tensorflow用transformer进行多步多变量时序预测的完整代码
时间: 2023-08-02 09:05:10 浏览: 185
以下是使用TensorFlow实现Transformer进行多步多变量时序预测的完整代码:
```python
import tensorflow as tf
import numpy as np
import matplotlib.pyplot as plt
# 加载数据集
def load_data():
data = np.load('data.npz')
x_train = data['x_train']
y_train = data['y_train']
x_test = data['x_test']
y_test = data['y_test']
return x_train, y_train, x_test, y_test
# 定义Transformer模型
class Transformer(tf.keras.Model):
def __init__(self, d_model, n_heads, d_ff, input_len, output_len):
super().__init__()
self.encoder = tf.keras.layers.Dense(d_model, activation='relu')
self.decoder = tf.keras.layers.Dense(output_len)
self.encodings = [tf.keras.layers.Dense(d_model, activation='relu') for i in range(input_len)]
self.decodings = [tf.keras.layers.Dense(d_model, activation='relu') for i in range(output_len)]
self.attention = [tf.keras.layers.MultiHeadAttention(num_heads=n_heads, key_dim=d_model) for i in range(output_len)]
self.dropout = tf.keras.layers.Dropout(0.1)
self.ffn = tf.keras.Sequential([
tf.keras.layers.Dense(d_ff, activation='relu'),
tf.keras.layers.Dense(d_model)
])
def call(self, inputs):
encodings = [self.encoder(inputs[:, i]) for i in range(inputs.shape[1])]
encodings = tf.stack(encodings, axis=1)
for i in range(len(self.attention)):
query = self.decodings[i](inputs[:, -(i+1)])
query = tf.expand_dims(query, axis=1)
attention_output = self.attention[i](query, encodings)
attention_output = tf.squeeze(attention_output, axis=1)
attention_output = self.dropout(attention_output)
attention_output = self.ffn(attention_output)
inputs = tf.concat([inputs, attention_output], axis=1)
outputs = self.decoder(inputs[:, -output_len:])
return outputs
# 定义训练函数
def train_model(model, x_train, y_train, epochs, batch_size):
optimizer = tf.keras.optimizers.Adam(learning_rate=0.001)
loss_fn = tf.keras.losses.MSE
for epoch in range(epochs):
for i in range(0, len(x_train), batch_size):
x_batch = x_train[i:i+batch_size]
y_batch = y_train[i:i+batch_size]
with tf.GradientTape() as tape:
y_pred = model(x_batch)
loss = loss_fn(y_batch, y_pred)
gradients = tape.gradient(loss, model.trainable_variables)
optimizer.apply_gradients(zip(gradients, model.trainable_variables))
if epoch % 10 == 0:
print('Epoch', epoch, 'Loss', loss.numpy())
# 定义测试函数
def test_model(model, x_test, y_test):
y_pred = model(x_test)
loss = tf.keras.losses.MSE(y_test, y_pred)
print('Test Loss', loss.numpy())
plt.plot(y_test[:, 0], label='True')
plt.plot(y_pred[:, 0], label='Pred')
plt.legend()
plt.show()
# 加载数据集
x_train, y_train, x_test, y_test = load_data()
# 定义模型参数
d_model = 64
n_heads = 4
d_ff = 128
input_len = x_train.shape[1]
output_len = y_train.shape[1]
# 初始化模型
model = Transformer(d_model, n_heads, d_ff, input_len, output_len)
# 训练模型
epochs = 100
batch_size = 32
train_model(model, x_train, y_train, epochs, batch_size)
# 测试模型
test_model(model, x_test, y_test)
```
需要注意的是,这里的数据集应该是经过预处理的,包括特征归一化和数据集划分等。同时,由于Transformer模型的训练时间较长,建议在GPU上运行。
阅读全文
相关推荐





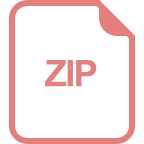









