lstm+注意力机制(attention)实现时间序列预测(pytorch版)
时间: 2024-01-05 08:01:02 浏览: 755
LSTM注意力机制是一种用于时间序列预测的模型,它结合了长短期记忆网络(LSTM)和注意力机制。在PyTorch中实现这个模型需要几个步骤。
首先,我们需要定义LSTM模型,它可以通过PyTorch的nn.LSTM模块来实现。LSTM模型可以有效地捕捉时间序列数据中的长期依赖关系。
其次,我们需要定义注意力机制。在PyTorch中,可以使用nn.Linear层来实现注意力权重的计算,然后使用softmax函数来得到权重的归一化值。通过将注意力权重与LSTM模型的输出进行加权求和,可以得到增强后的表示。
接下来,我们需要定义损失函数和优化器。在时间序列预测任务中,可以使用均方误差作为损失函数,然后使用Adam优化器来更新模型参数。
最后,我们可以训练模型并进行预测。通过将时间序列数据输入到LSTM模型中,然后利用注意力机制来获取增强后的表示,最终可以得到时间序列的预测结果。
总之,利用PyTorch实现LSTM注意力机制模型进行时间序列预测是一个相对复杂的过程,但通过合理的模块定义和训练流程,可以有效地实现对时间序列数据的准确预测。
相关问题
使用lstm和attention进行时间序列预测的pytorch代码
在PyTorch中,LSTM (长短期记忆网络) 和注意力机制可以结合用于时间序列预测,如文本生成、股票价格预测等。以下是一个简单的示例代码片段,展示了如何构建一个基于LSTM和自注意力机制的模型:
```python
import torch
import torch.nn as nn
class Attention(nn.Module):
def __init__(self, hidden_size):
super(Attention, self).__init__()
self.hidden_size = hidden_size
self.attn = nn.Linear(hidden_size * 2, hidden_size)
self.v = nn.Parameter(torch.rand(hidden_size))
def forward(self, encoder_outputs, decoder_hidden):
# encoder_outputs形状为(Batch, Seq_len, Hidden_size)
# decoder_hidden形状为(Batch, Hidden_size)
seq_len = encoder_outputs.size(1)
attn_weights = torch.bmm(encoder_outputs, decoder_hidden.unsqueeze(1)).squeeze(1)
attn_weights = F.softmax(attn_weights, dim=1)
context = torch.bmm(attn_weights.unsqueeze(1), encoder_outputs).squeeze(1)
energy = torch.tanh(self.attn(torch.cat((context, decoder_hidden), 1)))
attention_score = torch.sum(self.v * energy, dim=1).unsqueeze(1)
return attn_weights, attention_score
class LSTMAttnModel(nn.Module):
def __init__(self, input_size, hidden_size, num_layers, dropout):
super(LSTMAttnModel, self).__init__()
self.lstm = nn.LSTM(input_size, hidden_size, num_layers, dropout=dropout)
self.attention = Attention(hidden_size)
self.fc_out = nn.Linear(hidden_size, output_size)
def forward(self, inputs, hidden):
lstm_output, hidden = self.lstm(inputs, hidden)
attn_weights, _ = self.attention(lstm_output, hidden[-1])
weighted_sum = torch.bmm(attn_weights.permute(0, 2, 1), lstm_output)
output = self.fc_out(weighted_sum.squeeze(1))
return output, hidden
# 初始化模型参数
input_size = ... # 输入序列特征维度
hidden_size = ... # LSTM隐藏层大小
num_layers = ... # LSTM层数
output_size = ... # 输出序列预测元素数量
dropout = 0.5
model = LSTMAttnModel(input_size, hidden_size, num_layers, dropout)
inputs = ... # 形状为(Batch, Seq_len, Input_size)
hidden = model.init_hidden(batch_size) # 初始化隐状态
# 进行前向传播并预测
output, _ = model(inputs, hidden)
```
lstm attention预测pytorch
LSTM Attention是一种基于LSTM和注意力机制的模型,用于序列预测任务。在PyTorch中,可以使用nn.LSTM和nn.MultiheadAttention来实现LSTM Attention模型。该模型可以在输入序列中自动学习重要的信息,并将其应用于预测任务中。通过使用注意力机制,模型可以更好地处理长序列和变长序列,并提高预测准确性。
阅读全文
相关推荐
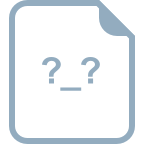
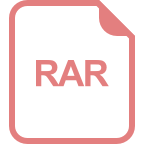
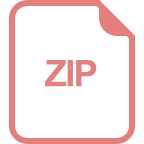
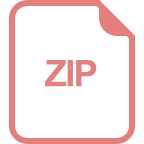
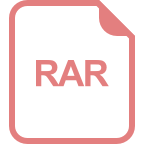
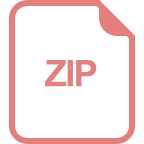
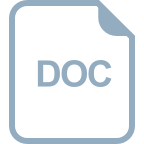
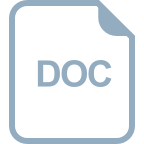
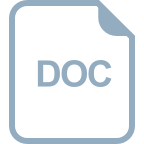
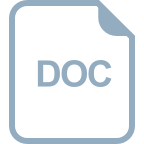

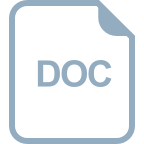
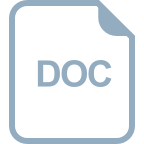
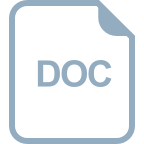
