def preprocess_sentence(sentence): return sentence.lower().split()解读一下代码
时间: 2024-05-19 17:17:58 浏览: 111
这段代码定义了一个名为 preprocess_sentence 的函数,它接收一个字符串参数 sentence,并执行以下操作:
1. 将字符串全部转换成小写字母。
2. 用空格将字符串分割成一个单词列表。
3. 返回这个单词列表作为处理后的结果。
这个函数主要用于对输入的句子进行预处理,以便后续处理和分析。
相关问题
import nltk.corpus import pandas as pd import re import matplotlib.pyplot as plt import seaborn as sns from stanfordcorenlp import StanfordCoreNLP # 导入数据 df = pd.read_csv('D:/file document/desktop/语料库大作业/Tweets.csv', usecols=['airline_sentiment', 'text']) def sentiment(x): if x == 'positive': return 1 elif x == 'negative': return -1 else: return 0 from nltk.corpus import stopwords from nltk.stem import SnowballStemmer from nltk.tokenize import RegexpTokenizer # 去除停用词 stopwords = nltk.corpus.stopwords.words('english') # 词还原 stemmer = SnowballStemmer('english') # 分词 tokenizer = RegexpTokenizer(r'\w+') # As this dataset is fetched from twitter so it has lots of people tag in tweets # we will remove them tags = r"@\w*" def preprocess_text(sentence, stem=False): # 去除text中一些影响文本分析的标签 sentence = [re.sub(tags, "", sentence)] text = [] for word in sentence: if word not in stopwords: if stem: text.append(stemmer.stem(word).lower()) else: text.append(word.lower()) return tokenizer.tokenize(" ".join(text)) # 将用preprocess_text() 函数处理后的text列保存回原始 DataFrame 的 text 列中 df['text'] = df['text'].map(preprocess_text) output_file = 'D:/file document/desktop/语料库大作业/output2.csv' # 输出文件路径 nlp = StanfordCoreNLP(r"D:/AppData/stanfordnlp", lang="en") # 定义函数,用于对指定文本进行依存句法分析 def dependency_parse(sentence): result = nlp.dependency_parse(sentence) return result # 对某一列进行依存句法分析,并将结果保存到新的一列中 df['dependency_parse'] = df['text'].apply(lambda x: dependency_parse(" ".join(x))) # 将结果保存到输出文件中 df.to_csv(output_file, index=False) nlp.close()优化这段代码
这段代码的主要任务是对一份推特数据集进行预处理,并对推特文本进行依存句法分析。以下是对该代码进行优化的一些建议:
1. 对导入的模块进行适当的组织和注释,以增加代码的可读性。
2. 将数据集的路径和输出文件的路径定义为变量,以方便修改。
3. 在进行依存句法分析之前,应该先将文本数据转换为字符串类型,以免引起类型错误。
4. 将StanfordCoreNLP的连接和关闭放在 with 语句块中,以确保它在使用后自动关闭连接。
5. 改进 preprocess_text() 函数,使其更加健壮和可读。
6. 使用 pandas 的 apply() 函数来遍历数据集,而不是使用 for 循环。
7. 将依存句法分析的结果转换为字符串类型,以便于保存到输出文件中。
下面是针对上述优化建议修改后的代码:
```
import re
import nltk
import pandas as pd
from nltk.corpus import stopwords
from nltk.stem import SnowballStemmer
from nltk.tokenize import RegexpTokenizer
from stanfordcorenlp import StanfordCoreNLP
# Define file paths
input_file = 'D:/file document/desktop/语料库大作业/Tweets.csv'
output_file = 'D:/file document/desktop/语料库大作业/output2.csv'
# Define sentiment function
def sentiment(x):
if x == 'positive':
return 1
elif x == 'negative':
return -1
else:
return 0
# Define preprocessing functions
stopwords = set(stopwords.words('english'))
stemmer = SnowballStemmer('english')
tokenizer = RegexpTokenizer(r'\w+')
tags = r"@\w*"
def preprocess_text(sentence, stem=False):
sentence = re.sub(tags, "", sentence)
words = tokenizer.tokenize(sentence)
