from accelerate import notebook_launcher notebook_launcher(training_loop, args, num_processes=2)
时间: 2024-05-29 22:14:28 浏览: 150
这是一个使用 Accelerate 库的代码片段,它可以并行执行指定数量的训练进程,以加速模型训练过程。具体来说,这段代码从 `accelerate` 库中导入了 `notebook_launcher` 函数,该函数将训练循环 `training_loop` 和参数 `args` 作为输入,并指定了要使用的进程数量 `num_processes=2`。最后,该函数将训练循环并行执行,以提高训练速度。需要注意的是,这段代码适用于在 Jupyter Notebook 中运行的情况。
相关问题
from accelerate import Accelerator
The `accelerate` package is a Python library developed by Hugging Face that provides an easy-to-use API for distributed training and inference of deep learning models. The `Accelerator` class in this package is a wrapper around PyTorch or TensorFlow distributed training backends, which provides a unified interface for training models on multi-GPU and multi-node environments.
To use the `Accelerator` class, you first need to install the `accelerate` package by running `pip install accelerate`. Then, in your Python script, you can import the package and create an instance of the `Accelerator` class as follows:
```
from accelerate import Accelerator
accelerator = Accelerator()
```
This will automatically detect the available GPUs and configure the distributed training backend based on the number of GPUs and nodes. You can then use the `accelerator` instance to wrap your PyTorch or TensorFlow model and data loaders, and call the training and evaluation methods as usual. The `Accelerator` class will take care of parallelizing the computations across the available devices and nodes.
create LoRA network. base dim (rank): 64, alpha: 32 neuron dropout: p=None, rank dropout: p=None, module dropout: p=None create LoRA for Text Encoder: 72 modules. create LoRA for U-Net: 192 modules. enable LoRA for text encoder enable LoRA for U-Net Traceback (most recent call last): File "D:\lora_lian\sd-scripts\train_network.py", line 873, in <module> train(args) File "D:\lora_lian\sd-scripts\train_network.py", line 242, in train info = network.load_weights(args.network_weights) File "D:\lora_lian\sd-scripts\networks\lora.py", line 884, in load_weights info = self.load_state_dict(weights_sd, False) File "D:\lora_lian\python\lib\site-packages\torch\nn\modules\module.py", line 2041, in load_state_dict raise RuntimeError('Error(s) in loading state_dict for {}:\n\t{}'.format( RuntimeError: Error(s) in loading state_dict for LoRANetwork: size mismatch for lora_unet_mid_block_attentions_0_proj_out.lora_up.weight: copying a param with shape torch.Size([1280, 128, 1, 1]) from checkpoint, the shape in current model is torch.Size([1280, 64, 1, 1]). Traceback (most recent call last): File "D:\lora_lian\python\lib\runpy.py", line 196, in _run_module_as_main return _run_code(code, main_globals, None, File "D:\lora_lian\python\lib\runpy.py", line 86, in _run_code exec(code, run_globals) File "D:\lora_lian\python\lib\site-packages\accelerate\commands\launch.py", line 1114, in <module> main() File "D:\lora_lian\python\lib\site-packages\accelerate\commands\launch.py", line 1110, in main launch_command(args) File "D:\lora_lian\python\lib\site-packages\accelerate\commands\launch.py", line 1104, in launch_command simple_launcher(args) File "D:\lora_lian\python\lib\site-packages\accelerate\commands\launch.py", line 567, in simple_launcher raise subprocess.CalledProcessError(returncode=process.returncode, cmd=cmd) subprocess.CalledProcessError: Command '['D:\\lora_lian\\python\\python.exe', './sd-scripts/train_network.py', '--config_file', 'D:\\lora_lian\\toml\\autosave\\20230709-112914.toml']' returned non-zero exit status 1. Training failed / 训练失败
根据错误消息,训练失败的原因是模型加载权重时发生了大小不匹配的错误。具体来说,`lora_unet_mid_block_attentions_0_proj_out.lora_up.weight` 的形状在当前模型中为 `torch.Size([1280, 64, 1, 1])`,但从检查点加载的权重形状为 `torch.Size([1280, 128, 1, 1])`,导致了大小不匹配的错误。
要解决这个问题,你可以尝试以下几个步骤:
1. 检查训练脚本中是否指定了正确的模型配置和权重文件。确保使用的权重文件与当前模型兼容,并且没有发生错误或损坏。
2. 确保使用相同版本的训练脚本和权重文件。不同版本之间的模型结构可能会有所不同,导致加载权重时发生大小不匹配的错误。
3. 如果你有其他可用的权重文件,可以尝试加载它们,看看是否能解决问题。有时候,使用不同的预训练权重可以解决加载错误的问题。
4. 如果以上步骤都没有解决问题,你可能需要检查训练脚本和模型代码中的网络结构定义,确保它们一致。可能存在某个部分定义的网络结构与加载的权重文件不匹配的情况。
如果问题仍然存在,你可以尝试向开发者或相关社区寻求帮助。提供更多详细的信息,如完整的错误消息、使用的软件版本等,有助于其他人更好地帮助你解决问题。
阅读全文
相关推荐
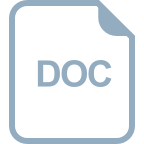
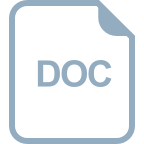
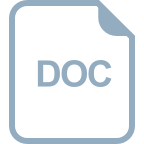
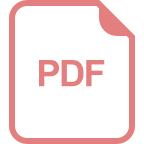
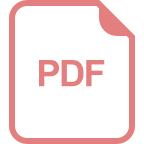
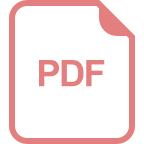
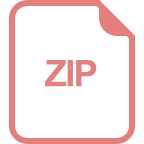
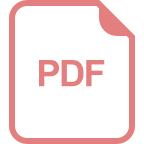
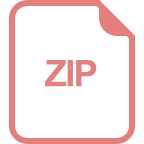
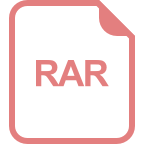
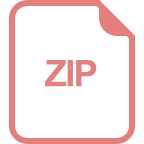






