dropout在pytorch,激活函数用Softmax
时间: 2023-11-04 19:04:41 浏览: 62
首先,dropout是一种在神经网络中防止过拟合的技术。在PyTorch中,可以通过在模型定义中添加`nn.Dropout`层来实现dropout。例如:
```
import torch.nn as nn
class MyModel(nn.Module):
def __init__(self):
super(MyModel, self).__init__()
self.fc1 = nn.Linear(10, 20)
self.dropout = nn.Dropout(0.5)
self.fc2 = nn.Linear(20, 5)
def forward(self, x):
x = self.fc1(x)
x = nn.functional.relu(x)
x = self.dropout(x)
x = self.fc2(x)
x = nn.functional.softmax(x, dim=1)
return x
```
在这个例子中,`nn.Dropout(0.5)`表示以0.5的概率随机将神经元输出置为0,从而防止过拟合。`nn.functional.softmax(x, dim=1)`则表示对输出进行softmax操作,得到每个类别的概率分布。
需要注意的是,softmax一般用于多分类问题,如果是二分类问题,可以使用sigmoid激活函数。
相关问题
PyTorch中还有哪些类似这样的函数
PyTorch中有很多类似affine_grid和grid_sample这样的函数,可以方便地进行张量操作和数学计算。以下是一些常用的函数:
1. torch.cat:用于沿着指定维度拼接张量。
2. torch.stack:用于沿着新的维度对张量序列进行堆叠。
3. torch.matmul:用于矩阵乘法。
4. torch.nn.functional.conv2d:用于二维卷积操作。
5. torch.nn.functional.max_pool2d:用于二维最大池化操作。
6. torch.nn.functional.dropout:用于随机失活操作。
7. torch.nn.functional.relu:用于ReLU激活函数。
8. torch.nn.functional.softmax:用于softmax函数。
这些函数可以大大简化模型的实现,提高代码效率和可读性,同时也可以帮助开发者更好地理解模型的内部机制。
用pytorch构建textcnn
### 回答1:
好的,下面是用PyTorch构建TextCNN的代码:
```python
import torch
import torch.nn as nn
import torch.nn.functional as F
class TextCNN(nn.Module):
def __init__(self, vocab_size, embedding_dim, num_classes, num_filters, filter_sizes):
super(TextCNN, self).__init__()
self.embedding = nn.Embedding(vocab_size, embedding_dim)
self.convs = nn.ModuleList([
nn.Conv2d(1, num_filters, (f, embedding_dim)) for f in filter_sizes
])
self.fc = nn.Linear(len(filter_sizes)*num_filters, num_classes)
def forward(self, x):
x = self.embedding(x) # [batch_size, seq_len, embedding_dim]
x = x.unsqueeze(1) # [batch_size, 1, seq_len, embedding_dim]
# Apply a convolution + max pool layer for each filter size
pooled_outputs = []
for conv in self.convs:
conv_out = F.relu(conv(x)) # [batch_size, num_filters, seq_len - filter_size + 1, 1]
pooled_out = F.max_pool2d(conv_out, (conv_out.shape[2], 1)).squeeze(3) # [batch_size, num_filters, 1]
pooled_outputs.append(pooled_out)
# Combine all the pooled features
x = torch.cat(pooled_outputs, dim=1) # [batch_size, len(filter_sizes)*num_filters]
x = self.fc(x) # [batch_size, num_classes]
return x
```
其中,`vocab_size`表示词汇表的大小,`embedding_dim`表示词向量的维度,`num_classes`表示分类的类别数,`num_filters`表示每个卷积核的数量,`filter_sizes`表示卷积核的大小列表。
