tensorflow怎么用gpu训练
时间: 2023-05-31 18:19:01 浏览: 146
### 回答1:
要使用GPU训练TensorFlow模型,需要确保您的计算机上安装了适当的GPU驱动程序和CUDA工具包。然后,您可以使用以下代码片段将TensorFlow配置为使用GPU:
```
import tensorflow as tf
# 创建一个TensorFlow会话
sess = tf.Session()
# 创建一个TensorFlow设备对象,指定使用GPU
device = tf.device("/gpu:")
# 在设备上运行TensorFlow操作
with device:
# 在此处编写您的TensorFlow代码
```
在上面的代码中,您可以将`/gpu:`替换为您计算机上可用的其他GPU设备的名称。然后,您可以在`with device:`块中编写您的TensorFlow代码,以确保它在GPU上运行。
### 回答2:
TensorFlow 是一个非常流行的深度学习框架,它支持在 GPU 上进行训练和推理。在本文中,我们将介绍如何使用 TensorFlow 在 GPU 上进行训练。
首先,我们需要检查您的计算机是否符合以下要求:
1. NVIDIA GPU。TensorFlow 支持 NVIDIA GPU,您的计算机上必须要有 NVIDIA 显卡。
2. NVIDIA CUDA 工具包。TensorFlow 使用 CUDA 工具包与 NVIDIA 显卡进行通信。
3. NVIDIA cuDNN 库。cuDNN 是 NVIDIA 的深度学习库,它可以提高 TensorFlow 在 GPU 上的性能。
完成这些设置后,您可以使用以下步骤在 TensorFlow 中使用 GPU 进行训练:
1. 获取 TensorFlow。您可以从 TensorFlow 官方网站获取 TensorFlow。
2. 安装 TensorFlow。您可以使用 pip 安装 TensorFlow,命令如下:
`pip install tensorflow-gpu`
3. 在 TensorFlow 中启用 GPU 加速。运行以下代码:
```
import tensorflow as tf
physical_devices = tf.config.list_physical_devices('GPU')
tf.config.experimental.set_memory_growth(physical_devices[0], True)
```
这个代码段会列出计算机上的物理 GPU,并将内存增长设置为动态分配。
4. 编写 TensorFlow 代码。现在,您已经准备好编写能够在 GPU 上进行训练的 TensorFlow 代码了。下面是一个简单的示例代码:
```
import tensorflow as tf
physical_devices = tf.config.list_physical_devices('GPU')
tf.config.experimental.set_memory_growth(physical_devices[0], True)
# 加载数据
(train_images, train_labels), (test_images, test_labels) = tf.keras.datasets.mnist.load_data()
# 数据预处理
train_images = train_images / 255.0
test_images = test_images / 255.0
# 构建模型
model = tf.keras.Sequential([
tf.keras.layers.Flatten(input_shape=(28, 28)),
tf.keras.layers.Dense(128, activation='relu'),
tf.keras.layers.Dropout(0.2),
tf.keras.layers.Dense(10, activation='softmax')
])
# 编译模型
model.compile(optimizer='adam',
loss='sparse_categorical_crossentropy',
metrics=['accuracy'])
# 训练模型
model.fit(train_images, train_labels, epochs=5)
# 评估模型
test_loss, test_acc = model.evaluate(test_images, test_labels, verbose=2)
print('\nTest accuracy:', test_acc)
```
5. 运行 TensorFlow 代码。您可以在命令行中输入以下命令来运行 TensorFlow 代码:
```
python your_tensorflow_code.py
```
在这个例子中,您应该能够在 GPU 上训练模型并获得比在 CPU 上训练更快的速度。
在 TensorFlow 中使用 GPU 进行训练可能需要进行一些额外的设置,但是一旦设置完成,您就可以享受到 GPU 提供的加速和性能改进,从而提高深度学习的训练速度和准确度。
### 回答3:
TensorFlow是一个开源的机器学习框架,它支持使用GPU来加速训练。在使用GPU时,我们需要了解以下几点:
1. 安装CUDA和cuDNN
在使用GPU训练前,我们需要安装CUDA和cuDNN。CUDA是一种并行计算方式的平台,它可以使我们的GPU参与计算任务。cuDNN是NVIDIA Deep Learning SDK里面的一个支持深度神经网络的库,可以加速神经网络训练。在安装时需要注意CUDA和cuDNN的版本兼容性问题。
2. 安装TensorFlow GPU版
为了在TensorFlow中使用GPU,我们需要安装TensorFlow的GPU版。安装方法可以在TensorFlow官网上找到对应的安装指南。
3. 编写TensorFlow代码
TensorFlow支持Python语言编写代码。在代码中,我们需要指定使用GPU进行计算。TensorFlow使用with tf.device('/gpu:0')语句用GPU计算张量。同时我们也可以使用tf.ConfigProto()函数对TensorFlow进行GPU配置,如设置GPU的个数等。
4. 运行TensorFlow代码
在安装CUDA、cuDNN和TensorFlow GPU版后,我们可以将代码运行在支持CUDA的GPU上。在运行代码前,需要确保我们的GPU可以支持CUDA和cuDNN。
总之,为了在TensorFlow中使用GPU进行训练,我们需要安装CUDA、cuDNN和TensorFlow GPU版,编写支持GPU计算的TensorFlow代码,并在支持CUDA的GPU上运行代码。这样可以大大加快模型的训练速度和提升训练的效率。
阅读全文
相关推荐
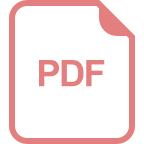
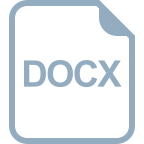


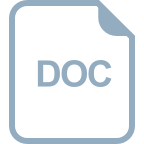



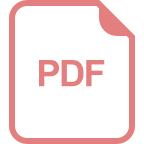
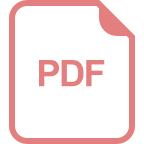
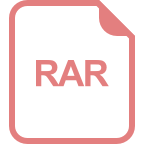






