HOUR_MODE) { hour--; } else if (mode == MINUTE_MODE) { minute--; } } The code for rotateDialRight() is similar. Apply the Open-Closed Principle to explain why the above code is unsatisfactory from the design viewpoint, considering the possibility of future change to the code, giving an example of such a change. 5)Give the code required for the classes introduced in question 3), focusing on the code for a method selectState() which changes the value that is being adjusted from years to months. Make it clear in which classes the code is to be found. Assume the existence of other methods that are needed such as getMonthSetUpState(). 8)Suppose that in a multiplayer role-playing game, a class Client has a dependency to an interface Fighter with public methods attack(), defend() and escape(). The game designer now wishes for Client to use a class Wizard with three different but equivalent public methods castDestructionSpell(), shield() and portal(). Explain how it is possible to do this using an appropriate design pattern.
3) The current implementation of the rotateDialLeft() method violates the Open-Closed Principle, which states that software entities (classes, modules, functions, etc.) should be open for extension but closed for modification. This means that we should be able to add new functionality without changing the existing code. In the current code, if we want to add a new mode (e.g. seconds), we would need to modify the existing if-else statements and add a new condition. This violates the Open-Closed Principle.
An example of a future change that could require modification of the existing code is if we wanted to add a new mode (e.g. century), we would need to modify the existing code and add a new condition. To fix this, we can use the Strategy pattern to encapsulate the different modes and their behavior and make the code more extensible.
5) Here is the code for the classes introduced in question 3:
```
public interface DialMode {
void rotateLeft();
void rotateRight();
void selectState();
}
public class YearMode implements DialMode {
private int year;
public YearMode(int year) {
this.year = year;
}
public void rotateLeft() {
year--;
}
public void rotateRight() {
year++;
}
public void selectState() {
// do nothing, already in year mode
}
}
public class MonthMode implements DialMode {
private int month;
public MonthMode(int month) {
this.month = month;
}
public void rotateLeft() {
month--;
}
public void rotateRight() {
month++;
}
public void selectState() {
// switch to day mode
int daysInMonth = getDaysInMonth();
DayMode dayMode = new DayMode(daysInMonth);
mode = dayMode;
}
private int getDaysInMonth() {
// implementation omitted
}
}
public class DayMode implements DialMode {
private int day;
public DayMode(int day) {
this.day = day;
}
public void rotateLeft() {
day--;
}
public void rotateRight() {
day++;
}
public void selectState() {
// switch to hour mode
HourMode hourMode = new HourMode(0);
mode = hourMode;
}
}
public class HourMode implements DialMode {
private int hour;
public HourMode(int hour) {
this.hour = hour;
}
public void rotateLeft() {
hour--;
}
public void rotateRight() {
hour++;
}
public void selectState() {
// switch to minute mode
MinuteMode minuteMode = new MinuteMode(0);
mode = minuteMode;
}
}
public class MinuteMode implements DialMode {
private int minute;
public MinuteMode(int minute) {
this.minute = minute;
}
public void rotateLeft() {
minute--;
}
public void rotateRight() {
minute++;
}
public void selectState() {
// switch to year mode
YearMode yearMode = new YearMode(2000);
mode = yearMode;
}
}
public class Clock {
private DialMode mode;
public Clock() {
YearMode yearMode = new YearMode(2000);
mode = yearMode;
}
public void rotateDialLeft() {
mode.rotateLeft();
}
public void rotateDialRight() {
mode.rotateRight();
}
public void selectState() {
mode.selectState();
}
}
```
The selectState() method is found in each DialMode implementation. In the MonthMode implementation, it switches to the DayMode, in the DayMode implementation, it switches to the HourMode, in the HourMode implementation, it switches to the MinuteMode, and in the MinuteMode implementation, it switches back to the YearMode.
