python遗传算法出下面TSP问题。 附 中国31省会城市坐标为: 1304 2312 3639 1315 4177 2244 3712 1399 3488 1535 3326 1556 3238 1229 4196 1004 4312 790 4386 570 3007 1970 2562 1756 2788 1491 2381 1676 1332 695 3715 1678 3918 2179 4061 2370 3780 2212 3676 2578 4029 2838 4263 2931 3429 1908 3507 2367 3394 2643 3439 3201 2935 3240 3140 3550 2545 2357 2778 2826 2370 2975
时间: 2024-02-10 12:04:25 浏览: 101
好的,这里给出一个简单的遗传算法实现TSP问题的代码示例,基本思路是使用遗传算法生成一组城市访问顺序的解,然后通过交叉、变异等操作来优化这些解,最终找到最优解。
```python
import random
# 定义城市坐标
cities = [(1304, 2312), (3639, 1315), (4177, 2244), (3712, 1399), (3488, 1535),
(3326, 1556), (3238, 1229), (4196, 1004), (4312, 790), (4386, 570),
(3007, 1970), (2562, 1756), (2788, 1491), (2381, 1676), (1332, 695),
(3715, 1678), (3918, 2179), (4061, 2370), (3780, 2212), (3676, 2578),
(4029, 2838), (4263, 2931), (3429, 1908), (3507, 2367), (3394, 2643),
(3439, 3201), (2935, 3240), (3140, 3550), (2545, 2357), (2778, 2826),
(2370, 2975)]
# 定义遗传算法参数
POP_SIZE = 50 # 种群大小
N_GEN = 1000 # 迭代次数
CROSS_RATE = 0.7 # 交叉概率
MUT_RATE = 0.01 # 变异概率
# 计算城市之间的距离
def distance(city1, city2):
return ((city1[0] - city2[0])**2 + (city1[1] - city2[1])**2)**0.5
# 计算一条路径的总长度
def path_length(path):
return sum(distance(cities[path[i]], cities[path[i+1]]) for i in range(len(path)-1))
# 生成初始种群
pop = [list(range(31)) for _ in range(POP_SIZE)]
# 遗传算法迭代
for i in range(N_GEN):
# 计算每个个体的适应度
fitness = [1/path_length(p) for p in pop]
# 选择父代
parents = []
for j in range(POP_SIZE):
p1 = random.choices(pop, weights=fitness)[0]
p2 = random.choices(pop, weights=fitness)[0]
parents.append((p1, p2))
# 交叉操作
for j in range(POP_SIZE):
if random.random() < CROSS_RATE:
# 随机选择交叉点
cross_point = random.randint(0, 30)
# 将两个父代的交叉点之间的城市编号互换
child1 = parents[j][0][:cross_point] + [c for c in parents[j][1] if c not in parents[j][0][:cross_point]]
child2 = parents[j][1][:cross_point] + [c for c in parents[j][0] if c not in parents[j][1][:cross_point]]
# 更新种群
pop[j] = child1
pop[(j+1)%POP_SIZE] = child2
# 变异操作
for j in range(POP_SIZE):
if random.random() < MUT_RATE:
# 随机选择两个城市,并交换它们的位置
mutate_point1, mutate_point2 = random.sample(range(31), 2)
pop[j][mutate_point1], pop[j][mutate_point2] = pop[j][mutate_point2], pop[j][mutate_point1]
# 找到最优解
best_path = min(pop, key=path_length)
print("最优路径:", best_path)
print("路径长度:", path_length(best_path))
```
注:这里使用了 Python 的内置 `random` 模块来实现随机操作,实际上还有更专业的遗传算法库可以使用,例如 DEAP、PyGAD 等。
阅读全文
相关推荐
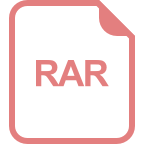
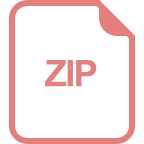
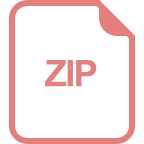
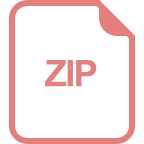
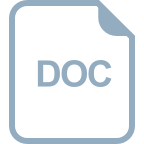
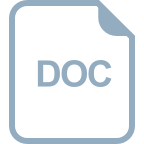
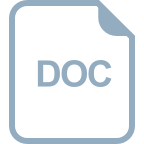

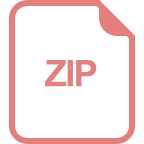
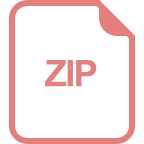
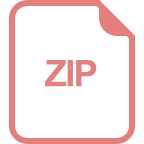
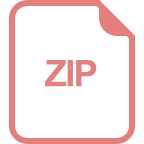
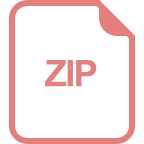
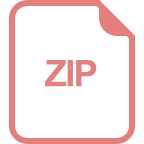
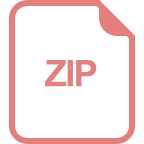
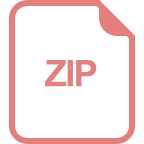