基于pytorch 生成一个vision transformer encoder
时间: 2023-10-09 11:13:14 浏览: 151
好的,可以使用 PyTorch 实现一个 Vision Transformer Encoder。首先,需要导入 PyTorch 和其他必要的库。
```python
import torch
import torch.nn as nn
import torch.nn.functional as F
```
接下来,定义一个 `PatchEmbedding` 类,用于将输入图像分割成大小为 `patch_size` 的小块,并将每个小块转换为向量。我们可以使用一个卷积层来实现这个过程。
```python
class PatchEmbedding(nn.Module):
def __init__(self, image_size=224, patch_size=16, in_channels=3, embed_dim=768):
super().__init__()
self.image_size = image_size
self.patch_size = patch_size
self.in_channels = in_channels
self.embed_dim = embed_dim
self.num_patches = (image_size // patch_size) ** 2
self.patch_embedding = nn.Conv2d(in_channels, embed_dim, kernel_size=patch_size, stride=patch_size)
def forward(self, x):
# Input shape: (batch_size, channels, height, width)
batch_size, channels, height, width = x.shape
assert height == width == self.image_size, f"Input image size must be {self.image_size}x{self.image_size}"
# Patch embedding
x = self.patch_embedding(x) # (batch_size, embed_dim, num_patches_h, num_patches_w)
x = x.flatten(2).transpose(1, 2) # (batch_size, num_patches, embed_dim)
return x
```
接下来,定义一个 `MultiHeadAttention` 类,用于实现多头自注意力机制。这里我们使用 PyTorch 中的 `MultiheadAttention` 模块。
```python
class MultiHeadAttention(nn.Module):
def __init__(self, embed_dim, num_heads, dropout=0.0):
super().__init__()
self.embed_dim = embed_dim
self.num_heads = num_heads
self.head_dim = embed_dim // num_heads
self.dropout = nn.Dropout(dropout)
self.qkv = nn.Linear(embed_dim, embed_dim * 3)
self.fc = nn.Linear(embed_dim, embed_dim)
self.scale = self.head_dim ** -0.5
def forward(self, x):
# Input shape: (batch_size, num_patches, embed_dim)
batch_size, num_patches, embed_dim = x.shape
# Compute queries, keys, and values
qkv = self.qkv(x).reshape(batch_size, num_patches, 3, self.num_heads, self.head_dim).permute(2, 0, 3, 1, 4)
q, k, v = qkv[0], qkv[1], qkv[2]
# Compute attention scores and attention weights
attn_scores = (q @ k.transpose(-2, -1)) * self.scale
attn_weights = F.softmax(attn_scores, dim=-1)
attn_weights = self.dropout(attn_weights)
# Compute the weighted sum of values
attn_output = attn_weights @ v
attn_output = attn_output.transpose(1, 2).reshape(batch_size, num_patches, embed_dim)
# Apply a linear layer and residual connection
x = self.fc(attn_output)
x = self.dropout(x)
x = x + attn_output
return x
```
接下来,定义一个 `FeedForward` 类,用于实现前馈神经网络。这里我们使用两个线性层和一个激活函数来实现。
```python
class FeedForward(nn.Module):
def __init__(self, embed_dim, hidden_dim, dropout=0.0):
super().__init__()
self.embed_dim = embed_dim
self.hidden_dim = hidden_dim
self.dropout = nn.Dropout(dropout)
self.fc1 = nn.Linear(embed_dim, hidden_dim)
self.fc2 = nn.Linear(hidden_dim, embed_dim)
def forward(self, x):
# Input shape: (batch_size, num_patches, embed_dim)
x = self.fc1(x)
x = F.gelu(x)
x = self.dropout(x)
x = self.fc2(x)
x = self.dropout(x)
x = x + x
return x
```
最后,定义一个 `TransformerEncoder` 类,它将上述三个模块组合在一起,实现 Vision Transformer Encoder 的功能。
```python
class TransformerEncoder(nn.Module):
def __init__(self, num_patches, embed_dim, num_heads, hidden_dim, dropout=0.0):
super().__init__()
self.num_patches = num_patches
self.embed_dim = embed_dim
self.num_heads = num_heads
self.hidden_dim = hidden_dim
self.patch_embedding = nn.Linear(3 * 16 * 16, embed_dim)
self.position_embedding = nn.Parameter(torch.randn(1, num_patches + 1, embed_dim))
self.dropout = nn.Dropout(dropout)
self.attention = MultiHeadAttention(embed_dim, num_heads, dropout)
self.feedforward = FeedForward(embed_dim, hidden_dim, dropout)
def forward(self, x):
# Input shape: (batch_size, channels, height, width)
x = self.patch_embedding(x)
x = x.permute(0, 2, 1)
x = torch.cat([self.position_embedding[:, :self.num_patches], x], dim=1)
x = self.dropout(x)
x = self.attention(x)
x = self.feedforward(x)
return x[:, 1:, :] # Remove the first token, which is the position embedding
```
现在,我们已经定义了一个 Vision Transformer Encoder。可以使用以下代码测试它:
```python
encoder = TransformerEncoder(num_patches=14 * 14, embed_dim=768, num_heads=12, hidden_dim=3072, dropout=0.1)
x = torch.randn(1, 3, 224, 224)
x = encoder(x)
print(x.shape) # Output shape: (1, 196, 768)
```
这个模型将一个大小为 $224 \times 224$ 的 RGB 图像转换为一个大小为 $196 \times 768$ 的向量序列。
阅读全文
相关推荐
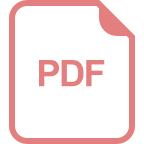
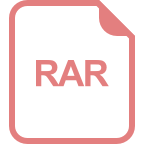
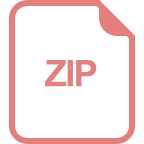

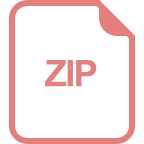
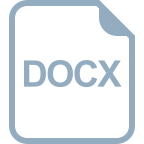
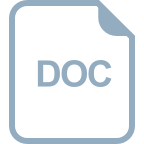
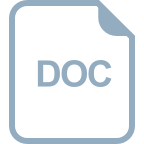
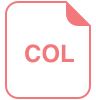
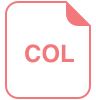
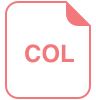
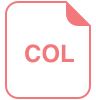
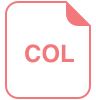
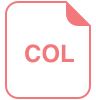
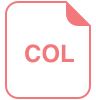
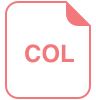
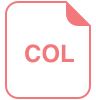