8) We can use the Adapter pattern to adapt the interface of the Wizard class to the Fighter interface. First, we create an adapter class that implements the Fighter interface and has a reference to a Wizard object:
```
public class WizardAdapter implements Fighter {
private Wizard wizard;
public WizardAdapter(Wizard wizard) {
this.wizard = wizard;
}
public void attack() {
wizard.castDestructionSpell();
}
public void defend() {
wizard.shield();
}
public void escape() {
wizard.portal();
}
}
```
Now, we can use an instance of the WizardAdapter class wherever we need a Fighter object:
```
Client client = new Client();
Wizard wizard = new Wizard();
Fighter wizardAdapter = new WizardAdapter(wizard);
client.setFighter(wizardAdapter);
```
In this example, the Client class has a dependency on the Fighter interface. We create a Wizard object and wrap it in a WizardAdapter object that implements the Fighter interface. We then set the wizardAdapter as the fighter for the client. When the client calls the attack(), defend(), or escape() method, it will actually call the corresponding method on the Wizard object through the adapter. This allows us to use the Wizard class as if it implements the Fighter interface, without changing the interface of the Fighter or the implementation of the Wizard.
Sklearn.metrics.roc_auc_score模块中的源代码
以下是sklearn.metrics.roc_auc_score模块的源代码:
```python
def roc_auc_score(y_true, y_score, average='macro', sample_weight=None,
max_fpr=None, multi_class='raise', labels=None):
"""Compute Area Under the Receiver Operating Characteristic Curve (ROC AUC)
from prediction scores.
Note: this implementation can be used with binary, multiclass and
multilabel classification, but some restrictions apply (see Parameters).
Read more in the :ref:`User Guide <roc_metrics>`.
Parameters
----------
y_true : array-like of shape (n_samples,) or (n_samples, n_classes)
True labels or binary label indicators. The binary and multiclass cases
expect labels with shape (n_samples,) while the multilabel case expects
binary label indicators with shape (n_samples, n_classes).
y_score : array-like of shape (n_samples,) or (n_samples, n_classes)
Target scores. In the binary and multilabel cases, these can be either
probability estimates or non-thresholded decision values (as returned
by `decision_function` on some classifiers). In the multiclass case,
these must be probability estimates which sum to 1. The binary case
expects a shape (n_samples,), and the scores must be the scores of
the class with the greater label. The multiclass and multilabel
cases expect a shape (n_samples, n_classes).
average : {'micro', 'macro', 'samples', 'weighted'} or None, \
default='macro'
If ``None``, the scores for each class are returned. Otherwise,
this determines the type of averaging performed on the data:
``'micro'``:
Calculate metrics globally by counting the total true positives,
false negatives and false positives.
``'macro'``:
Calculate metrics for each label, and find their unweighted
mean. This does not take label imbalance into account.
``'weighted'``:
Calculate metrics for each label, and find their average, weighted
by support (the number of true instances for each label). This
alters 'macro' to account for label imbalance; it can result in an
F-score that is not between precision and recall.
``'samples'``:
Calculate metrics for each instance, and find their average
(only meaningful for multilabel classification).
sample_weight : array-like of shape (n_samples,), default=None
Sample weights.
max_fpr : float or None, default=None
If not ``None``, the standardized partial AUC [2]_ over the range
[0, max_fpr] is returned. For the multiclass case, ``max_fpr``
should be either ``None`` or ``1.0`` as partial AUC makes
sense for binary classification only.
multi_class : {'raise', 'ovr', 'ovo'}, default='raise'
Multiclass only. Determines the type of configuration to use.
The default value raises an error, so either ``'ovr'`` or
``'ovo'`` must be passed explicitly.
``'ovr'``:
Computes ROC curve independently for each class. For each class,
the binary problem y_true == i or not is solved and the
corresponding ROC curve is computed and averaged across
classes. This is a commonly used strategy for multiclass
or multi-label classification problems.
