我想在jupyter中看运行的epoch
时间: 2024-11-23 08:39:35 浏览: 21
在Jupyter Notebook中查看训练过程中每个epoch的进度通常是通过TensorFlow、PyTorch等深度学习库中的可视化工具或自定义代码实现的。例如,在TensorFlow中,你可以使用`tf.keras.callbacks.TensorBoard`回调函数来实时更新训练过程中的图表,包括损失值、准确率等指标随epoch的变化。
如果你使用的是Keras API,可以在模型.fit()方法中添加一个`TensorBoard`实例,并指定保存路径:
```python
tb_callback = TensorBoard(log_dir="logs/fit", histogram_freq=1)
model.fit(x_train, y_train, epochs=num_epochs, validation_data=(x_val, y_val), callbacks=[tb_callback])
```
在这个例子中,打开浏览器访问`http://localhost:6006`可以查看TensorBoard页面,其中会有epoch对应的训练曲线和验证结果。
如果你正在手动跟踪数据,可能需要在每次epoch结束时更新一个DataFrame或者其他数据结构,然后使用matplotlib或其他可视化库绘制图表。
相关问题
帮我写一份可以在jupyter上运行的用NASnet模型训练cifar10数据集的代码
好的,以下是可以在Jupyter上运行的使用NASnet模型训练CIFAR10数据集的代码示例。注意,由于NASnet模型较大,需要较长的时间进行训练,建议在GPU环境下运行。
首先,我们需要导入所需的库和模块:
``` python
import tensorflow as tf
from tensorflow.keras.datasets import cifar10
from tensorflow.keras.preprocessing.image import ImageDataGenerator
from tensorflow.keras.utils import to_categorical
from tensorflow.keras.applications.nasnet import NASNetMobile
from tensorflow.keras.layers import Dense, GlobalAveragePooling2D
from tensorflow.keras.models import Model
```
接下来,我们需要加载CIFAR10数据集:
``` python
(x_train, y_train), (x_test, y_test) = cifar10.load_data()
```
然后,我们需要对数据进行预处理:
``` python
# 将像素值归一化到0-1之间
x_train = x_train.astype('float32') / 255
x_test = x_test.astype('float32') / 255
# 将标签进行one-hot编码
y_train = to_categorical(y_train, num_classes=10)
y_test = to_categorical(y_test, num_classes=10)
```
接下来,我们需要定义NASnet模型:
``` python
# 加载NASnet模型
base_model = NASNetMobile(weights='imagenet', include_top=False, input_shape=(32, 32, 3))
# 添加全局平均池化层和全连接层
x = base_model.output
x = GlobalAveragePooling2D()(x)
x = Dense(1024, activation='relu')(x)
predictions = Dense(10, activation='softmax')(x)
# 构建完整模型
model = Model(inputs=base_model.input, outputs=predictions)
```
接下来,我们需要编译模型并开始训练:
``` python
# 编译模型
model.compile(optimizer='adam', loss='categorical_crossentropy', metrics=['accuracy'])
# 数据增强
datagen = ImageDataGenerator(
rotation_range=20,
width_shift_range=0.2,
height_shift_range=0.2,
horizontal_flip=True)
# 开始训练
model.fit_generator(datagen.flow(x_train, y_train, batch_size=32),
steps_per_epoch=len(x_train) / 32, epochs=10,
validation_data=(x_test, y_test))
```
最后,我们可以使用测试集对模型进行评估:
``` python
# 对测试集进行评估
score = model.evaluate(x_test, y_test, verbose=0)
print("Test Loss: {:.3f}, Test Accuracy: {:.3f}".format(score[0], score[1]))
```
希望这份代码能够帮助到你!
在jupyter notebook训练过程中断了,如何继续上次的epoch继续运行?
在Jupyter Notebook中中断模型训练后,想要恢复并继续上一个epoch,通常需要保存一些关键信息以便后续加载。以下是一般的步骤:
1. **保存模型状态**:在训练过程中,确保在每个epoch结束时或者每达到一定迭代次数后,保存模型的状态(通常是权重)。可以使用`model.save_weights('path/to/weights.h5')`(如果你使用的是Keras)或者`torch.save(model.state_dict(), 'path/to/model.pth')`(如果你使用PyTorch)。
2. **恢复训练**:在新的会话中打开同一个Notebook,首先加载之前保存的模型权重。例如,对于Keras:
```python
model = YourModel() # 重建模型结构
model.load_weights('path/to/weights.h5')
```
或者 PyTorch:
```python
model = YourModel() # 重建模型实例
model.load_state_dict(torch.load('path/to/model.pth'))
```
3. **设置起始epoch数**:如果训练过程中有跟踪当前epoch的变量,比如`epoch_number`,记得在开始新训练时更新它,使其从上次中断的地方开始。
4. **继续训练循环**:现在模型已经准备好了,你可以继续训练过程,从上次的epoch数开始迭代数据。
```python
for epoch in range(resumed_epoch, total_epochs): # resumed_epoch是你上次停止的地方
for batch_data in train_loader:
# 开始训练...
```
阅读全文
相关推荐
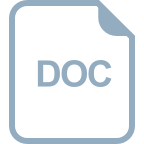
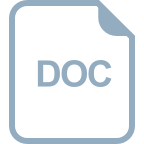
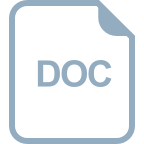
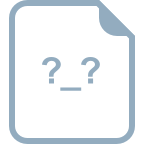
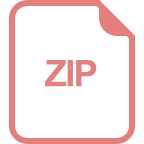
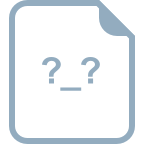










