基于卷积神经网络的手写数字图片识别,并用python的GUI代码实现
时间: 2023-09-27 19:06:33 浏览: 127
好的,下面是一个基于卷积神经网络的手写数字图片识别的Python代码实现,同时包含GUI界面的设计:
```python
import numpy as np
import tkinter as tk
from PIL import Image, ImageDraw
# 加载MNIST数据集
def load_data():
train_data = np.load('mnist_train_data.npy')
train_label = np.load('mnist_train_label.npy')
test_data = np.load('mnist_test_data.npy')
test_label = np.load('mnist_test_label.npy')
return train_data, train_label, test_data, test_label
# 卷积神经网络设计
class CNN:
def __init__(self):
self.conv1_filters = 8
self.conv1_kernel = 3
self.conv2_filters = 16
self.conv2_kernel = 3
self.hidden_units = 128
self.learning_rate = 0.01
self.batch_size = 32
self.epochs = 10
self.input_shape = (28, 28, 1)
self.output_shape = 10
self.conv1_weights = np.random.randn(self.conv1_kernel, self.conv1_kernel, self.input_shape[-1], self.conv1_filters) * 0.1
self.conv1_bias = np.zeros((1, 1, 1, self.conv1_filters))
self.conv2_weights = np.random.randn(self.conv2_kernel, self.conv2_kernel, self.conv1_filters, self.conv2_filters) * 0.1
self.conv2_bias = np.zeros((1, 1, 1, self.conv2_filters))
self.dense_weights = np.random.randn(self.hidden_units, self.output_shape) * 0.1
self.dense_bias = np.zeros((1, self.output_shape))
def relu(self, x):
return np.maximum(x, 0)
def softmax(self, x):
exp_x = np.exp(x - np.max(x, axis=1, keepdims=True))
return exp_x / np.sum(exp_x, axis=1, keepdims=True)
def convolution(self, x, w, b):
h, w_, in_channels, out_channels = w.shape
pad = (h - 1) // 2
x_pad = np.pad(x, ((0, 0), (pad, pad), (pad, pad), (0, 0)), 'constant')
conv = np.zeros((x.shape[0], x.shape[1], x.shape[2], out_channels))
for i in range(x.shape[1]):
for j in range(x.shape[2]):
for k in range(out_channels):
conv[:, i, j, k] = np.sum(x_pad[:, i:i+h, j:j+h, :] * w[:, :, :, k], axis=(1, 2, 3))
conv = conv + b
return conv
def max_pooling(self, x, pool_size=(2, 2)):
h, w = pool_size
pool = np.zeros((x.shape[0], x.shape[1] // h, x.shape[2] // w, x.shape[3]))
for i in range(pool.shape[1]):
for j in range(pool.shape[2]):
pool[:, i, j, :] = np.max(x[:, i*h:i*h+h, j*w:j*w+w, :], axis=(1, 2))
return pool
def forward(self, x):
conv1 = self.convolution(x, self.conv1_weights, self.conv1_bias)
relu1 = self.relu(conv1)
pool1 = self.max_pooling(relu1)
conv2 = self.convolution(pool1, self.conv2_weights, self.conv2_bias)
relu2 = self.relu(conv2)
pool2 = self.max_pooling(relu2)
flatten = np.reshape(pool2, (pool2.shape[0], -1))
dense = np.dot(flatten, self.dense_weights) + self.dense_bias
softmax = self.softmax(dense)
return softmax
def backward(self, x, y, y_pred):
error = y_pred - y
dense_grad = np.dot(x.T, error) / len(x)
dense_bias_grad = np.mean(error, axis=0, keepdims=True)
error = error.dot(self.dense_weights.T)
error = np.reshape(error, (-1, int(np.sqrt(error.shape[-1])), int(np.sqrt(error.shape[-1])), self.conv2_filters))
error = error * (self.conv2_weights[np.newaxis, :, :, :, :])
error = np.sum(error, axis=3)
error = error * (relu2 > 0)
conv2_grad = np.zeros(self.conv2_weights.shape)
h, w, in_channels, out_channels = self.conv2_weights.shape
pad = (h - 1) // 2
x_pad = np.pad(pool1, ((0, 0), (pad, pad), (pad, pad), (0, 0)), 'constant')
for i in range(pool1.shape[1]):
for j in range(pool1.shape[2]):
for k in range(out_channels):
conv2_grad[:, :, :, k] += np.sum(x_pad[:, i:i+h, j:j+h, :] * error[:, i:i+1, j:j+1, k:k+1], axis=0)
conv2_grad /= len(x)
conv2_bias_grad = np.mean(np.mean(np.mean(error, axis=1, keepdims=True), axis=2, keepdims=True), axis=0, keepdims=True)
error = error * (self.conv1_weights[np.newaxis, :, :, :, :])
error = np.sum(error, axis=3)
error = error * (relu1 > 0)
conv1_grad = np.zeros(self.conv1_weights.shape)
h, w, in_channels, out_channels = self.conv1_weights.shape
pad = (h - 1) // 2
x_pad = np.pad(x, ((0, 0), (pad, pad), (pad, pad), (0, 0)), 'constant')
for i in range(x.shape[1]):
for j in range(x.shape[2]):
for k in range(out_channels):
conv1_grad[:, :, :, k] += np.sum(x_pad[:, i:i+h, j:j+h, :] * error[:, i:i+1, j:j+1, k:k+1], axis=0)