``'ovo'``:
Computes pairwise ROC curve for each pair of classes. For each
pair of classes, the binary problem y_true == i or y_true == j
is solved and the corresponding ROC curve is computed. The
micro-averaged ROC curve is computed from the individual curves
and hence is agnostic to the class balance.
labels : array-like of shape (n_classes,), default=None
Multiclass only. List of labels to index ``y_score`` used for
multiclass. If ``None``, the lexical order of ``y_true`` is used to
index ``y_score``.
Returns
-------
auc : float or dict (if ``multi_class`` is ``'ovo'`` or ``'ovr'``)
AUC of the ROC curves.
If ``multi_class`` is ``'ovr'``, then returns an array of shape
``(n_classes,)`` such that each element corresponds to the AUC of
the ROC curve for a specific class.
If ``multi_class`` is ``'ovo'``, then returns a dict where the keys
are ``(i, j)`` tuples and the values are the AUCs of the ROC curve
for the binary problem of predicting class ``i`` vs. class ``j``.
See also
--------
roc_curve : Compute Receiver operating characteristic (ROC) curve.
roc_auc : Compute Area Under the Receiver Operating Characteristic Curve
(ROC AUC) from prediction scores
Examples
--------
>>> import numpy as np
>>> from sklearn.metrics import roc_auc_score
>>> y_true = np.array([0, 0, 1, 1])
>>> y_scores = np.array([0.1, 0.4, 0.35, 0.8])
>>> roc_auc_score(y_true, y_scores)
0.75
>>> y_true = np.array([0, 0, 1, 1])
>>> y_scores = np.array([[0.1, 0.9], [0.4, 0.6], [0.35, 0.65], [0.8, 0.2]])
>>> roc_auc_score(y_true, y_scores, multi_class='ovo')
0.6666666666666667
>>> roc_auc_score(y_true, y_scores[:, 1])
0.75
"""
# validation of the input y_score
if not (y_true.shape == y_score.shape):
raise ValueError("y_true and y_score have different shape.")
if (not is_multilabel(y_true) and not is_multiclass(y_true)):
# roc_auc_score only supports binary and multiclass classification
# for the time being
if len(np.unique(y_true)) == 2:
# Only one class present in y_true. ROC AUC score is not defined
# in that case. Note that raising an error is consistent with the
# deprecated roc_auc_score behavior.
raise ValueError(
"ROC AUC score is not defined in that case: "
"y_true contains only one label ({0}).".format(
format_label(y_true[0])
)
)
else:
raise ValueError(
"ROC AUC score is not defined in that case: "
"y_true has {0} unique labels. ".format(len(np.unique(y_true))) +
"ROC AUC score is defined only for binary or multiclass "
"classification where the number of classes is greater than "
"one."
)
if multi_class == 'raise':
raise ValueError("multi_class must be in ('ovo', 'ovr')")
elif multi_class == 'ovo':
if is_multilabel(y_true):
# check if max_fpr is valid in this case
if max_fpr is not None and (max_fpr == 0 or max_fpr > 1):
raise ValueError("Expected max_fpr in range (0, 1], got: %f" %
max_fpr)
return _multiclass_roc_auc_score_ovr(y_true, y_score,
average, sample_weight,
max_fpr=max_fpr)
else:
return _binary_roc_auc_score(y_true, y_score, average,
sample_weight, max_fpr=max_fpr)
elif multi_class == 'ovr':
if is_multilabel(y_true):
return _multilabel_roc_auc_score_ovr(y_true, y_score,
average, sample_weight)
else:
return _multiclass_roc_auc_score_ovr(y_true, y_score,
average, sample_weight,
labels=labels)
else:
raise ValueError("Invalid multi_class parameter: {0}".format(multi_class))
```
这段代码实现了计算ROC AUC的功能,支持二元、多类和多标签分类。其中,分为'ovo'和'ovr'两种多类模式,'ovo'表示一对一,'ovr'表示一对多。
阅读全文
相关推荐




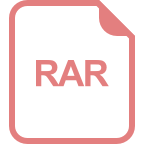


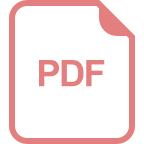








