帮我修改一下这段代码的错误import networkx as nx G = nx.DiGraph() start = [1, 3, 5, 7] to = [2, 4, 6, 8] for j in range(len(start)): G.add_edge(start[j], to[j]) nx.draw(G, with_lables=True)
时间: 2023-06-16 21:02:30 浏览: 55
import networkx as nx
G = nx.DiGraph()
start = [1, 3, 5, 7]
to = [2, 4, 6, 8]
for j in range(len(start)):
G.add_edge(start[j], to[j])
nx.draw(G, with_labels=True)
# 你在原来的代码中写成了 with_lables,正确的参数名称应该是 with_labels。此外,你需要在绘图之前先添加边。
相关问题
import pandas as pd import numpy as np import networkx as nx import matplotlib.pyplot as plt df = pd.read_excel(r"C:\Users\li'yi'jie\Desktop\运筹学网络规划数据.xlsx") edges = [] for i in range(len(df)): edge = { "id": df.loc[i, "边的编号"], "tail": df.loc[i, "边的尾节点"], "head": df.loc[i, "边的头节点"], "length": df.loc[i, "长度"], "capacity": df.loc[i, "容量"] } edges.append(edge) plt.figure(figsize=(15,15)) G = nx.DiGraph() for edge in edges: G.add_edge(edge["tail"], edge["head"], weight=edge["length"]) pos = nx.spring_layout(G) nx.draw(G, pos, with_labels=True) labels = nx.get_edge_attributes(G, "weight") nx.draw_networkx_edge_labels(G, pos, edge_labels=labels) #nx.draw_networkx_edge_labels(G, pos, edge_labels=labels, label_pos=0.3) plt.show() all_pairs = dict(nx.all_pairs_dijkstra_path_length(G)) rows = [] for start_node, dist_dict in all_pairs.items(): for end_node, dist in dist_dict.items(): rows.append({'起始节点': start_node, '终止节点': end_node, '最短路径长度': dist}) df_result = pd.DataFrame(rows) df_result.to_excel('all_pairs.xlsx', index=False)请问在写入excel的时候,能否把经过的边的编号一起写入呢?麻烦给下修改后的代码,谢谢你!
可以在遍历最短路径的时候记录下经过的边的编号,然后将其存储到结果表格中。以下是修改后的代码:
```
import pandas as pd
import numpy as np
import networkx as nx
import matplotlib.pyplot as plt
df = pd.read_excel(r"C:\Users\li'yi'jie\Desktop\运筹学网络规划数据.xlsx")
edges = []
for i in range(len(df)):
edge = {
"id": df.loc[i, "边的编号"],
"tail": df.loc[i, "边的尾节点"],
"head": df.loc[i, "边的头节点"],
"length": df.loc[i, "长度"],
"capacity": df.loc[i, "容量"]
}
edges.append(edge)
plt.figure(figsize=(15,15))
G = nx.DiGraph()
for edge in edges:
G.add_edge(edge["tail"], edge["head"], weight=edge["length"])
pos = nx.spring_layout(G)
nx.draw(G, pos, with_labels=True)
labels = nx.get_edge_attributes(G, "weight")
nx.draw_networkx_edge_labels(G, pos, edge_labels=labels)
#plt.draw()
plt.show()
all_pairs = dict(nx.all_pairs_dijkstra_path(G))
rows = []
for start_node, paths in all_pairs.items():
for end_node, path in paths.items():
path_edges = []
for i in range(len(path)-1):
edge_id = G[path[i]][path[i+1]]['id']
path_edges.append(edge_id)
row = {
'起始节点': start_node,
'终止节点': end_node,
'最短路径长度': nx.dijkstra_path_length(G, start_node, end_node),
'经过的边编号': path_edges
}
rows.append(row)
df_result = pd.DataFrame(rows)
df_result.to_excel('all_pairs.xlsx', index=False)
```
修改后的代码中,我们在遍历最短路径时,记录下了经过的边的编号,并将其存储到结果表格的“经过的边编号”一列中。
Traceback (most recent call last): File "C:\Users\ying\Desktop\pythonProject5\main.py", line 84, in <module> end_times = nx.dag_late_finish(G, start_times) AttributeError: module 'networkx' has no attribute 'dag_late_finish'如何解决
这个错误是因为 `networkx` 模块中没有 `dag_late_finish` 属性。这个属性在较早的版本中可能存在,但在最新版本中已被删除。
你可以使用 `networkx` 中的 `topological_sort` 函数来实现类似的功能。下面是一个示例:
```python
import networkx as nx
G = nx.DiGraph()
# 添加节点和边
start_times = nx.topological_sort(G)
end_times = {node: max(end_times.get(child, 0) for child in G.successors(node)) + weight for node, weight in G.nodes(data="weight")}
```
在这个示例中,我们使用了 `topological_sort` 函数来获取拓扑排序后的节点列表,然后使用一个字典推导式计算每个节点的最晚完成时间。
你可以根据你的具体需求进行修改。
相关推荐
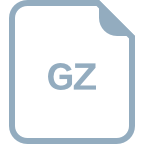









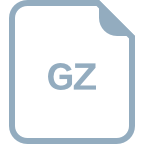