完成填空 # 数据预处理 te = TransactionEncoder() te_ary = #对数据进行转换 data = pd.DataFrame(te_ary, columns=te.columns_) # 挖掘频繁项集 frequent_itemsets = # 根据频繁项集生成关联规则 rules = # 输出关联规则 print("关联规则:\n", rules[['antecedents', 'consequents', 'support', 'confidence']])
时间: 2024-01-28 19:04:18 浏览: 88
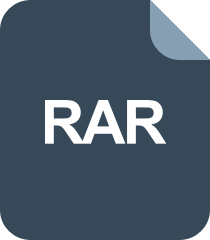
频繁项及关联规则的挖掘
填空:
关联规则挖掘是一种常用的数据挖掘技术,可以用于发现数据集中项之间的关联关系。其中,频繁项集是指在数据集中经常出现的项的集合,而关联规则是指项之间的关联关系,其中包括前项和后项以及它们之间的支持度和置信度等信息。
因此,可以使用 `mlxtend.frequent_patterns` 模块中的 `apriori` 和 `association_rules` 函数来进行关联规则挖掘。具体步骤如下:
```python
from mlxtend.preprocessing import TransactionEncoder
from mlxtend.frequent_patterns import apriori, association_rules
# 对数据进行转换
te = TransactionEncoder()
te_ary = te.fit_transform(data)
data = pd.DataFrame(te_ary, columns=te.columns_)
# 挖掘频繁项集
frequent_itemsets = apriori(data, min_support=0.05, use_colnames=True)
# 根据频繁项集生成关联规则
rules = association_rules(frequent_itemsets, metric="confidence", min_threshold=0.5)
# 输出关联规则
print("关联规则:\n", rules[['antecedents', 'consequents', 'support', 'confidence']])
```
其中,`min_support` 参数表示频繁项集的最小支持度,`use_colnames` 参数表示使用列名而非列索引来表示项集,`metric` 参数表示用于评估关联规则的质量指标,`min_threshold` 参数表示关联规则的最小阈值。
阅读全文
相关推荐
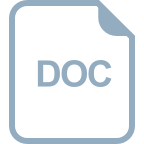
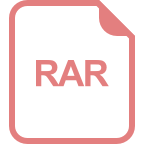






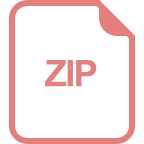
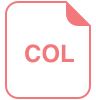







