keras bert crt bilstm
时间: 2023-12-03 18:00:39 浏览: 256
Keras是一个高级深度学习库,可用于构建和训练神经网络模型。BERT(Bidirectional Encoder Representations from Transformers)是一种预训练的自然语言处理模型,能够有效地表征文本数据。CRT(Conditional Random Field)是一种用于序列标注任务的概率图模型。BiLSTM(Bidirectional Long Short-Term Memory)是一种能够处理时序数据的循环神经网络模型。
在自然语言处理领域,可以使用Keras来构建一个集成了BERT模型的神经网络,其中可以包括BiLSTM模型作为BERT输出的后续处理器,同时结合CRT模型进行序列标注任务。这样的模型可以在文本分类、命名实体识别、情感分析等任务中发挥作用。
使用Keras作为深度学习库,可以方便地构建出复杂的神经网络模型,同时结合BERT等预训练模型,可以提高模型的性能和效果。BiLSTM模型能够更好地处理时序数据,而CRT模型则可以提高序列标注任务的准确性。综合利用这些模型,可以在自然语言处理任务中取得更好的效果。
总之,Keras、BERT、CRT和BiLSTM是在自然语言处理领域中非常有用的工具和模型,它们可以结合使用,共同构建出高性能的神经网络模型,用于解决文本处理的各种任务。
相关问题
Traceback (most recent call last): File "E:\pycharm-workspace\BERT\BERT-BiLSTM-CRF-NER-master\run.py", line 37, in <module> train_ner() File "E:\pycharm-workspace\BERT\BERT-BiLSTM-CRF-NER-master\run.py", line 24, in train_ner from bert_base.train.bert_lstm_ner import train File "E:\pycharm-workspace\BERT\BERT-BiLSTM-CRF-NER-master\bert_base\train\bert_lstm_ner.py", line 23, in <module> from bert_base.train.models import create_model, InputFeatures, InputExample File "E:\pycharm-workspace\BERT\BERT-BiLSTM-CRF-NER-master\bert_base\train\models.py", line 10, in <module> from bert_base.train.lstm_crf_layer import BLSTM_CRF File "E:\pycharm-workspace\BERT\BERT-BiLSTM-CRF-NER-master\bert_base\train\lstm_crf_layer.py", line 9, in <module> from tensorflow.contrib import rnn ModuleNotFoundError: No module named 'tensorflow.contrib'
这个错误是因为 TensorFlow 2.x 中移除了 `tensorflow.contrib` 模块。在 TensorFlow 2.x 版本中,很多以前位于 `tensorflow.contrib` 的功能被整合到其他模块中或者被废弃,所以您的代码不再兼容 TensorFlow 2.x。
为了解决这个问题,您需要修改您的代码,将 `tensorflow.contrib` 相关的导入语句替换为 TensorFlow 2.x 的等效方式。
具体来说,在您的代码中,将 `bert_base\train\lstm_crf_layer.py` 文件中的以下行:
```python
from tensorflow.contrib import rnn
```
替换为:
```python
import tensorflow as tf
from tensorflow.keras import layers
```
然后,您还需要确保在其他地方使用到 `tensorflow.contrib` 的地方也做相应的修改。
请注意,这只是一个示例,您可能还需要根据您的代码结构和需求做一些适当的修改。确保仔细检查并更新代码中所有相关的 `tensorflow.contrib` 导入语句。
bert-bilstm-attention代码,基于Keras
下面是一个基于Keras的BERT-BiLSTM-Attention代码示例:
```python
import tensorflow as tf
from tensorflow.keras.layers import Input, Dense, Dropout, LSTM, Bidirectional, TimeDistributed, Masking, \
Concatenate, Attention
from transformers import TFBertModel
# 定义模型输入
input_ids = Input(shape=(max_seq_length,), dtype=tf.int32, name='input_ids')
attention_mask = Input(shape=(max_seq_length,), dtype=tf.int32, name='attention_mask')
# 载入预训练的BERT
bert_model = TFBertModel.from_pretrained('bert-base-chinese')
# BERT编码层
output = bert_model(input_ids, attention_mask=attention_mask)[0]
# BiLSTM层
output = Bidirectional(LSTM(128, return_sequences=True))(output)
# Attention层
attention = Attention()([output, output])
# 将BiLSTM和Attention的输出连接起来
output = Concatenate()([output, attention])
# Dropout层
output = Dropout(0.5)(output)
# 全连接层
output = TimeDistributed(Dense(num_tags, activation='softmax'))(output)
# 定义模型
model = tf.keras.models.Model(inputs=[input_ids, attention_mask], outputs=output)
# 编译模型
optimizer = tf.keras.optimizers.Adam(lr=2e-5)
loss = tf.keras.losses.SparseCategoricalCrossentropy(from_logits=True)
metric = tf.keras.metrics.SparseCategoricalAccuracy('accuracy')
model.compile(optimizer=optimizer, loss=loss, metrics=[metric])
```
其中,`max_seq_length`是输入序列的最大长度,`num_tags`是标签的数量。我们使用了`transformers`库来载入预训练的BERT模型,使用了Keras的层来构建BiLSTM和Attention层,最后使用Keras的`Model`类定义整个模型。在编译模型时,我们使用了Adam优化器、交叉熵损失和稀疏分类精度作为评估指标。
阅读全文
相关推荐
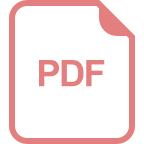
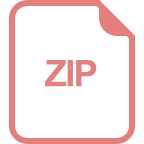
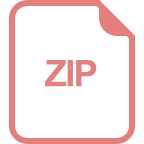
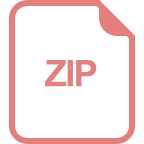
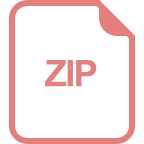
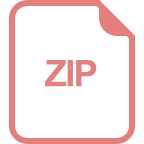
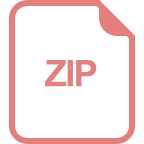
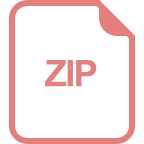
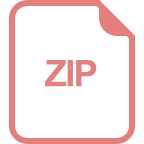
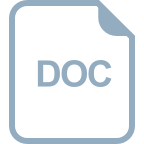
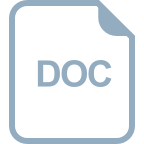
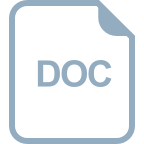
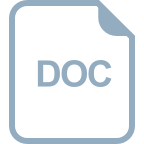


