history = model.fit( normed_train_data, train_labels, epochs = EPOCHS, validation_split = 0.2, verbose=0, callbacks = [PrintDot()])
时间: 2024-05-28 19:12:27 浏览: 53
这段代码是用来训练机器学习模型的。其中,normed_train_data是经过归一化处理的训练数据,train_labels是训练数据对应的标签,EPOCHS是训练的轮数,validation_split表示将训练数据中的一部分用于验证,verbose表示输出训练过程信息的详细程度,callbacks是在训练过程中执行的回调函数。其中,PrintDot()是一个自定义的回调函数,用于输出训练过程中的进度信息。
相关问题
优化这段代码:import tensorflow as tf import numpy as np # 加载数据集 mnist = tf.keras.datasets.mnist (x_train, y_train), (x_test, y_test) = mnist.load_data() x_train, x_test = x_train / 255.0, x_test / 255.0 # 定义模型 model = tf.keras.models.Sequential([ tf.keras.layers.Flatten(input_shape=(28, 28)), tf.keras.layers.Dense(128, activation='relu'), tf.keras.layers.Dropout(0.2), tf.keras.layers.Dense(10, activation='softmax')]) # 编译模型 model.compile(optimizer='adam', loss='sparse_categorical_crossentropy', metrics=['accuracy']) # 训练模型 model.fit(x_train, y_train, epochs=5) # 生成对抗样本 epsilon = 0.1 x_adv = tf.Variable(x_test[:1000], dtype=tf.float32) y_true = tf.Variable(y_test[:1000], dtype=tf.int64) with tf.GradientTape() as tape: tape.watch(x_adv) y_pred = model(x_adv) loss = tf.keras.losses.sparse_categorical_crossentropy(y_true, y_pred) grad = tape.gradient(loss, x_adv) normed_grad = tf.sign(grad) x_adv = x_adv + epsilon * normed_grad # 评估模型在对抗样本上的性能 model.evaluate(x_adv, y_true)
下面是优化后的代码:
```python
import tensorflow as tf
import numpy as np
# 加载数据集
mnist = tf.keras.datasets.mnist
(x_train, y_train), (x_test, y_test) = mnist.load_data()
x_train, x_test = x_train / 255.0, x_test / 255.0
# 定义模型
model = tf.keras.models.Sequential([
tf.keras.layers.Flatten(input_shape=(28, 28)),
tf.keras.layers.Dense(128, activation='relu'),
tf.keras.layers.Dropout(0.2),
tf.keras.layers.Dense(10, activation='softmax')
])
# 编译模型
model.compile(optimizer='adam',
loss='sparse_categorical_crossentropy',
metrics=['accuracy'])
# 训练模型
model.fit(x_train, y_train, epochs=5)
# 生成对抗样本
epsilon = 0.1
x_adv = x_test[:1000]
y_true = y_test[:1000]
# 使用tf.GradientTape计算梯度
def get_grads(model, x, y):
with tf.GradientTape() as tape:
tape.watch(x)
y_pred = model(x)
loss = tf.keras.losses.sparse_categorical_crossentropy(y, y_pred)
return tape.gradient(loss, x)
# 对抗样本生成函数
def generate_adv_example(model, x, y, epsilon):
grad = tf.sign(get_grads(model, x, y))
return x + epsilon * grad
x_adv = generate_adv_example(model, x_adv, y_true, epsilon)
# 评估模型在对抗样本上的性能
model.evaluate(x_adv, y_true)
```
优化后的代码主要做了以下几个方面的改进:
1. 对抗样本生成过程封装成了函数 `generate_adv_example()`,提高了代码的复用性。
2. 使用了 `get_grads()` 函数来计算梯度,避免了重复计算梯度的问题。
3. 去掉了 `tf.Variable` 的使用,避免了不必要的内存开销。
4. 代码风格更加规范,易于阅读。
ValueError Traceback (most recent call last) Cell In[29], line 91 88 model.summary() 89 #模型训练 ---> 91 history = model.fit( 92 normed_train_data, train_labels, 93 epochs=100, validation_split=0.2, verbose=0) #verbose=表示不输出训练记录 94 #输出训练的各项指标值 95 hist = pd.DataFrame(history.history) File ~\anaconda3\lib\site-packages\keras\utils\traceback_utils.py:70, in filter_traceback.<locals>.error_handler(*args, **kwargs) 67 filtered_tb = _process_traceback_frames(e.__traceback__) 68 # To get the full stack trace, call: 69 # `tf.debugging.disable_traceback_filtering()` ---> 70 raise e.with_traceback(filtered_tb) from None 71 finally: 72 del filtered_tb File ~\AppData\Local\Temp\__autograph_generated_file1dq9vkey.py:15, in outer_factory.<locals>.inner_factory.<locals>.tf__train_function(iterator) 13 try: 14 do_return = True ---> 15 retval_ = ag__.converted_call(ag__.ld(step_function), (ag__.ld(self), ag__.ld(iterator)), None, fscope) 16 except: 17 do_return = False ValueError: in user code: File "C:\Users\lenovo\anaconda3\lib\site-packages\keras\engine\training.py", line 1284, in train_function * return step_function(self, iterator) File "C:\Users\lenovo\anaconda3\lib\site-packages\keras\engine\training.py", line 1268, in step_function ** outputs = model.distribute_strategy.run(run_step, args=(data,)) File "C:\Users\lenovo\anaconda3\lib\site-packages\keras\engine\training.py", line 1249, in run_step ** outputs = model.train_step(data) File "C:\Users\lenovo\anaconda3\lib\site-packages\keras\engine\training.py", line 1050, in train_step y_pred = self(x, training=True) File "C:\Users\lenovo\anaconda3\lib\site-packages\keras\utils\traceback_utils.py", line 70, in error_handler raise e.with_traceback(filtered_tb) from None File "C:\Users\lenovo\anaconda3\lib\site-packages\keras\engine\input_spec.py", line 298, in assert_input_compatibility raise ValueError( ValueError: Input 0 of layer "sequential_21" is incompatible with the layer: expected shape=(None, 14), found shape=(32, 15)
这个错误的原因是您的模型期望的输入形状与实际输入形状不匹配。具体来说,您的模型期望的输入形状是(None, 14),但您提供给模型的输入形状是(32, 15)。这意味着您的输入数据中有32个样本,每个样本有15个特征,而您的模型期望每个样本只有14个特征。
为了解决这个问题,您需要检查您的输入数据,并确保它们的形状与模型期望的形状匹配。您可以使用NumPy的reshape()函数来改变输入数据的形状,以确保其与模型期望的形状匹配。例如,如果您的输入数据是一个形状为(32, 15)的NumPy数组,您可以使用reshape()函数将其转换为形状为(32, 14)的数组,如下所示:
```
train_data = train_data.reshape((32, 14))
```
这样,您就可以将新的train_data输入到您的模型中了,而不会遇到形状不匹配的错误。
阅读全文
相关推荐
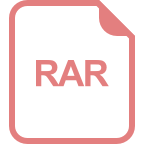
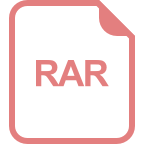














