蚁群算法通过python实现
时间: 2023-08-29 14:13:37 浏览: 43
蚁群算法是一种模拟蚂蚁寻找食物的行为来解决优化问题的算法。下面介绍如何使用 Python 实现蚁群算法。
步骤1:定义问题
首先,需要定义一个优化问题,例如:求解函数 $f(x)=x^2+2x+1$ 的最小值,其中 $x$ 的范围为 $[-5,5]$。
步骤2:初始化参数
在蚁群算法中,需要定义许多参数,包括蚂蚁数量、迭代次数、信息素挥发系数、信息素增加系数等。这里定义蚂蚁数量为 20,迭代次数为 100,信息素挥发系数为 0.5,信息素增加系数为 1。
```python
ant_num = 20 # 蚂蚁数量
iter_num = 100 # 迭代次数
evap_rate = 0.5 # 信息素挥发系数
alpha = 1 # 信息素增加系数
```
步骤3:初始化蚂蚁位置和信息素
接下来,需要随机初始化蚂蚁的位置和信息素的初始值。蚂蚁的位置可以在 $[-5,5]$ 的范围内随机生成,信息素的初始值设置为一个很小的数,例如 0.01。
```python
import numpy as np
ant_pos = np.random.uniform(-5, 5, size=(ant_num, 1)) # 蚂蚁位置
pheromone = np.ones((100,)) * 0.01 # 信息素
```
步骤4:定义蚂蚁的移动规则
根据蚂蚁寻找食物的行为,蚂蚁在搜索过程中会遵循一定的规则。这里定义蚂蚁的移动规则为:蚂蚁按照一定的概率选择下一步的移动方向,概率与当前位置的信息素浓度有关;蚂蚁移动一步后,会在当前位置留下一定的信息素。
```python
def ant_move(ant_pos, pheromone):
# 计算每只蚂蚁下一步移动的方向
prob = pheromone / np.sum(pheromone)
move_dir = np.random.choice([-1, 1], size=(ant_num,), p=[prob, 1 - prob])
# 更新蚂蚁的位置和留下的信息素
ant_pos += move_dir.reshape(-1, 1)
for i in range(ant_num):
idx = int(ant_pos[i])
pheromone[idx] += alpha
return ant_pos, pheromone
```
步骤5:定义蚂蚁的选择规则
当所有蚂蚁都完成一次移动后,需要根据蚂蚁的选择规则更新信息素。在蚁群算法中,蚂蚁会选择路径上信息素浓度高的方向,因此需要根据蚂蚁的位置更新信息素。
```python
def update_pheromone(ant_pos, pheromone, evap_rate):
# 计算每只蚂蚁经过的路径上留下的信息素
ant_path = np.zeros((100,))
for i in range(ant_num):
idx = int(ant_pos[i])
ant_path[idx] += 1
# 更新信息素
pheromone *= (1 - evap_rate) # 信息素挥发
pheromone += ant_path # 信息素增加
return pheromone
```
步骤6:迭代求解
最后,将上述步骤组合起来,进行迭代求解。每次迭代后,记录当前的最优解和最优解对应的位置。迭代结束后,返回最优解和最优解对应的位置。
```python
def ant_colony_optimization():
ant_pos = np.random.uniform(-5, 5, size=(ant_num, 1))
pheromone = np.ones((100,)) * 0.01
best_fitness = np.inf
best_position = None
for i in range(iter_num):
ant_pos, pheromone = ant_move(ant_pos, pheromone)
fitness = ant_pos ** 2 + 2 * ant_pos + 1
if np.min(fitness) < best_fitness:
best_fitness = np.min(fitness)
best_position = ant_pos[np.argmin(fitness)]
pheromone = update_pheromone(ant_pos, pheromone, evap_rate)
return best_fitness, best_position
```
完整代码如下:
相关推荐
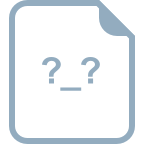














