cv2.imwrite(save_path_file,im)
时间: 2024-09-10 11:16:36 浏览: 61
`cv2.imwrite()` 是 OpenCV 提供的一个用于将图像保存到磁盘的函数。它的基本语法是:
```python
cv2.imwrite(path_to_save, image_data)
```
这里的参数含义如下:
- `save_path_file`(字符串):你要保存图像的完整文件路径,比如 "image.jpg"。这是函数期望输入的目标位置,OpenCV会尝试在此位置创建对应文件。
- `im`(numpy数组或Mat对象):这是待保存的图像数据,通常来自于 `cv2.imread()`、`cap.read()` 或其他OpenCV读取图像的函数返回的结果。
这个函数的作用是将 OpenCV 形式的图像数据按照指定的文件格式(由文件扩展名决定)保存到硬盘上。例如,如果你想保存一张BGR格式的图片为JPG格式,可以这样做:
```python
im = cv2.imread('input_image.png')
cv2.imwrite('output_image.jpg', im)
```
如果函数返回 `True`,说明保存成功;如果返回 `False` 或抛出异常,则可能是路径问题、权限不足,或者是 OpenCV 无法找到适当的编码器来保存该类型的图像。
相关问题
代码解释# Process detections for i, det in enumerate(pred): # detections per image if webcam: # batch_size >= 1 p, s, im0 = path[i], '%g: ' % i, im0s[i].copy() else: p, s, im0 = path, '', im0s save_path = str(Path(out) / Path(p).name) s += '%gx%g ' % img.shape[2:] # print string gn = torch.tensor(im0.shape)[[1, 0, 1, 0]] # normalization gain whwh if det is not None and len(det): # Rescale boxes from img_size to im0 size det[:, :4] = scale_coords(img.shape[2:], det[:, :4], im0.shape).round() # Print results for c in det[:, -1].unique(): n = (det[:, -1] == c).sum() # detections per class s += '%g %ss, ' % (n, names[int(c)]) # add to string # Write results for *xyxy, conf, cls in det: if save_txt: # Write to file xywh = (xyxy2xywh(torch.tensor(xyxy).view(1, 4)) / gn).view(-1).tolist() # normalized xywh with open(save_path[:save_path.rfind('.')] + '.txt', 'a') as file: file.write(('%g ' * 5 + '\n') % (cls, *xywh)) # label format if save_img or view_img: # Add bbox to image label = '%s %.2f' % (names[int(cls)], conf) if label is not None: if (label.split())[0] == 'person': people_coords.append(xyxy) # plot_one_box(xyxy, im0, line_thickness=3) plot_dots_on_people(xyxy, im0) # Plot lines connecting people distancing(people_coords, im0, dist_thres_lim=(100, 150)) # Print time (inference + NMS) print('%sDone. (%.3fs)' % (s, t2 - t1)) # Stream results if 1: ui.showimg(im0) if cv2.waitKey(1) == ord('q'): # q to quit raise StopIteration # Save results (image with detections) if save_img: if dataset.mode == 'images': cv2.imwrite(save_path, im0) else: if vid_path != save_path: # new video vid_path = save_path if isinstance(vid_writer, cv2.VideoWriter): vid_writer.release() # release previous video writer fps = vid_cap.get(cv2.CAP_PROP_FPS) w = int(vid_cap.get(cv2.CAP_PROP_FRAME_WIDTH)) h = int(vid_cap.get(cv2.CAP_PROP_FRAME_HEIGHT)) vid_writer = cv2.VideoWriter(save_path, cv2.VideoWriter_fourcc(*opt.fourcc), fps, (w, h)) vid_writer.write(im0)
这段代码是目标检测算法的输出结果处理部分。主要包括以下几个步骤:
1. 对每张图片的检测结果进行处理,包括将检测框从输入图像的尺寸缩放到输出图像的尺寸,并将结果写入文本文件中。
2. 对每个类别的检测结果统计数量,并将数量和类别名称添加到输出字符串中。
3. 对每个检测到的目标绘制边界框,并在边界框上标注类别和置信度。
4. 如果检测到的目标是人,则将其坐标保存在列表中,并在图像上绘制点和连线进行社交距离监测。
5. 将处理后的图像展示出来,并将图像保存到文件中。
yYOLOv5 val.py重写
重写 YOLOv5 的 val.py 可以通过以下步骤完成:
1. 导入必要的库和模块:
```
import argparse
import os
import time
import torch
import torch.backends.cudnn as cudnn
from numpy import random
from models.experimental import attempt_load
from utils.datasets import LoadImages
from utils.general import check_img_size, non_max_suppression, scale_coords
from utils.plots import plot_one_box
from utils.torch_utils import select_device, time_synchronized
```
2. 设置命令行参数并解析:
```
parser = argparse.ArgumentParser()
parser.add_argument('--weights', nargs='+', type=str, default='yolov5s.pt', help='model.pt path(s)')
parser.add_argument('--source', type=str, default='data/images', help='source') # file/folder, 0 for webcam
parser.add_argument('--img-size', type=int, default=640, help='inference size (pixels)')
parser.add_argument('--conf-thres', type=float, default=0.25, help='object confidence threshold')
parser.add_argument('--iou-thres', type=float, default=0.45, help='IOU threshold for NMS')
parser.add_argument('--max-det', type=int, default=1000, help='maximum number of detections per image')
parser.add_argument('--device', default='', help='cuda device, i.e. 0 or 0,1,2,3 or cpu')
parser.add_argument('--view-img', action='store_true', help='display results')
parser.add_argument('--save-txt', action='store_true', help='save results to *.txt')
parser.add_argument('--save-conf', action='store_true', help='save confidences in --save-txt labels')
parser.add_argument('--save-crop', action='store_true', help='save cropped prediction boxes')
