import os.path import pdb import time
时间: 2023-11-16 12:07:29 浏览: 150
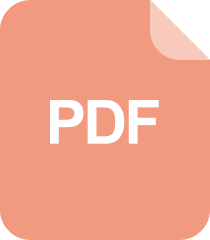
Python os模块学习笔记
import os.path import pdb import time 是Python中的标准库模块。其中,os.path提供了一些与文件路径和名称相关的方法,例如os.path.basename()可以返回一个路径的基本名称,如示例中的"c:\\Python\\a.txt"返回"a.txt"。pdb模块是用于调试Python代码的工具,可以在代码中设置断点并逐行执行以检查代码执行的过程。time模块提供了与时间相关的方法,例如time.time()可以返回当前时间的时间戳。
阅读全文
相关推荐
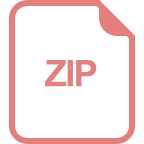
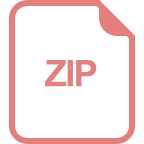

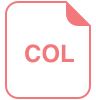



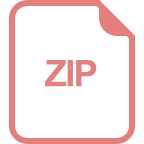
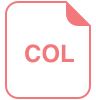
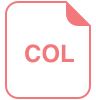







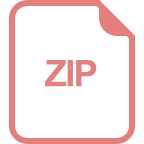