ransac去除误匹配代码
时间: 2023-07-09 12:17:24 浏览: 106
以下是使用RANSAC算法去除误匹配的示例代码,其中假设我们有两个点集A和B,需要找到它们之间的对应关系:
```python
import numpy as np
from sklearn.linear_model import RANSACRegressor
# 假设我们有两个点集A和B,需要找到它们之间的对应关系
points_A = np.random.rand(10, 2) # 生成10个随机二维坐标
points_B = np.random.rand(10, 2)
# 构建RANSAC模型
model = RANSACRegressor()
# 训练模型并获取内点
model.fit(points_A, points_B)
inliers_A = points_A[model.inlier_mask_]
inliers_B = points_B[model.inlier_mask_]
# 输出去除误匹配后的内点
print('去除误匹配后的内点A:', inliers_A)
print('去除误匹配后的内点B:', inliers_B)
```
需要注意的是,这里使用的是`sklearn`库中的`RANSACRegressor`模型,如果需要使用其他类型的模型,则需要根据具体情况进行更改。
相关问题
PCL中ransac去除误匹配代码
以下是使用PCL库中RANSAC算法去除误匹配的示例代码,其中假设我们有两个点云A和B,需要找到它们之间的对应关系:
```cpp
#include <pcl/sample_consensus/ransac.h>
#include <pcl/sample_consensus/sac_model_registration.h>
#include <pcl/registration/transforms.h>
// 假设我们有两个点云A和B,需要找到它们之间的对应关系
pcl::PointCloud<pcl::PointXYZ>::Ptr cloudA(new pcl::PointCloud<pcl::PointXYZ>);
pcl::PointCloud<pcl::PointXYZ>::Ptr cloudB(new pcl::PointCloud<pcl::PointXYZ>);
// ... 从文件或传感器中读取点云数据,将数据存储到cloudA和cloudB中 ...
// 构建RANSAC模型
pcl::SampleConsensusModelRegistration<pcl::PointXYZ, pcl::PointXYZ>::Ptr model(new pcl::SampleConsensusModelRegistration<pcl::PointXYZ, pcl::PointXYZ>(cloudB));
pcl::RandomSampleConsensus<pcl::PointXYZ> ransac(model);
ransac.setDistanceThreshold(0.01);
ransac.computeModel();
Eigen::Matrix4f transform = ransac.getBestTransformation();
// 对点云A进行变换,去除误匹配
pcl::transformPointCloud(*cloudA, *cloudA, transform);
// 输出去除误匹配后的点云数据
pcl::PointCloud<pcl::PointXYZ>::Ptr cloudInliers(new pcl::PointCloud<pcl::PointXYZ>);
pcl::copyPointCloud(*cloudA, ransac.getInliers(), *cloudInliers);
std::cout << "去除误匹配后的点云A:" << std::endl;
for (int i = 0; i < cloudInliers->size(); ++i)
std::cout << "x: " << cloudInliers->points[i].x << ", y: " << cloudInliers->points[i].y << ", z: " << cloudInliers->points[i].z << std::endl;
```
需要注意的是,这里使用的是PCL库中的`SampleConsensusModelRegistration`模型和`RandomSampleConsensus`算法,如果需要使用其他类型的模型和算法,则需要根据具体情况进行更改。
pythonopencv利用ransac去除误匹配点
在使用OpenCV进行图像处理时,我们可以使用RANSAC算法去除误匹配点。RANSAC是一种基于随机采样的局部优化算法,可以解决数据中存在噪声或者异常值时的问题。
以下是一个简单的RANSAC去除误匹配点的示例代码:
```python
import numpy as np
import cv2
def ransacFilter(matches, keypoints1, keypoints2, threshold):
src_pts = np.float32([ keypoints1[m.queryIdx].pt for m in matches ]).reshape(-1,2)
dst_pts = np.float32([ keypoints2[m.trainIdx].pt for m in matches ]).reshape(-1,2)
# 使用RANSAC算法去除误匹配点
M, mask = cv2.findHomography(src_pts, dst_pts, cv2.RANSAC, threshold)
# 返回去除误匹配点后的匹配结果
good_matches = []
for i in range(len(matches)):
if mask[i][0] == 1:
good_matches.append(matches[i])
return good_matches
# 示例代码中,matches为初始匹配结果,keypoints1和keypoints2分别为两幅图像的特征点,threshold为阈值
```
在使用RANSAC算法时,需要先将初始匹配结果中的特征点坐标提取出来,并调用cv2.findHomography函数计算出变换矩阵M和匹配点的掩膜mask。通过掩膜mask可以得到去除误匹配点后的匹配结果。
阅读全文
相关推荐
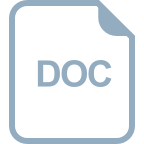
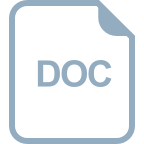
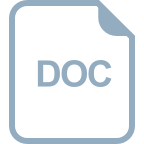
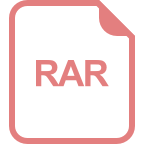
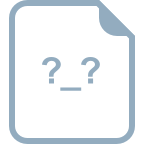
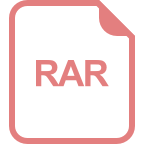
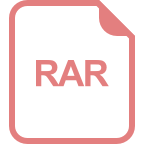
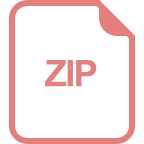




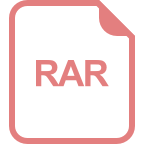
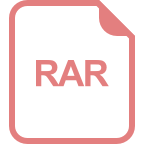
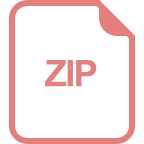
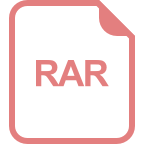
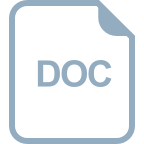