模型的主体部分是一个卷积层和一个最大池化层的组合,对于每个卷积核大小,都会产生一个卷积层,然后把所有卷积层的输出拼接起来,再通过一个全连接层将其映射到类别概率输出。
### 回答2:
TextCNN(Text Convolutional Neural Network)是一种用于文本分类的深度学习模型,它结合了卷积神经网络(CNN)与文本特征提取的方法。在PyTorch中构建TextCNN模型可以按照以下步骤进行:
1. 导入所需的库和模块:
```python
import torch
import torch.nn as nn
import torch.nn.functional as F
```
2. 定义TextCNN类,继承自nn.Module类:
```python
class TextCNN(nn.Module):
def __init__(self, num_classes, vocab_size, embedding_dim, filter_sizes, num_filters, dropout_rate):
super(TextCNN, self).__init__()
# 定义Embedding层
self.embedding = nn.Embedding(vocab_size, embedding_dim)
# 定义卷积层
self.convs = nn.ModuleList([
nn.Conv2d(1, num_filters, (filter_size, embedding_dim)) for filter_size in filter_sizes
])
# 定义全连接层
self.fc = nn.Linear(num_filters * len(filter_sizes), num_classes)
# 定义dropout层
self.dropout = nn.Dropout(dropout_rate)
```
3. 定义前向传播函数:
```python
def forward(self, x):
x = self.embedding(x) # Embedding层
x = x.unsqueeze(1) # 增加维度以适应卷积层的输入
x = [F.relu(conv(x)).squeeze(3) for conv in self.convs] # 卷积层
x = [F.max_pool1d(conv, conv.size(2)).squeeze(2) for conv in x] # 池化层
x = torch.cat(x, 1) # 拼接所有池化层输出
x = self.dropout(x) # dropout层
x = self.fc(x) # 全连接层
return x
```
4. 创建TextCNN对象:
```python
num_classes = 10 # 分类类别数
vocab_size = 10000 # 词汇表大小
embedding_dim = 100 # 词向量维度
filter_sizes = [3, 4, 5] # 卷积核大小
num_filters = 100 # 卷积核数量
dropout_rate = 0.5 # dropout概率
model = TextCNN(num_classes, vocab_size, embedding_dim, filter_sizes, num_filters, dropout_rate)
```
通过以上步骤,我们可以使用PyTorch构建一个简单的TextCNN模型。请根据自己的实际需求和数据特点调整模型的参数和结构。
### 回答3:
TextCNN是一种用于文本分类任务的深度学习模型,可以通过使用PyTorch构建。PyTorch是一个开源的深度学习框架,可以提供高效的张量操作和自动微分。
首先,我们需要使用PyTorch构建TextCNN模型的网络结构。TextCNN由三个主要的组件构成:输入层、卷积层和全连接层。
输入层将文本数据转换为词向量,可以使用预训练的词向量模型(如Word2Vec或GloVe)来获取词向量。在PyTorch中,我们可以使用nn.Embedding层来实现这一步骤。
卷积层用于提取文本特征。我们可以使用不同大小的卷积核对文本进行卷积操作,并通过应用ReLU激活函数进行非线性变换。PyTorch提供了nn.Conv1d层来实现这一步骤。
全连接层将提取的特征映射到类别空间。我们可以使用nn.Linear层实现这一步骤,并通过softmax函数将输出转换为类别概率分布。
在模型训练过程中,我们可以使用PyTorch提供的优化器如Adam或SGD来更新模型的参数。我们还可以使用交叉熵损失函数来度量模型的分类性能。
最后,我们可以使用PyTorch的训练循环来训练TextCNN模型。训练循环包括数据加载、正向传播、计算损失、反向传播和参数更新等步骤。
总结来说,使用PyTorch构建TextCNN模型需要设计网络结构、选择优化器和损失函数以及实现训练循环。通过PyTorch的丰富功能,我们可以更轻松地构建和训练TextCNN模型,以达到准确分类文本的目标。
相关推荐
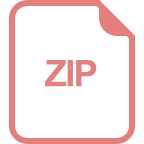
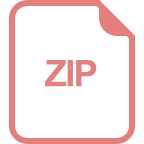












