刘二大人循环神经网络代码
时间: 2023-09-03 13:13:20 浏览: 87
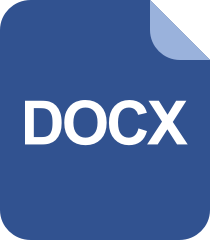
B站刘二大人Pytorch课程学习笔记及课后作业

循环神经网络的代码实现可以使用 Python 编程语言来完成。以下是一个简单的循环神经网络的 Python 代码示例,可以供您参考:
```python
import numpy as np
# 定义 sigmoid 激活函数
def sigmoid(x):
return 1 / (1 + np.exp(-x))
# 定义循环神经网络类
class RNN:
def __init__(self, input_size, output_size, hidden_size):
self.input_size = input_size
self.output_size = output_size
self.hidden_size = hidden_size
# 定义网络权重
self.Wxh = np.random.randn(hidden_size, input_size) * 0.01
self.Whh = np.random.randn(hidden_size, hidden_size) * 0.01
self.Why = np.random.randn(output_size, hidden_size) * 0.01
# 定义网络偏置
self.bh = np.zeros((hidden_size, 1))
self.by = np.zeros((output_size, 1))
# 定义前向传播函数
def forward(self, inputs):
h = np.zeros((self.hidden_size, 1))
self.last_inputs = inputs
self.last_hs = {0: h}
# 循环计算每个时间步的隐藏状态和输出
for i, x in enumerate(inputs):
h = sigmoid(np.dot(self.Wxh, x) + np.dot(self.Whh, h) + self.bh)
self.last_hs[i+1] = h
y = np.dot(self.Why, h) + self.by
return y
# 定义反向传播函数
def backward(self, dy):
n = len(self.last_inputs)
# 计算输出层的权重和偏置的梯度
dWhy = np.dot(dy, self.last_hs[n].T)
dby = dy
# 初始化隐藏层的梯度
dh = np.dot(self.Why.T, dy)
dhraw = (1 - self.last_hs[n] ** 2) * dh
# 计算隐藏层和输入层的权重和偏置的梯度
dWxh = np.zeros_like(self.Wxh)
dWhh = np.zeros_like(self.Whh)
dbh = np.zeros_like(self.bh)
for t in reversed(range(n)):
x = self.last_inputs[t]
dhraw_t = dhraw * (1 - self.last_hs[t+1] ** 2)
dWxh += np.dot(dhraw_t, x.T)
dWhh += np.dot(dhraw_t, self.last_hs[t].T)
dbh += dhraw_t
dhraw = np.dot(self.Whh.T, dhraw_t)
return dWxh, dWhh, dWhy, dbh, dby
# 定义更新网络参数的函数
def update(self, dWxh, dWhh, dWhy, dbh, dby, learning_rate):
self.Wxh -= learning_rate * dWxh
self.Whh -= learning_rate * dWhh
self.Why -= learning_rate * dWhy
self.bh -= learning_rate * dbh
self.by -= learning_rate * dby
# 定义训练函数
def train(self, inputs, targets, learning_rate):
# 前向传播
output = self.forward(inputs)
# 计算输出误差
dy = output - targets
# 反向传播
dWxh, dWhh, dWhy, dbh, dby = self.backward(dy)
# 更新网络参数
self.update(dWxh, dWhh, dWhy, dbh, dby, learning_rate)
return np.sum(dy ** 2)
# 定义预测函数
def predict(self, inputs):
output = self.forward(inputs)
return np.argmax(output)
```
在实际使用过程中,您需要根据具体的问题和数据集进行修改和调整。
阅读全文
相关推荐
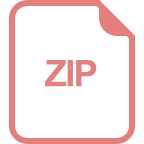


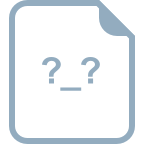
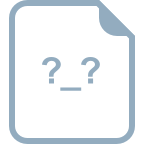
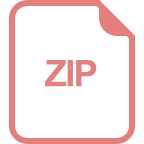
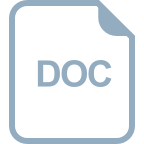








