最速下降法求解最小二乘估计matlab
时间: 2024-10-24 22:00:42 浏览: 19
最速下降法(Gradient Descent)是一种常用的优化算法,用于寻找函数的局部最小值,在求解最小二乘估计问题中非常常见,特别是在MATLAB等数学软件中。最小二乘估计通常涉及到拟合数据,使得实际观测值与模型预测之间的误差平方和最小。
在MATLAB中,可以使用`fminunc`函数来应用最速下降法求解线性或非线性回归问题。假设我们有一个关于自变量x的数据集以及相应的因变量y,目标函数可能是形式化的残差平方和:
\[ \text{Minimize} \quad J(\theta) = \frac{1}{2m}\sum_{i=1}^{m}(h_\theta(x_i) - y_i)^2 \]
其中,\( h_\theta(x) \) 是线性模型 \( \hat{y} = \theta_0 + \theta_1 x \),\( \theta = [\theta_0, \theta_1]^T \) 是待估计的参数向量,\( m \) 是样本数。
使用`fminunc`的基本步骤如下:
1. 定义目标函数(损失函数),如上面所示。
2. 初始化参数向量θ。
3. 调用`fminunc(loss_function, initial_theta)`,loss_function是你之前定义的目标函数,initial_theta是初始猜测的参数。
4. `fminunc`会返回找到的最小化参数θ。
```matlab
function loss = loss_function(theta)
% 这里是计算损失的具体实现,比如使用X,y作为输入
model_output = theta(1) + theta(2)*X;
error = (model_output - y).^2; % 残差平方
loss = mean(error); % 平均误差作为损失
end
initial_theta = [0; 0]; % 初始的θ值
optimal_theta = fminunc(@loss_function, initial_theta);
```
阅读全文
相关推荐
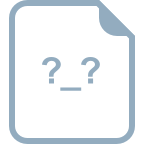
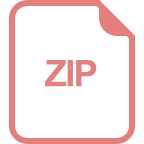
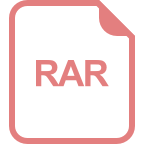


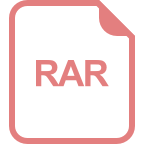
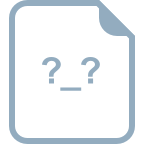
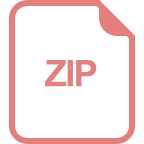
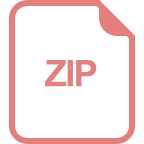
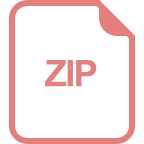
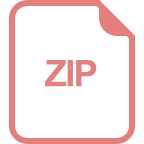
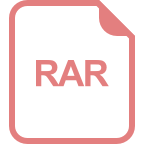
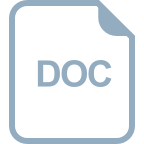
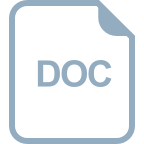
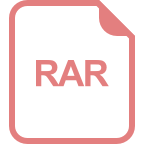
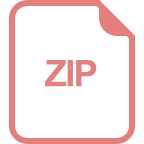
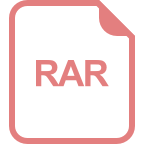
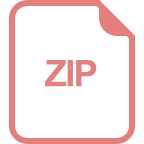
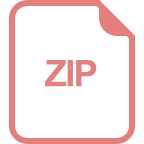