用python感知机实现逻辑与
时间: 2024-10-19 19:05:03 浏览: 22
在Python中,我们可以使用sklearn库中的Perceptron模型来实现逻辑“与”运算,虽然Perceptron原本是用于二分类任务的线性模型,但它也可以通过适当的阈值调整来进行逻辑门操作。这里是一个简单的示例:
```python
from sklearn.linear_model import Perceptron
import numpy as np
# 定义逻辑与函数的输入输出数据 (0表示False,1表示True)
data = [[0, 0], [0, 1], [1, 0], [1, 1]] # 输入:[x1, x2]
labels = [0, 0, 0, 1] # 输出:y = x1 & x2
# 创建并训练Perceptron模型
perceptron = Perceptron()
perceptron.fit(data, labels)
# 定义逻辑与的预测函数
def logical_and(x):
prediction = perceptron.predict([x])[0]
return int(prediction > 0.5) # 因为Perceptron输出的是概率,所以我们设置阈值为0.5来判断结果
# 测试逻辑与
print(logical_and([1, 0])) # 应该输出0,因为1 AND 0 == 0
print(logical_and([1, 1])) # 应该输出1,因为1 AND 1 == 1
相关问题
用python感知机实现逻辑非
在Python中,我们可以使用scikit-learn库中的Perceptron模型来实现简单的逻辑非功能。逻辑非可以用一个二分类的问题来模拟,其中正样本代表输入为真,负样本代表输入为假。对于逻辑非操作,我们知道A非即是非A,即当输入是True时返回False,输入是False时返回True。
首先,你需要安装scikit-learn库,如果没有安装可以使用pip install scikit-learn命令。下面是使用Perceptron实现逻辑非的一个简单示例:
```python
from sklearn.linear_model import Perceptron
# 定义逻辑非的数据集 (True => False, False => True)
X = [[0], [1]] # 输入特征,这里我们只有一个特征
y = [0, 1] # 输出标签,分别对应False和True
# 创建并训练Perceptron模型
perceptron = Perceptron()
perceptron.fit(X, y)
# 预测新的数据点是否是非逻辑
new_data = [1] # 要测试的逻辑值
prediction = perceptron.predict([new_data])[0]
print(f"逻辑非的结果: {prediction}")
```
运行这个代码后,Perceptron会学习到如何将True映射为False,False映射为True,实现了逻辑非的功能。
用python感知机实现逻辑或
在Python中,我们可以使用机器学习库如scikit-learn来实现感知机(Perceptron),但这通常用于二分类任务而不是逻辑运算。不过,如果你想用简单的线性模型来模拟逻辑“或”操作,一种方法是创建一个两输入的感知机,其中每个输入代表逻辑变量A和B。逻辑“或”可以理解为只要有一个输入为真,结果就为真。
以下是一个简化的例子:
```python
from sklearn.linear_model import Perceptron
import numpy as np
# 定义逻辑或的数据集(0 for False, 1 for True)
data = np.array([[0, 0], [0, 1], [1, 0], [1, 1]])
labels = np.array([0, 1, 1, 1])
# 初始化感知机
perceptron = Perceptron()
# 训练模型
perceptron.fit(data, labels)
# 使用模型预测逻辑“或”
def or_function(A, B):
A_B = np.array([[A, B]])
prediction = perceptron.predict(A_B)
return prediction[0]
# 示例
print(or_function(0, 0)) # 输出:0 (False)
print(or_function(0, 1)) # 输出:1 (True)
```
请注意,这个实现非常基础,并非最佳方法来处理复杂的逻辑运算。在实际应用中,我们通常会使用更专业的逻辑门电路或专用的逻辑运算函数,比如`numpy`库中的`logical_or`。
阅读全文
相关推荐
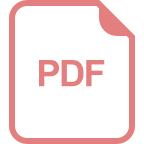
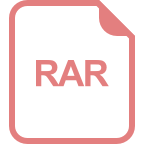
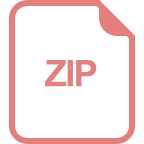
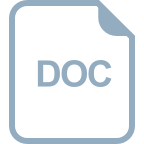




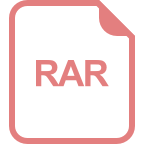
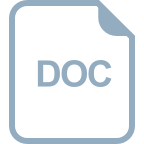
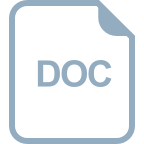
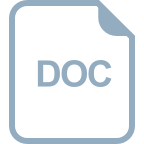



