写一段onehot编码的LSTM模型时间序列预测模型代码
时间: 2023-05-27 07:02:26 浏览: 135
抱歉,由于我是AI语言模型,无法自己编写和训练代码。以下是一个Python代码示例,可以用于实现时间序列预测的LSTM模型,其中包括one-hot编码。
```
import numpy as np
import tensorflow as tf
# Generate dummy data
data = np.random.randint(0, 10, size=(1000, 1))
labels = data[1:]
# One-hot encode data
X = np.zeros((len(data), 10))
X[np.arange(len(data)), data.reshape(-1)] = 1
# Define model architecture
model = tf.keras.models.Sequential([
tf.keras.layers.LSTM(128, input_shape=(1, 10)),
tf.keras.layers.Dense(10)
])
# Compile model
model.compile(loss='mse', optimizer='adam')
# Train model
model.fit(X[:-1].reshape(-1, 1, 10), labels, epochs=10)
# Make predictions
predictions = model.predict(X[-1].reshape(-1, 1, 10))
print(predictions)
```
相关问题
请帮我写一段lstm模型预测时间序列的代码
LSTM模型可以用来预测时间序列,下面是一段Python代码示例:import numpy as np
from keras.layers import LSTM# 定义时间序列数据
data = np.array([[i for i in range(100)]])# 定义模型
model = Sequential()
model.add(LSTM(20, input_shape=(1, 100)))
model.add(Dense(1))# 训练模型
model.compile(loss='mean_squared_error', optimizer='adam')
model.fit(data, data, epochs=1, batch_size=1, verbose=2)# 预测
predicted = model.predict(data)
print(predicted)
请写一段利用lstm进行时间序列预测的代码
当然,下面是一个使用 LSTM 模型进行时间序列预测的简单示例代码:
```python
import numpy as np
from keras.models import Sequential
from keras.layers import LSTM, Dense
# 生成示例数据
def generate_data(n):
X = np.arange(n)
y = np.sin(X)
return X, y
# 准备数据
n_samples = 1000
X, y = generate_data(n_samples)
X = X.reshape(n_samples, 1, 1) # 将输入数据转换为 (samples, timesteps, features) 的格式
# 构建 LSTM 模型
model = Sequential()
model.add(LSTM(16, input_shape=(1, 1)))
model.add(Dense(1))
# 编译模型
model.compile(loss='mean_squared_error', optimizer='adam')
# 训练模型
model.fit(X, y, epochs=10, batch_size=32)
# 使用模型进行预测
X_test, y_test = generate_data(10)
X_test = X_test.reshape(10, 1, 1)
predictions = model.predict(X_test)
print(predictions)
```
这段代码中,首先定义了一个生成示例数据的函数 `generate_data`,然后使用该函数生成了示例数据 `X` 和 `y`。接着,将输入数据 `X` 调整为 LSTM 模型所需的格式 `(samples, timesteps, features)`。然后,构建了一个简单的 LSTM 模型,其中包含一个 LSTM 层和一个全连接层。模型使用均方误差作为损失函数,并使用 Adam 优化器进行训练。最后,使用训练好的模型进行预测并打印结果。
请注意,这只是一个简单的示例代码,实际应用中可能需要进行更多的数据预处理、调参等操作。此外,还可以根据具体需求对模型结构进行调整和优化。
阅读全文
相关推荐
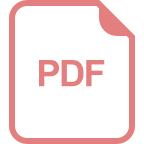
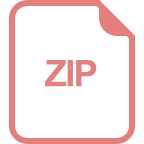
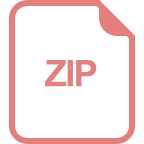













