p ython画玫瑰花的代码
时间: 2024-09-24 07:08:50 浏览: 59
Python可以使用turtle模块来绘制简单的图形,包括玫瑰花。以下是一个基本的例子,展示了如何使用循环和turtle库来绘制一个五瓣的玫瑰花:
```python
import turtle
# 创建turtle对象并设置名字
t = turtle.Turtle()
t.speed(0) # 设置速度为最快
# 定义颜色和花瓣角度
colors = ['red', 'orange', 'yellow', 'green', 'blue']
angle = 360 / len(colors)
# 绘制函数
def draw_petals():
for i in range(5):
t.color(colors[i % len(colors)]) # 循环使用颜色列表
t.forward(100)
t.right(angle)
# 开始绘制
draw_petals()
# 关闭窗口
turtle.done()
```
运行这段代码后,你会看到一个简化的五瓣玫瑰花出现在窗口上。如果你想创建更复杂的玫瑰图案,可能需要调整颜色组合、花瓣数以及花瓣间的旋转角度。
相关问题
p ython 判断玫瑰花
Python是一门广泛使用的高级编程语言,它支持多种编程范式,包括面向对象、命令式、函数式和过程式编程。在Python中,我们可以使用机器学习库如scikit-learn、TensorFlow或PyTorch来判断玫瑰花的种类。
通常,这类问题可以通过构建一个图像识别模型来解决。基本步骤如下:
1. 数据收集:首先需要收集不同种类玫瑰花的图片数据集。这些数据集应包含已标记的图片,即每张图片对应一种特定的玫瑰花种类。
2. 数据预处理:对于图像数据,需要进行一些预处理工作,如调整图片大小、归一化像素值、数据增强等,以提高模型的泛化能力。
3. 模型选择:根据问题的复杂程度,选择合适的机器学习模型。对于图像识别任务,常用的模型是卷积神经网络(CNN)。可以使用已有的预训练模型进行迁移学习,也可以从头开始构建模型。
4. 模型训练:使用收集的数据集对模型进行训练,调整参数以达到较高的准确率。
5. 模型评估:使用验证集和测试集评估模型的性能,确保模型在未知数据上也能有良好的表现。
6. 应用模型:训练完成并经过评估的模型可以用来对新的玫瑰花图片进行分类。
示例代码框架(使用scikit-learn)可能如下:
```python
from sklearn.datasets import load_iris
from sklearn.model_selection import train_test_split
from sklearn.preprocessing import StandardScaler
from sklearn.svm import SVC
from sklearn.metrics import accuracy_score
# 假设我们有标签化好的玫瑰花数据集
X, y = load_iris(return_X_y=True)
# 划分训练集和测试集
X_train, X_test, y_train, y_test = train_test_split(X, y, test_size=0.2, random_state=42)
# 数据标准化
scaler = StandardScaler()
X_train = scaler.fit_transform(X_train)
X_test = scaler.transform(X_test)
# 创建分类器
clf = SVC(kernel='linear') # 使用线性核函数
# 训练模型
clf.fit(X_train, y_train)
# 预测测试集
predictions = clf.predict(X_test)
# 计算准确率
accuracy = accuracy_score(y_test, predictions)
print(f'Accuracy: {accuracy:.2f}')
```
p ython画浪漫动态樱花代码
在Python中绘制浪漫动态的樱花效果通常会利用matplotlib库结合numpy生成随机数据并动态更新。这里是一个简单的例子,它将创建一个随时间变化的樱花动画:
```python
import numpy as np
import matplotlib.pyplot as plt
from matplotlib.animation import FuncAnimation
# 樱花花瓣坐标和颜色的数据生成
def get_sakura_points(num_points=50):
x = np.random.rand(num_points) * 4 - 2 # 随机x坐标
y = np.sin(x) + np.random.randn(num_points) * 0.2 # 随机y坐标,模拟花瓣上下波动
colors = plt.cm.spring(np.linspace(0, 1, num_points)) # 随机颜色
return x, y, colors
# 初始化图形
fig, ax = plt.subplots()
scat = ax.scatter([], [], c=[], s=100, cmap='spring', lw=0)
# 动画函数
def update(frame):
x, y, colors = get_sakura_points()
scat.set_offsets((x, y))
scat.set_array(colors)
return scat,
# 创建动画
ani = FuncAnimation(fig, update, frames=np.arange(0, 2*np.pi, 0.01), blit=True)
plt.title('动态樱花')
plt.show()
阅读全文
相关推荐
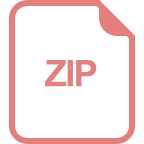












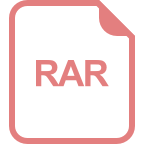