错误信息如下Dot indexing is not supported for variables of this type. Error in untitled15 (line 13) for j=1:length(line_data.stations)
时间: 2024-02-20 12:01:46 浏览: 233
根据错误信息来看,可能的原因是line_data不是一个结构体或类,无法使用点运算符(.)进行索引,因此需要使用括号运算符([])进行索引。可以将代码修改为以下形式:
```
for j=1:length(line_data.stations)
station_name=line_data.stations{j}.name;
station_ur1=sprintf(subway_station_ur1,ak,city,station_name,city);
station_data=webread(station_ur1);
end
```
这里的关键是将line_data.stations{j}改为line_data.stations(j),使用括号运算符进行索引。
相关问题
Dot indexing is not supported for variables of this type. Error in untitled15 (line 13) for j=1:length(line_data.stations)怎么修改
这个错误通常表示您正在尝试对不支持点索引的变量进行点索引操作。这可能是因为该变量不是结构体或类对象。
在这个具体的问题中,可能是因为`line_data`不是一个结构体或类对象,因此无法使用点索引来访问其属性。为了解决这个问题,您可以考虑使用其他适当的索引方法,例如括号索引。
例如,您可以将`for`循环修改为以下形式:
```
for j = 1:numel(line_data.stations)
station = line_data.stations{j};
% 对station执行您需要的操作
end
```
在这个示例中,我们使用了`numel()`函数来获取`line_data.stations`中单元格的数量,然后使用`for`循环遍历这些单元格。在每个循环中,我们使用大括号索引来提取当前单元格中的数据,并将其存储在一个名为`station`的变量中。然后,您可以对`station`变量执行您需要的操作。
请注意,这只是一个示例,具体的修改取决于您的代码实现和数据类型。
Brace indexing is not supported for variables of this type. Error in hw (line 45) mean_image = mean(abs(sumOfSquares{coilIndex}), 2);
这个错误消息表明你试图对某个变量使用花括号(`{}`)进行索引,但是这种语法对于当前变量的数据类型并不支持。在MATLAB中,`brace indexing`(花括号索引)通常用于结构体(struct)或者某些特定类型的细胞数组(cell array),特别是那些包含不同类型数据的复杂结构。
在这个例子中,`sumOfSquares{coilIndex}` 表明`sumOfSquares` 可能是一个cell数组,其中每个元素都是一个二维数组。`mean` 函数期望一个一维向量作为输入,所以当你尝试用 `2` 作为第二个维度索引来取出一列(相当于column-wise求平均)时,产生了错误。
解决此问题的一种可能方法是首先将每一列提取出来,然后对每一列求平均。这可以通过 `squeeze` 函数去除多余的维度后,使用 `:` 通配符来选择所有的列,例如:
```matlab
columnVectors = squeeze(sumOfSquares{coilIndex});
meanImage = mean(columnVectors, 2);
```
如果你的 `sumOfSquares` 是一个普通的矩阵而非cell,那么直接使用`mean`函数应该就没问题了:
```matlab
meanImage = mean(sumOfSquares, 2);
```
如果仍然有误,确认一下`sumOfSquares`的数据类型以及它是否真的包含了二维数据,并确保`coilIndex`指向的是正确的子数组。
阅读全文
相关推荐
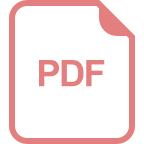
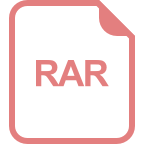










