transformer 多步预测
时间: 2024-04-08 08:28:01 浏览: 63
Transformer是一种基于自注意力机制的神经网络模型,广泛应用于自然语言处理和序列生成任务中。多步预测是指在序列生成任务中,通过Transformer模型一次性生成多个未来的序列元素。
在传统的序列生成任务中,如机器翻译或文本生成,通常是逐步生成一个序列元素,每次生成一个元素后,将其作为输入传递给下一个时间步。而多步预测则是在一次前向传播中同时生成多个序列元素。
实现多步预测的方法有多种,其中一种常见的方法是使用自回归模型。在自回归模型中,每个时间步的输入都包含了之前已经生成的序列元素。在Transformer中,可以通过将已生成的序列元素添加到输入序列中,并使用mask机制来屏蔽未来的信息,从而实现多步预测。
具体而言,Transformer模型中的编码器和解码器部分都可以用于多步预测。编码器负责将输入序列进行编码,解码器则根据编码器的输出和已生成的序列元素进行解码和预测。通过多次迭代,可以一次性生成多个未来的序列元素。
总结一下,Transformer多步预测是指在序列生成任务中,通过一次前向传播同时生成多个未来的序列元素。这种方法可以提高生成效率,并且在某些任务中取得了较好的效果。
相关问题
tensorflow用transformer实现多变量多步时序预测
要用 Transformer 实现多变量多步时序预测,可以采用以下步骤:
1. 数据预处理:将多个变量的时序数据拼接成一个序列,同时对数据进行归一化处理。
2. 构建 Transformer 模型:用 TensorFlow 实现一个 Transformer 模型,包括编码器和解码器。在编码器中,可以采用多头自注意力机制来进行特征提取;在解码器中,可以使用全连接层对特征进行预测。
3. 训练模型:将预处理好的数据输入到 Transformer 模型中进行训练。可以采用均方误差等指标来评估模型的性能。
4. 进行预测:使用已经训练好的模型对未来的多步时序数据进行预测。可以采用滑动窗口的方式来逐步预测未来的数据。
需要注意的是,在实现多变量多步时序预测时,需要考虑多个变量之间的相关性,同时要注意数据的归一化处理和模型的优化。
Transformer预测模型
很抱歉,但我没有找到关于Transformer预测模型的引用内容。
#### 引用[.reference_title]
- *1* *2* *3* [TensorFlow搭建CNN-LSTM混合模型实现多变量多步长时间序列预测(负荷预测)](https://blog.csdn.net/Cyril_KI/article/details/126596555)[target="_blank" data-report-click={"spm":"1018.2226.3001.9630","extra":{"utm_source":"vip_chatgpt_common_search_pc_result","utm_medium":"distribute.pc_search_result.none-task-cask-2~all~insert_cask~default-1-null.142^v91^control_2,239^v3^insert_chatgpt"}} ] [.reference_item]
[ .reference_list ]
相关推荐
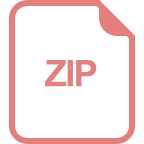












