import torch有红色波浪线
时间: 2023-05-10 07:50:18 浏览: 325
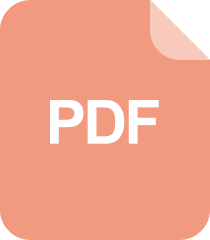
Pycharm中import torch报错的快速解决方法
在使用PyTorch时,有时会发现当引入`import torch`时会出现红色波浪线的情况。这是因为编辑器(如PyCharm)无法自动识别和找到PyTorch库中的模块和函数,因此会给出错误提示。但实际上这并不影响PyTorch的运行和使用。
要解决这个问题,最好的方法是在编辑器中添加PyTorch库的路径。可以通过以下步骤完成:
1. 确认PyTorch的安装路径。可以在终端中输入以下代码查看PyTorch的路径:
`python -c "import torch; print(torch.__file__)"`
2. 将上一步中输出的路径复制。
3. 在PyCharm中,点击 “File” -> “Settings” -> “Project Interpreter”。
4. 找到当前项目所使用的Python环境,并点击右侧的齿轮图标。
5. 选择“Show All…”选项。
6. 找到PyTorch库的路径并将其添加到解释器路径中。
完成上述步骤后,编辑器就可以正确地识别和使用PyTorch库中的模块和函数了。同时,也可以确保代码在运行时能够正确地导入PyTorch库。
阅读全文
相关推荐
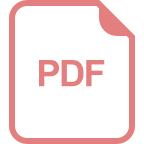














