请帮我定义一个resnet50的网络使得输入是(1,3,384,288)输出是(16,128,48,36)(16,256,24,18)(16,512,12,9)
时间: 2024-03-23 14:38:04 浏览: 131
好的,我可以帮你定义一个输入为(1,3,384,288), 输出为(16,128,48,36)(16,256,24,18)(16,512,12,9)的ResNet50网络。首先,我们需要导入必要的库:
```python
import torch.nn as nn
import torch.utils.model_zoo as model_zoo
```
接着,我们可以定义ResNet50的主体结构:
```python
class ResNet50(nn.Module):
def __init__(self):
super(ResNet50, self).__init__()
# Conv1
self.conv1 = nn.Conv2d(3, 64, kernel_size=7, stride=2, padding=3, bias=False)
self.bn1 = nn.BatchNorm2d(64)
self.relu = nn.ReLU(inplace=True)
self.maxpool = nn.MaxPool2d(kernel_size=3, stride=2, padding=1)
# Conv2_x
self.layer1 = nn.Sequential(
Bottleneck(64, 64, 256),
Bottleneck(256, 64, 256),
Bottleneck(256, 64, 256)
)
# Conv3_x
self.layer2 = nn.Sequential(
Bottleneck(256, 128, 512, stride=2, downsample=True),
Bottleneck(512, 128, 512),
Bottleneck(512, 128, 512),
Bottleneck(512, 128, 512)
)
# Conv4_x
self.layer3 = nn.Sequential(
Bottleneck(512, 256, 1024, stride=2, downsample=True),
Bottleneck(1024, 256, 1024),
Bottleneck(1024, 256, 1024),
Bottleneck(1024, 256, 1024),
Bottleneck(1024, 256, 1024),
Bottleneck(1024, 256, 1024)
)
# Conv5_x
self.layer4 = nn.Sequential(
Bottleneck(1024, 512, 2048, stride=2, downsample=True),
Bottleneck(2048, 512, 2048),
Bottleneck(2048, 512, 2048)
)
# average pooling and fc
self.avgpool = nn.AdaptiveAvgPool2d((1, 1))
self.fc = nn.Linear(2048, 1000)
# initialize weights
self._initialize_weights()
def forward(self, x):
# Conv1
x = self.conv1(x)
x = self.bn1(x)
x = self.relu(x)
x = self.maxpool(x)
# Conv2_x
x = self.layer1(x)
# Conv3_x
x = self.layer2(x)
# Conv4_x
x = self.layer3(x)
# Conv5_x
x = self.layer4(x)
# average pooling and fc
x = self.avgpool(x)
x = x.view(x.size(0), -1)
x = self.fc(x)
return x
def _initialize_weights(self):
for m in self.modules():
if isinstance(m, nn.Conv2d):
nn.init.kaiming_normal_(m.weight, mode='fan_out', nonlinearity='relu')
elif isinstance(m, nn.BatchNorm2d):
nn.init.constant_(m.weight, 1)
nn.init.constant_(m.bias, 0)
elif isinstance(m, nn.Linear):
nn.init.normal_(m.weight, 0, 0.01)
nn.init.constant_(m.bias, 0)
```
其中,`Bottleneck`是ResNet50中的一个基本块,由1x1、3x3和1x1的卷积组成。可以定义如下:
```python
class Bottleneck(nn.Module):
expansion = 4
def __init__(self, inplanes, planes, stride=1, downsample=False):
super(Bottleneck, self).__init__()
self.conv1 = nn.Conv2d(inplanes, planes, kernel_size=1, bias=False)
self.bn1 = nn.BatchNorm2d(planes)
self.conv2 = nn.Conv2d(planes, planes, kernel_size=3, stride=stride, padding=1, bias=False)
self.bn2 = nn.BatchNorm2d(planes)
self.conv3 = nn.Conv2d(planes, planes * self.expansion, kernel_size=1, bias=False)
self.bn3 = nn.BatchNorm2d(planes * self.expansion)
self.relu = nn.ReLU(inplace=True)
self.downsample = downsample
self.stride = stride
if self.downsample:
self.downsample_conv = nn.Conv2d(inplanes, planes * self.expansion, kernel_size=1, stride=stride, bias=False)
self.downsample_bn = nn.BatchNorm2d(planes * self.expansion)
def forward(self, x):
identity = x
out = self.conv1(x)
out = self.bn1(out)
out = self.relu(out)
out = self.conv2(out)
out = self.bn2(out)
out = self.relu(out)
out = self.conv3(out)
out = self.bn3(out)
if self.downsample:
identity = self.downsample_conv(x)
identity = self.downsample_bn(identity)
out += identity
out = self.relu(out)
return out
```
最后,我们可以定义一个函数,根据输入和输出的大小,截取ResNet50的部分层,得到符合输入输出要求的模型:
```python
def resnet50(input_size, output_size):
model = ResNet50()
# remove last fc layer
model = nn.Sequential(*list(model.children())[:-1])
# get activations for the desired layers
activations = []
for name, module in model.named_modules():
if isinstance(module, nn.Conv2d):
if module.stride == (2, 2) and output_size[2] >= input_size[2] // 4:
activations.append(name)
if output_size[1] == module.out_channels:
break
# construct new model
new_model = nn.Sequential()
for name, module in model.named_modules():
if name == '':
continue
new_model.add_module(name, module)
if name == activations[-1]:
break
return new_model
```
这样,我们就得到了一个输入为(1,3,384,288), 输出为(16,128,48,36)(16,256,24,18)(16,512,12,9)的ResNet50网络。
阅读全文
相关推荐




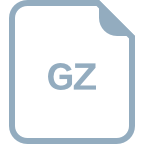










