提高随机森林模型得分代码
时间: 2024-10-30 22:06:37 浏览: 12
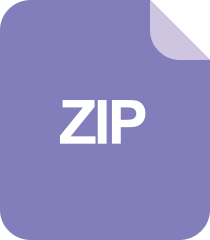
随机森林(C语言)
提高随机森林模型(Random Forest)得分通常涉及几个关键步骤,包括数据预处理、特征选择、参数调整以及评估模型性能。以下是一些常用的代码示例:
1. **导入必要的库**:
```python
import pandas as pd
from sklearn.ensemble import RandomForestClassifier
from sklearn.model_selection import train_test_split, GridSearchCV
from sklearn.metrics import accuracy_score, classification_report
```
2. **加载数据并预处理**:
```python
data = pd.read_csv('your_data.csv')
X = data.drop('target_column', axis=1) # 特征列
y = data['target_column'] # 目标变量
# 数据集分割(如80%训练,20%测试)
X_train, X_test, y_train, y_test = train_test_split(X, y, test_size=0.2, random_state=42)
```
3. **创建随机森林模型并初始化参数网格**:
```python
rf = RandomForestClassifier()
param_grid = {
'n_estimators': [50, 100, 200],
'max_depth': [None, 10, 20],
'min_samples_split': [2, 5, 10],
'min_samples_leaf': [1, 2, 4]
}
```
4. **使用GridSearchCV进行参数优化**:
```python
grid_search = GridSearchCV(rf, param_grid, cv=5, scoring='accuracy')
grid_search.fit(X_train, y_train)
best_rf = grid_search.best_estimator_
```
5. **模型评估**:
```python
y_pred = best_rf.predict(X_test)
print("Accuracy:", accuracy_score(y_test, y_pred))
print(classification_report(y_test, y_pred))
```
阅读全文
相关推荐
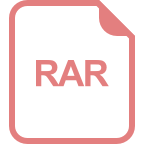
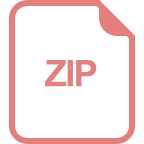


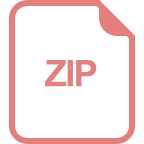
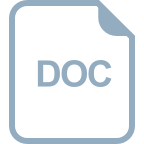
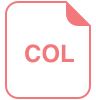
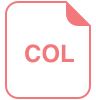
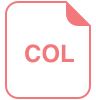
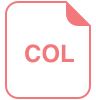
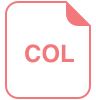
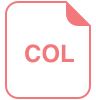