parser.add_argument('--nosave', action='store_true', help='do not save images/videos')
parser.add_argument('--classes', nargs='+', type=int, help='filter by class: --class 0, or --class 0 2 3')
parser.add_argument('--agnostic-nms', action='store_true', help='class-agnostic NMS')
parser.add_argument('--augment', action='store_true', help='augmented inference')
parser.add_argument('--update', action='store_true', help='update all models')
parser.add_argument('--project', default='runs/detect', help='save results to project/name')
parser.add_argument('--name', default='exp', help='save results to project/name')
parser.add_argument('--exist-ok', action='store_true', help='existing project/name ok, do not increment')
opt = parser.parse_args()
```
3. 加载模型:
```
device = select_device(opt.device)
half = device.type != 'cpu' # half precision only supported on CUDA
# Load model
model = attempt_load(opt.weights, map_location=device) # load FP32 model
if half:
model.half() # to FP16
# Set Dataloader
vid_path, vid_writer = None, None
if opt.source.endswith('.txt'):
with open(opt.source, 'r') as f:
dataset = [x.strip() for x in f.readlines()]
elif opt.source.endswith(('mp4', 'avi', 'mov')):
vid_path = opt.source
if not os.path.exists(opt.source):
raise FileNotFoundError(f'File not found: {opt.source}')
dataset = [opt.source]
else:
dataset = LoadImages(opt.source, img_size=opt.img_size)
# Get names and colors
names = model.module.names if hasattr(model, 'module') else model.names
colors = [[random.randint(0, 255) for _ in range(3)] for _ in names]
```
4. 设置图片大小和推理模式:
```
imgsz = check_img_size(opt.img_size, s=model.stride.max()) # check img_size
if opt.device.type != 'cpu':
cudnn.benchmark = True # set True to speed up constant image size inference
```
5. 对每张图片进行推理:
```
for path, img, im0s, vid_cap in dataset:
t1 = time_synchronized()
# Get detections
img = torch.from_numpy(img).to(device)
if img.ndimension() == 3:
img = img.unsqueeze(0)
pred = model(img, augment=opt.augment)[0]
# Apply NMS
pred = non_max_suppression(pred, opt.conf_thres, opt.iou_thres, opt.classes, opt.agnostic_nms, max_det=opt.max_det)
t2 = time_synchronized()
# Process detections
for i, det in enumerate(pred): # detections per image
if webcam:
p, s, im0 = path[i], f'{i}: ', im0s[i].copy()
else:
p, s, im0 = path, '', im0s
save_path = str(Path(opt.project) / Path(p).name)
txt_path = str(Path(opt.project) / Path(p).stem) + ('' if dataset.mode == 'image' else f'_{dataset.count}.txt') # txt filename
s += '%gx%g ' % img.shape[2:] # print string
gn = torch.tensor(im0.shape)[[1, 0, 1, 0]] # normalization gain whwh
if det is not None and len(det):
# Rescale boxes from img_size to im0 size
det[:, :4] = scale_coords(img.shape[2:], det[:, :4], im0.shape).round()
# Print results
for c in det[:, -1].unique():
n = (det[:, -1] == c).sum() # detections per class
s += f"{n} {names[int(c)]}{'s' * (n > 1)}, " # add to string
# Write results
for *xyxy, conf, cls in reversed(det):
if opt.save_txt: # Write to file
xywh = (xyxy2xywh(torch.tensor(xyxy).view(1, 4)) / gn).view(-1).tolist() # normalized xywh
with open(txt_path, 'a') as f:
f.write(('%g ' * 5 + '\n') % (cls, *xywh, conf))
if opt.save_crop or opt.save_conf: # Save cropped prediction boxes
save_one_box(xyxy, im0, file=save_path, BGR=True)
if opt.view_img: # Add bbox to image
label = f'{names[int(cls)]} {conf:.2f}'
plot_one_box(xyxy, im0, label=label, color=colors[int(cls)], line_thickness=3)
# Print time (inference + NMS)
print(f'{s}Done. ({t2 - t1:.3f}s)')
# Stream results
if opt.view_img:
cv2.imshow(p, im0)
if cv2.waitKey(1) == ord('q'): # q to quit
raise StopIteration
```
6. 保存结果:
```
# Save results (image with detections)
if not opt.nosave:
if dataset.mode == 'images':
cv2.imwrite(save_path, im0)
else:
if vid_path != vid_writer: # new video
vid_writer = cv2.VideoWriter(save_path, cv2.VideoWriter_fourcc(*opt.fourcc), vid_cap.get(cv2.CAP_PROP_FPS), (round(vid_cap.get(cv2.CAP_PROP_FRAME_WIDTH)), round(vid_cap.get(cv2.CAP_PROP_FRAME_HEIGHT))))
vid_writer.write(im0)
```
以上就是重写 YOLOv5 的 val.py 的主要步骤,可以根据实际需求进行调整和修改。
阅读全文
相关推荐
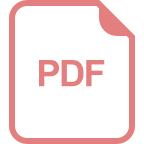
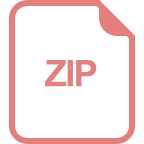
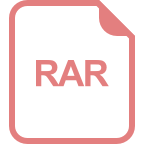
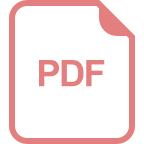




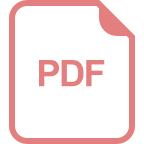
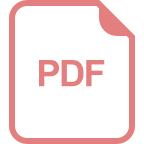
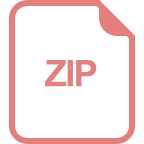