words = [word.lower() for word in words if word.lower() not in stopwords]
if stem:
words = [stemmer.stem(word) for word in words]
return words
# Load data
df = pd.read_csv(input_file, usecols=['airline_sentiment', 'text'])
# Preprocess text
df['text'] = df['text'].apply(lambda x: preprocess_text(x))
# Connect to StanfordCoreNLP
with StanfordCoreNLP(r"D:/AppData/stanfordnlp", lang="en") as nlp:
# Define function for dependency parsing
def dependency_parse(sentence):
result = nlp.dependency_parse(str(sentence))
return str(result)
# Apply dependency parsing to text column and save results to new column
df['dependency_parse'] = df['text'].apply(lambda x: dependency_parse(x))
# Save preprocessed data to output file
df.to_csv(output_file, index=False)
```
在优化后的代码中,我们将数据集的路径和输出文件的路径定义为变量,以方便修改和维护。同时,我们对代码进行了适当的注释和组织,以增加代码的可读性。我们也改进了 preprocess_text() 函数,使其更加健壮和可读。最后,我们还使用了 with 语句块来管理 StanfordCoreNLP 的连接和关闭,以确保它在使用后自动关闭连接。
请在注释处填入代码完成对训练集和测试集的结巴分词from paddlenlp.datasets import load_dataset def read(data_path): data_set = [] with open(data_path, 'r', encoding='utf-8') as f: for line in f: l = line.strip('\n').split('\t') if len(l) != 2: print (len(l), line) words, labels = line.strip('\n').split('\t') data_set.append((words,labels)) return data_set train_ds = read(data_path='train.txt') dev_ds = read(data_path='dev.txt') test_ds = read(data_path='test.txt') for i in range(5): print("sentence %d" % (i), train_ds[i][0]) print("sentence %d" % (i), train_ds[i][1]) print(len(train_ds),len(dev_ds)) import jieba def data_preprocess(corpus): data_set = [] ####填结巴分词代码 for text in corpus: seg_list = jieba.cut(text) data_set.append(" ".join(seg_list)) return data_set train_corpus = data_preprocess(train_ds) test_corpus = data_preprocess(test_ds) print(train_corpus[:2]) print(test_corpus[:2])
from paddlenlp.datasets import load_dataset
def read(data_path):
data_set = []
with open(data_path, 'r', encoding='utf-8') as f:
for line in f:
l = line.strip('\n').split('\t')
if len(l) != 2:
print (len(l), line)
words, labels = line.strip('\n').split('\t')
data_set.append((words,labels))
return data_set
train_ds = read(data_path='train.txt')
dev_ds = read(data_path='dev.txt')
test_ds = read(data_path='test.txt')
for i in range(5):
print("sentence %d" % (i), train_ds[i][0])
print("sentence %d" % (i), train_ds[i][1])
print(len(train_ds),len(dev_ds))
import jieba
def data_preprocess(corpus):
data_set = []
for text in corpus:
seg_list = jieba.cut(text[0])
data_set.append((" ".join(seg_list), text[1]))
return data_set
train_corpus = data_preprocess(train_ds)
test_corpus = data_preprocess(test_ds)
print(train_corpus[:2])
print(test_corpus[:2])
阅读全文
相关推荐
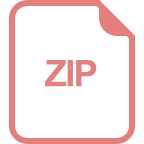
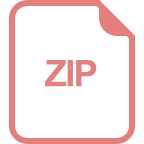
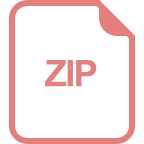
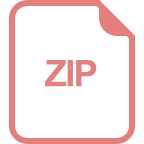
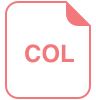
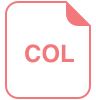










