x_test = x_test.astype( np.float )
时间: 2023-10-24 14:05:48 浏览: 92
`x_test.astype(np.float)` 是将 `x_test` 数组转换为 `np.float` 类型的操作。
`astype()` 是 NumPy 数组的方法,用于将数组的数据类型转换为指定的类型。在给定的代码中,`x_test.astype(np.float)` 将 `x_test` 数组中的元素转换为 `np.float` 类型。
这种类型转换可以用于确保数据在进行某些计算或操作时具有正确的数据类型。在这种情况下,将 `x_test` 数组中的元素转换为 `np.float` 类型可能是为了在后续的计算中使用浮点数运算。
相关问题
x = train['contents_clean'][:10000] y = train['label'][:10000] x_train, x_test, y_train, y_test = train_test_split(x,y,test_size=0.2) vec = TfidfVectorizer(ngram_range=(1, 2)) ####考虑二维的特征 临近的两个特征组合 X_train_vec = vec.fit_transform(x_train) X_test_vec = vec.transform(x_test) display(X_train_vec, X_test_vec) from sklearn.feature_selection import SelectKBest X_train_vec = X_train_vec.astype(np.float32) X_test_vec = X_test_vec.astype(np.float32) selector = SelectKBest(f_classif, k=min(20000, X_train_vec.shape[1])) selector.fit(X_train_vec, y_train) X_train_vec = selector.transform(X_train_vec) X_test_vec = selector.transform(X_test_vec) print(X_train_vec.shape, X_test_vec.shape)
这段代码是一个经典的文本分类流程,具体来说:
- 首先,从 `train` 数据集中选择前 10000 条数据作为训练集,分别将文本和标签存储在 `x` 和 `y` 变量中。
- 然后,使用 `train_test_split` 函数将训练集划分为训练集和测试集,其中测试集大小为训练集大小的 20%。
- 接下来,使用 `TfidfVectorizer` 对文本进行特征提取,其中 `ngram_range=(1, 2)` 表示提取单个词和二元组合的词作为特征。文本特征提取后,将训练集和测试集的特征向量分别存储在 `X_train_vec` 和 `X_test_vec` 变量中。
- 然后,使用 `SelectKBest` 进行特征选择,选择最相关的前 20000 个特征。这里使用的是 `f_classif` 评价函数,该函数计算每个特征与目标变量之间的方差比值(ANOVA F-value)。
- 最后,将训练集和测试集的特征向量转换为经过特征选择后的特征向量,并打印出它们的形状。
这段代码的目的是为了将原始的文本数据转换为可以用于机器学习模型训练的特征向量,并选择最相关的特征,以提高模型的性能。
X_test = [] Y_test = [] for filename in glob.glob(TEST_DATA_DIR + "*.png"): X_test.append(np.array(Image.open(filename))) Y_test.append(filename.lstrip(TEST_DATA_DIR).rstrip("*.png")) # list->numpy(rgb)->gray->normalization->fit keras X_test = np.array(X_test,dtype=np.float32) X_test = rgb2gray(X_test) X_test = X_test / 255 X_test,_ = fit_keras_channels(X_test) Y_test = list(Y_test) for i in range(len(Y_test)): Y_test[i] = text2vec(Y_test[i]) Y_test = np.asarray(Y_test) print(X_test.shape,type(X_test)) print(Y_test.shape,type(Y_test))
这段代码看起来是一个测试数据集的预处理过程。它首先使用glob库来读取一个文件夹中所有以.png结尾的文件,然后将每个文件读取为numpy数组,并将它们添加到X_test列表中。同时,文件名也被添加到Y_test列表中,并且经过一些处理(lstrip和rstrip),只保留了文件名中间的文本部分。接下来,X_test数组被转换为灰度图像,并进行了归一化处理。最后,Y_test列表中的每个文本标签也被转换为向量形式,并转换为numpy数组。最后打印出X_test和Y_test的形状和类型。
阅读全文
相关推荐
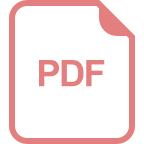












