求解TSP问题,python实现,采用遗传算法其中选择算子使用竞标赛选择,请给出具体代码实现
时间: 2024-05-05 17:20:19 浏览: 15
以下是关于 TSP 问题的遗传算法实现的 Python 代码,包括竞标赛选择:
```python
import random
# 样本点列表,示例中为 5 个点
points = [(1,1), (2,3), (5,2), (7,6), (4,4)]
# 每个个体表示为一个排列,示例中为 [3, 1, 2, 4, 0]
init_population = [[i for i in range(len(points))] for j in range(10)]
# 计算代价函数,即路径总长度
def calculate_fitness(individual):
total_distance = 0
for i in range(len(individual)):
if i == len(individual) - 1:
total_distance += ((points[individual[i]][0] - points[individual[0]][0]) ** 2 + (points[individual[i]][1] - points[individual[0]][1]) ** 2) ** 0.5
else:
total_distance += ((points[individual[i]][0] - points[individual[i+1]][0]) ** 2 + (points[individual[i]][1] - points[individual[i+1]][1]) ** 2) ** 0.5
return total_distance
# 竞标赛选择算子
def tournament_selection(population, fitness_values, k):
selected_individual = None
selected_fitness = 0
for i in range(k):
index = random.randint(0, len(population)-1)
if fitness_values[index] > selected_fitness:
selected_individual = population[index]
selected_fitness = fitness_values[index]
return selected_individual
# 交叉算子,示例中使用 PMX 交叉
def pmx_crossover(parent1, parent2):
child1, child2 = parent1[:], parent2[:]
length = len(parent1)
point1 = random.randint(0, length-1)
point2 = random.randint(point1, length-1)
for i in range(point1, point2):
temp1, temp2 = child1[i], child2[i]
index1 = child1.index(temp2)
index2 = child2.index(temp1)
child1[i], child1[index1] = temp2, temp1
child2[i], child2[index2] = temp1, temp2
return child1, child2
# 变异算子,示例中使用交换变异
def swap_mutation(individual):
length = len(individual)
point1 = random.randint(0, length-1)
point2 = random.randint(0, length-1)
individual[point1], individual[point2] = individual[point2], individual[point1]
return individual
# 遗传算法主函数
def genetic_algorithm(population, fitness_func, tournament_size, crossover_rate, mutation_rate, max_generations):
for i in range(max_generations):
# 评估种群
fitness_values = [fitness_func(individual) for individual in population]
# 找出最优个体
best_index = fitness_values.index(min(fitness_values))
best_individual = population[best_index]
# 输出每代最优个体的路径长度
print('Generation {}: {}'.format(i+1, calculate_fitness(best_individual)))
# 进行竞标赛选择,生成新的子代种群
new_population = []
while len(new_population) < len(population):
parent1 = tournament_selection(population, fitness_values, tournament_size)
parent2 = tournament_selection(population, fitness_values, tournament_size)
if random.uniform(0, 1) < crossover_rate:
child1, child2 = pmx_crossover(parent1, parent2)
new_population.append(child1)
new_population.append(child2)
else:
new_population.append(parent1)
new_population.append(parent2)
# 进行变异操作
for j in range(len(new_population)):
if random.uniform(0, 1) < mutation_rate:
new_population[j] = swap_mutation(new_population[j])
# 更新种群
population = new_population
return population[best_index]
# 运行遗传算法
best_path = genetic_algorithm(init_population, calculate_fitness, 3, 0.8, 0.01, 10)
print('Best path: {}'.format(best_path))
```
其中,样本点列表 `points` 表示所有的点坐标,例如 `(1,1)` 表示横坐标为 1,纵坐标为 1 的点。`init_population` 是种群的初始化列表,其中每个个体表示为一个排列。
`calculate_fitness` 函数计算一个个体的代价函数,本例中使用的是路径总长度。`tournament_selection` 是竞标赛选择算子。`pmx_crossover` 是 PMX 交叉算子,交叉率为 `crossover_rate`。`swap_mutation` 是交换变异算子,变异率为 `mutation_rate`。
`genetic_algorithm` 是遗传算法的主函数,其中的参数包括竞标赛选择算子的比赛规模 `tournament_size`、交叉率 `crossover_rate`、变异率 `mutation_rate`、最大迭代次数 `max_generations` 等。其中,每代种群的最优个体的路径长度会被输出,最终的最优路径会被返回。
使用以上代码运行 TSP 问题的遗传算法,可以得到求解结果。
相关推荐
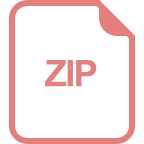
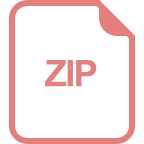
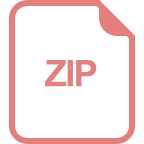














