python在已经有训练集和测试集的情况下绘制随机森林分类模型的学习曲线
时间: 2024-11-13 18:22:28 浏览: 15
在Python中,使用`sklearn`库中的`RandomForestClassifier`绘制学习曲线通常涉及以下几个步骤:
1. **导入所需库**:
```python
import numpy as np
from sklearn.datasets import load_iris
from sklearn.model_selection import train_test_split, learning_curve
from sklearn.ensemble import RandomForestClassifier
import matplotlib.pyplot as plt
```
2. **加载数据集**:
```python
iris = load_iris()
X = iris.data
y = iris.target
```
3. **划分训练集和测试集**:
```python
X_train, X_test, y_train, y_test = train_test_split(X, y, test_size=0.2, random_state=42)
```
4. **创建随机森林分类器并设置参数**:
```python
rf_clf = RandomForestClassifier(random_state=42)
```
5. **计算学习曲线所需的分数**:
```python
train_sizes, train_scores, test_scores = learning_curve(rf_clf, X_train, y_train, cv=5, scoring='accuracy', n_jobs=-1, train_sizes=np.linspace(0.1, 1.0, 10))
```
这里`cv=5`表示交叉验证次数,`n_jobs=-1`用于并行处理。
6. **绘制学习曲线**:
```python
plt.figure(figsize=(10, 6))
plt.plot(train_sizes, train_scores.mean(axis=1), label='Training accuracy')
plt.plot(train_sizes, test_scores.mean(axis=1), label='Cross-validation accuracy')
plt.xlabel('Number of samples')
plt.ylabel('Accuracy')
plt.title('Learning Curve for Random Forest Classifier')
plt.legend()
plt.show()
```
这个图会显示随着训练样本数量增加,模型在训练集和测试集上的性能变化情况。学习曲线可以帮助我们了解模型是否过拟合或欠拟合,并优化模型复杂度。
阅读全文
相关推荐
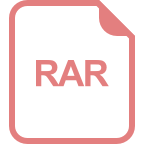
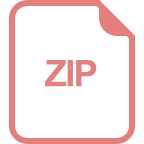
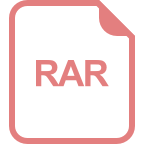

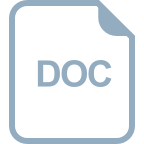
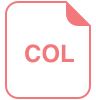
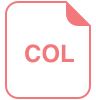
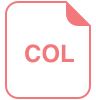
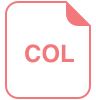
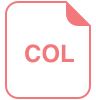
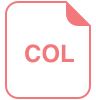







