决策树ID3算法后剪枝
时间: 2023-05-20 21:03:28 浏览: 40
决策树ID3算法后剪枝是一种用于决策树的优化方法,它可以通过剪枝来减少决策树的复杂度,提高模型的泛化能力。具体实现方法是在决策树构建完成后,对树进行剪枝,将一些不必要的分支或叶子节点删除,从而达到简化模型的目的。
相关问题
能给出决策树ID3算法的后剪枝部分代码吗
当然可以,以下是决策树ID3算法的后剪枝部分代码:
```python
def prune(tree, validation_set):
if not tree.children:
return tree
# Recursively prune children
for child in tree.children:
prune(child, validation_set)
# Check if pruning this node improves accuracy on validation set
before_pruning = accuracy(tree, validation_set)
tree.is_leaf = True
after_pruning = accuracy(tree, validation_set)
if before_pruning >= after_pruning:
tree.is_leaf = False
return tree
```
这段代码会递归地遍历决策树的所有节点,然后对每个非叶子节点进行剪枝。具体来说,它会将该节点标记为叶子节点,然后计算在验证集上的准确率。如果剪枝后的准确率比剪枝前高,那么就保留该节点,否则就将该节点还原为非叶子节点。最终返回剪枝后的决策树。
决策树id3算法国内研究现状
决策树是一种常用的机器学习算法,ID3算法是其中的一种经典算法。国内在决策树ID3算法研究方面也有相应的现状。
首先,国内研究者对决策树ID3算法进行了深入研究,并在算法原理和优化方面进行了探索。例如,研究者提出了改进的ID3算法,如C4.5算法、CART算法等,通过引入剪枝策略和连续值处理等优化方法,提高了决策树的性能和泛化能力。
其次,国内学者还通过对决策树ID3算法的应用实践进行研究,尤其在领域特定的决策树构建方面取得了不错的成果。例如,在医疗领域,研究者利用决策树ID3算法对疾病诊断和治疗决策进行了研究,提高了医疗决策的准确性和效率。此外,在金融领域、教育领域、社交网络等领域也都有一定的应用研究。
同时,国内研究者还对决策树ID3算法进行了改进和拓展。例如,在处理大数据和高维数据方面,研究者提出了基于决策树的并行化算法和增量学习算法,以提高算法的可扩展性和效率。
总体而言,决策树ID3算法在国内的研究得到了广泛的关注和应用,并在算法原理、优化方法、应用实践等方面有所突破。未来,可进一步深化决策树算法的研究,探索更多的优化策略和应用场景,以提升决策树算法的性能和适用性。
相关推荐
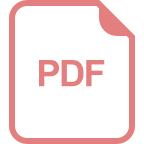
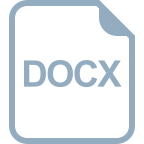
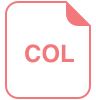
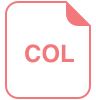
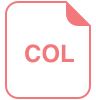

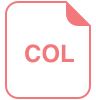







