从一个DataFrame对象中选择一个子集,并根据某一列进行合并
时间: 2024-01-23 18:02:27 浏览: 23
可以使用 Pandas 库中的 `groupby` 方法和 `agg` 方法来实现。
假设我们有一个 DataFrame 对象 `df`,其中有多个列,我们需要根据其中一列 `col_name` 进行合并。可以通过以下代码实现:
``` python
subset = df.loc[:, ['col_name', 'other_col']]
result = subset.groupby('col_name').agg({'other_col': 'sum'}).reset_index()
```
第一行代码中,使用 `loc` 方法选择了所有行和 `col_name`、`other_col` 两列,生成了一个名为 `subset` 的 DataFrame 子集。第二行代码中,使用 `groupby` 方法对 `subset` 进行分组操作,按 `col_name` 列的值进行分组,然后使用 `agg` 方法对分组后的 `other_col` 列进行求和操作,生成了一个名为 `result` 的新 DataFrame。最后,使用 `reset_index` 方法重置了索引,使 `col_name` 列变成了一个新的列。
这样,我们就得到了按 `col_name` 列合并后的结果,其中每个不同的 `col_name` 对应一个唯一的值。如果 `other_col` 列不是数值类型,可以使用其他方法进行合并,例如使用 `join` 方法进行字符串拼接。
相关问题
从一个DataFrame对象中选择一个子集,并根据某一列进行去重。
可以使用 Pandas 库中的 `loc` 方法和 `drop_duplicates` 方法来实现。
假设我们有一个 DataFrame 对象 `df`,其中有多个列,我们需要根据其中一列 `col_name` 进行去重。可以通过以下代码实现:
``` python
subset = df.loc[:, ['col_name']]
subset = subset.drop_duplicates()
```
第一行代码中,使用 `loc` 方法选择了所有行和 `col_name` 列,生成了一个名为 `subset` 的 DataFrame 子集。第二行代码中,使用 `drop_duplicates` 方法对 `subset` 进行去重操作,生成了一个不含重复值的新 DataFrame。
注意:`drop_duplicates` 方法会默认保留第一个出现的重复值,删除后续出现的重复值。如果需要保留最后一个出现的重复值,可以传入参数 `keep='last'`。
从一个DataFrame对象中选择一个子集,并根据某一列进行连接。
可以使用 Pandas 库中的 `merge` 方法来实现。
假设我们有两个 DataFrame 对象 `df1` 和 `df2`,它们都有多个列,需要根据其中一列 `col_name` 进行连接。可以通过以下代码实现:
``` python
subset1 = df1.loc[:, ['col_name', 'other_col1']]
subset2 = df2.loc[:, ['col_name', 'other_col2']]
result = pd.merge(subset1, subset2, on='col_name')
```
第一行代码中,使用 `loc` 方法选择了 `df1` 中的所有行和 `col_name`、`other_col1` 两列,生成了一个名为 `subset1` 的 DataFrame 子集。第二行代码中,使用 `loc` 方法选择了 `df2` 中的所有行和 `col_name`、`other_col2` 两列,生成了一个名为 `subset2` 的 DataFrame 子集。第三行代码中,使用 `merge` 方法对 `subset1` 和 `subset2` 进行连接操作,按 `col_name` 列的值进行连接,生成了一个名为 `result` 的新 DataFrame。
这样,我们就得到了按 `col_name` 列连接后的结果,其中每个不同的 `col_name` 对应一个包含两个其他列的新行。如果需要按照多个列进行连接,可以在 `on` 参数中传入一个列表。如果需要使用不同的连接方式,例如左连接、右连接、外连接等,可以在 `merge` 方法中传入 `how` 参数。
相关推荐
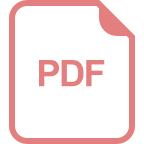