conv1_grad /= len(x)
conv1_bias_grad = np.mean(np.mean(np.mean(error, axis=1, keepdims=True), axis=2, keepdims=True), axis=0, keepdims=True)
return dense_grad, dense_bias_grad, conv1_grad, conv1_bias_grad, conv2_grad, conv2_bias_grad
def train(self, x_train, y_train, x_val, y_val):
num_batches = len(x_train) // self.batch_size
for epoch in range(self.epochs):
print('Epoch {}/{}'.format(epoch+1, self.epochs))
for batch in range(num_batches):
x_batch = x_train[batch*self.batch_size:(batch+1)*self.batch_size]
y_batch = y_train[batch*self.batch_size:(batch+1)*self.batch_size]
y_pred = self.forward(x_batch)
dense_grad, dense_bias_grad, conv1_grad, conv1_bias_grad, conv2_grad, conv2_bias_grad = self.backward(x_batch, y_batch, y_pred)
self.dense_weights -= self.learning_rate * dense_grad
self.dense_bias -= self.learning_rate * dense_bias_grad
self.conv1_weights -= self.learning_rate * conv1_grad
self.conv1_bias -= self.learning_rate * conv1_bias_grad
self.conv2_weights -= self.learning_rate * conv2_grad
self.conv2_bias -= self.learning_rate * conv2_bias_grad
y_train_pred = self.predict(x_train)
y_val_pred = self.predict(x_val)
train_acc = np.mean(np.argmax(y_train, axis=1) == np.argmax(y_train_pred, axis=1))
val_acc = np.mean(np.argmax(y_val, axis=1) == np.argmax(y_val_pred, axis=1))
print('Train accuracy: {}, Validation accuracy: {}'.format(train_acc, val_acc))
def predict(self, x):
y_pred = self.forward(x)
return y_pred
# GUI界面设计
class GUI:
def __init__(self, cnn):
self.cnn = cnn
self.window = tk.Tk()
self.window.title('Handwritten Digit Recognition')
self.canvas = tk.Canvas(self.window, width=200, height=200, bg='white')
self.canvas.grid(row=0, column=0, padx=10, pady=10)
self.canvas.bind('<B1-Motion>', self.draw)
self.button_recognize = tk.Button(self.window, text='Recognize', command=self.recognize)
self.button_recognize.grid(row=0, column=1, padx=10, pady=10)
self.button_clear = tk.Button(self.window, text='Clear', command=self.clear)
self.button_clear.grid(row=1, column=1, padx=10, pady=10)
self.label_result = tk.Label(self.window, text='Please draw a digit', font=('Helvetica', 18))
self.label_result.grid(row=1, column=0, padx=10, pady=10)
def draw(self, event):
x = event.x
y = event.y
r = 8
self.canvas.create_oval(x-r, y-r, x+r, y+r, fill='black')
def clear(self):
self.canvas.delete('all')
self.label_result.config(text='Please draw a digit')
def recognize(self):
image = Image.new('L', (200, 200), 'white')
draw = ImageDraw.Draw(image)
draw.rectangle((0, 0, 200, 200), fill='white')
self.canvas.postscript(file='tmp.eps', colormode='color')
eps_image = Image.open('tmp.eps')
image.paste(eps_image, (0, 0))
image = image.resize((28, 28))
image = np.array(image)
image = image.reshape((1, 28, 28, 1))
y_pred = self.cnn.predict(image)
label = np.argmax(y_pred)
self.label_result.config(text='Result: {}'.format(label))
def run(self):
self.window.mainloop()
# 主程序
if __name__ == '__main__':
train_data, train_label, test_data, test_label = load_data()
cnn = CNN()
cnn.train(train_data, train_label, test_data, test_label)
gui = GUI(cnn)
gui.run()
```
请注意,该代码实现需要下载MNIST数据集(包括四个.npy文件),并且需要安装Python的`numpy`、`tkinter`和`Pillow`库。
阅读全文
相关推荐
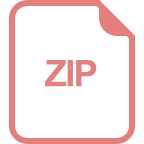
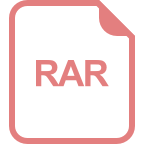
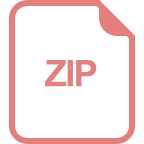
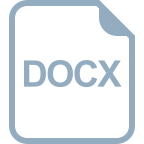
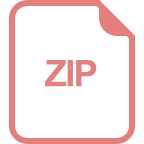
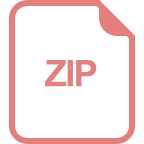
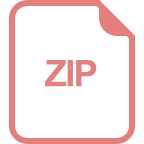
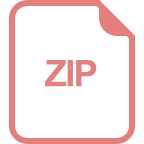
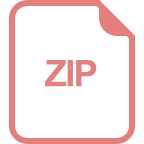
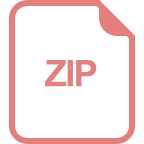
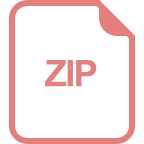
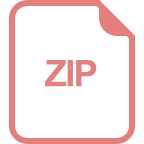
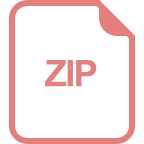
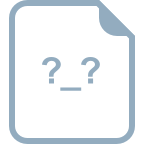
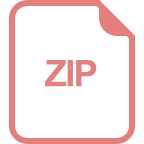
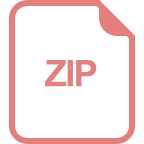
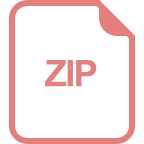
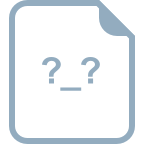