语义分割可以用什么代码
时间: 2024-01-13 15:02:44 浏览: 55
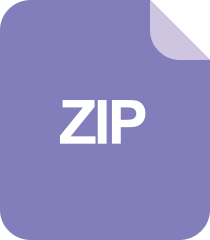
语义分割源代码

语义分割可以使用以下代码:
1. PyTorch:
```
import torch
import torchvision
import torchvision.transforms as transforms
# Load the dataset
transform = transforms.Compose(
[transforms.ToTensor(),
transforms.Normalize((0.5,), (0.5,))])
trainset = torchvision.datasets.MNIST(root='./data', train=True,
download=True, transform=transform)
trainloader = torch.utils.data.DataLoader(trainset, batch_size=4,
shuffle=True, num_workers=0)
# Define the model
import torch.nn as nn
import torch.nn.functional as F
class Net(nn.Module):
def __init__(self):
super(Net, self).__init__()
self.conv1 = nn.Conv2d(1, 6, 5)
self.pool = nn.MaxPool2d(2, 2)
self.conv2 = nn.Conv2d(6, 16, 5)
self.fc1 = nn.Linear(16 * 4 * 4, 120)
self.fc2 = nn.Linear(120, 84)
self.fc3 = nn.Linear(84, 10)
def forward(self, x):
x = self.pool(F.relu(self.conv1(x)))
x = self.pool(F.relu(self.conv2(x)))
x = x.view(-1, 16 * 4 * 4)
x = F.relu(self.fc1(x))
x = F.relu(self.fc2(x))
x = self.fc3(x)
return x
net = Net()
# Define the loss function and optimizer
import torch.optim as optim
criterion = nn.CrossEntropyLoss()
optimizer = optim.SGD(net.parameters(), lr=0.001, momentum=0.9)
# Train the model
for epoch in range(2): # loop over the dataset multiple times
running_loss = 0.0
for i, data in enumerate(trainloader, 0):
# get the inputs; data is a list of [inputs, labels]
inputs, labels = data
# zero the parameter gradients
optimizer.zero_grad()
# forward + backward + optimize
outputs = net(inputs)
loss = criterion(outputs, labels)
loss.backward()
optimizer.step()
# print statistics
running_loss += loss.item()
if i % 2000 == 1999: # print every 2000 mini-batches
print('[%d, %5d] loss: %.3f' %
(epoch + 1, i + 1, running_loss / 2000))
running_loss = 0.0
print('Finished Training')
```
2. TensorFlow:
```
import tensorflow as tf
# Load the dataset
mnist = tf.keras.datasets.mnist
(x_train, y_train), (x_test, y_test) = mnist.load_data()
x_train, x_test = x_train / 255.0, x_test / 255.0
# Define the model
model = tf.keras.models.Sequential([
tf.keras.layers.Flatten(input_shape=(28, 28)),
tf.keras.layers.Dense(128, activation='relu'),
tf.keras.layers.Dropout(0.2),
tf.keras.layers.Dense(10)
])
# Define the loss function and optimizer
loss_fn = tf.keras.losses.SparseCategoricalCrossentropy(from_logits=True)
optimizer = tf.keras.optimizers.Adam()
# Train the model
model.compile(optimizer=optimizer,
loss=loss_fn,
metrics=['accuracy'])
model.fit(x_train, y_train, epochs=5)
model.evaluate(x_test, y_test, verbose=2)
```
3. Caffe:
```
# Load the dataset
import caffe
import numpy as np
caffe.set_device(0)
caffe.set_mode_gpu()
solver = caffe.SGDSolver('/path/to/solver.prototxt')
# Define the model
net = caffe.Net('/path/to/deploy.prototxt', caffe.TEST)
# Define the loss function and optimizer
solver.net.blobs['data'].reshape(4, 1, 28, 28)
solver.net.blobs['label'].reshape(4,)
# Train the model
for i in range(10000):
solver.step(1)
if i % 1000 == 0:
print('iteration', i)
# Evaluate the model
test_net = caffe.Net('/path/to/deploy.prototxt', caffe.TEST)
test_net.copy_from('/path/to/weights.caffemodel')
test_net.blobs['data'].reshape(1, 1, 28, 28)
test_net.blobs['data'].data[...] = x_test[0:1, :, :, :]
out = test_net.forward()
print('predicted class:', out['prob'].argmax())
```
阅读全文
相关推荐
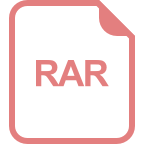
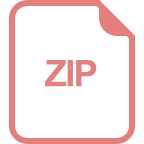
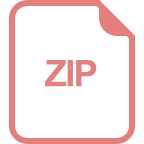
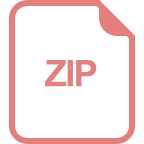
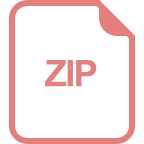
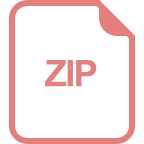
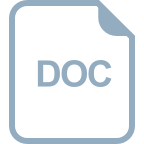








