Input X contains infinity or a value too large for dtype('float64')
时间: 2023-09-28 11:12:45 浏览: 244
这个错误提示表明,你的输入数据包含了无穷大(infinity)或过大的数值,导致无法将其转换为float64类型的数据。
出现这种情况的原因可能是数据中存在一些极端值或异常值,例如某些极大的数值或除以0的情况,这些情况可能会导致数据溢出或无限大。
如果你想处理这种情况,可以先使用numpy库的isinf()函数和isnan()函数,检查输入数据中是否包含无穷大或NaN值,然后将这些值替换为一个合适的值,例如0或平均值。示例代码如下:
```python
import numpy as np
# 假设X为输入数据,包含无穷大或NaN值
X = np.array([[1, 2, 3], [4, np.inf, 6], [7, 8, np.nan]])
# 检查输入数据中是否包含无穷大或NaN值
isinf = np.isinf(X)
isnan = np.isnan(X)
# 将无穷大或NaN值替换为0或平均值
X[isinf] = 0
X[isnan] = np.mean(X[~isnan])
# 输出处理后的数据
print(X)
```
以上代码使用了numpy库的isinf()函数和isnan()函数,检查输入数据中是否包含无穷大或NaN值,然后将这些值替换为0或平均值。注意,这里使用了~isnan()过滤掉了NaN值,以防止平均值受到NaN值的影响。
相关问题
ValueError:Input X contains infinity or a value too large for dtype('float64
这个错误通常是由于输入数据中包含无穷大或超出浮点数范围的数值而引起的。要解决这个问题,你可以尝试以下几种方法:
1. 检查输入数据:确保输入数据中没有包含无穷大或超出浮点数范围的数值。可以使用函数如`np.isinf()`和`np.isnan()`来检查数据是否包含无穷大或NaN值,并进行相应的处理。
2. 数据缩放:如果你的数据范围过大,可以考虑对数据进行缩放,将其归一化到一个合适的范围内。可以使用函数如`sklearn.preprocessing.MinMaxScaler`或`sklearn.preprocessing.StandardScaler`来进行数据缩放。
3. 数据类型转换:如果你确定数据中没有无穷大或超出浮点数范围的数值,并且错误仍然存在,你可以尝试将数据类型转换为更大范围的浮点数类型。例如,将`float64`转换为`float128`。
4. 数据预处理:根据具体情况,你可能需要对数据进行一些预处理操作,例如去除异常值、填充缺失值等。
如果以上方法都无法解决问题,建议提供更多关于你的具体问题和代码的细节,以便更好地帮助你解决该错误。
ValueError: Input X contains infinity or a value too large for dtype('float64')
This error occurs when the input data (X) contains a value that is either infinity or too large to be handled by the float64 data type. This can happen if the input data is not properly preprocessed or if there is an issue with the data source.
To fix this error, you can try the following solutions:
1. Check the input data for any values that may be causing the error. Look for any NaN or infinite values and remove or replace them with appropriate values.
2. Scale down the input data to a smaller range that can be handled by float64 data type. You can use techniques such as normalization or standardization to achieve this.
3. Check the data source for any issues, such as incorrect data formats or missing values. Fix any issues before passing the data to the model.
4. Try using a different data type, such as float32 or float16, if the data is not too large. However, keep in mind that using a smaller data type may result in a loss of precision.
阅读全文
相关推荐
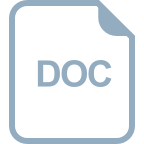
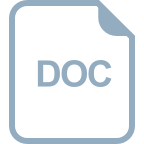
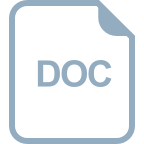







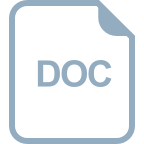
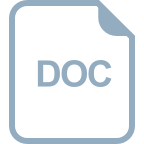
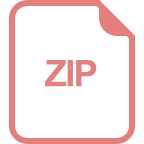
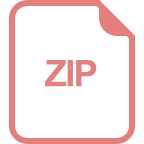