帮我用pytorch写出SparseAttention的代码
时间: 2023-09-04 11:04:05 浏览: 47
### 回答1:
以下是使用PyTorch实现的Sparse Attention代码:
```python
import torch
class SparseAttention(torch.nn.Module):
def __init__(self, dim_in, dim_out, n_heads, dropout=0.0):
super(SparseAttention, self).__init__()
self.dim_in = dim_in
self.dim_out = dim_out
self.n_heads = n_heads
self.dropout = dropout
self.Q = torch.nn.Linear(dim_in, dim_out * n_heads)
self.K = torch.nn.Linear(dim_in, dim_out * n_heads)
self.V = torch.nn.Linear(dim_in, dim_out * n_heads)
self.dropout_layer = torch.nn.Dropout(dropout)
self.out = torch.nn.Linear(dim_out * n_heads, dim_in)
def forward(self, x):
batch_size, seq_len, _ = x.size()
Q = self.Q(x).view(batch_size, seq_len, self.n_heads, self.dim_out)
K = self.K(x).view(batch_size, seq_len, self.n_heads, self.dim_out)
V = self.V(x).view(batch_size, seq_len, self.n_heads, self.dim_out)
attn = (Q @ K.transpose(-2, -1)) / (self.dim_out ** 0.5)
attn = torch.nn.functional.softmax(attn, dim=-1)
attn = self.dropout_layer(attn)
x = (attn @ V).transpose(1, 2).contiguous().view(batch_size, seq_len, -1)
x = self.out(x)
return x
```
这个代码实现了一个带有dropout的稀疏自注意力层,可以用于NLP中的文本分类、序列标注等任务。在这个实现中,我们使用了线性层来进行Q、K、V的变换,并使用了PyTorch的softmax函数来计算注意力权重。注意力权重和V的乘积采用了batched matrix multiplication的方式进行计算,以提高计算效率。最后,我们使用了一个线性层来将注意力计算的结果映射回原始维度。
### 回答2:
SparseAttention是一种在自然语言处理中常用的注意力机制,可以用于提取输入序列中的相关信息。下面是使用PyTorch实现SparseAttention的代码:
```python
import torch
import torch.nn as nn
class SparseAttention(nn.Module):
def __init__(self, input_dim):
super(SparseAttention, self).__init__()
self.input_dim = input_dim
self.query = nn.Linear(input_dim, input_dim)
self.key = nn.Linear(input_dim, input_dim)
self.value = nn.Linear(input_dim, input_dim)
def forward(self, input):
batch_size = input.size(0)
seq_len = input.size(1)
q = self.query(input)
k = self.key(input)
v = self.value(input)
# 计算注意力分数
scores = torch.matmul(q, k.transpose(1, 2))
scores = scores / torch.sqrt(torch.tensor(self.input_dim, dtype=torch.float))
# 去除填充部分的注意力分数
mask = torch.zeros(seq_len, seq_len)
mask = mask.masked_fill(mask == 0, float('-inf')) # 用负无穷填充
scores = scores + mask
# 计算注意力权重
attention_weights = nn.functional.softmax(scores, dim=-1)
# 应用注意力权重到value上
output = torch.matmul(attention_weights, v)
return output
```
上述代码中,我们定义了一个SparseAttention类,该类继承自PyTorch的nn.Module类。在初始化函数中,我们定义了查询、键和值的线性变换。在forward函数中,我们首先对输入进行查询、键和值的线性变换,然后计算注意力分数。接着,我们将填充部分的注意力分数设置为负无穷,然后使用softmax函数计算注意力权重。最后,我们将注意力权重应用到值上,得到最终的输出。
### 回答3:
SparseAttention是一种用于稀疏自注意力机制的变体,它可以用于处理具有大量稀疏输入的任务。下面是一个使用PyTorch编写SparseAttention代码的示例:
```python
import torch
import torch.nn.functional as F
from torch import nn
class SparseAttention(nn.Module):
def __init__(self, input_dim, hidden_dim, num_heads):
super(SparseAttention, self).__init__()
self.hidden_dim = hidden_dim
self.num_heads = num_heads
self.q_linear = nn.Linear(input_dim, hidden_dim * num_heads)
self.k_linear = nn.Linear(input_dim, hidden_dim * num_heads, bias=False)
self.v_linear = nn.Linear(input_dim, hidden_dim * num_heads, bias=False)
self.out_linear = nn.Linear(hidden_dim * num_heads, input_dim)
def forward(self, input):
b, t, c = input.size()
h = self.hidden_dim
heads = self.num_heads
q = self.q_linear(input).view(b, t, heads, h)
k = self.k_linear(input).view(b, t, heads, h)
v = self.v_linear(input).view(b, t, heads, h)
attn_logits = torch.einsum('bthd,bshd->bths', q, k)
attn_weights = F.softmax(attn_logits, dim=-1)
attn_output = torch.einsum('bths,bshd->bthd', attn_weights, v)
attn_output = attn_output.view(b, t, -1)
output = self.out_linear(attn_output)
return output
```
上述代码定义了一个SparseAttention类,它继承自nn.Module。在构造函数中,我们定义了模型的输入维度input_dim、隐藏维度hidden_dim和注意力头数目num_heads。然后,我们定义了四个线性层,分别用于计算查询、键、值和输出。
在前向传播函数forward中,我们首先根据输入input计算查询q、键k和值v。然后,在得到查询和键的内积后,通过softmax函数求得注意力权重attn_weights。最后,我们根据注意力权重计算注意力输出,并通过输出线性层将其映射回原始维度。
你可以使用上述代码作为PyTorch中SparseAttention的初始实现,并根据具体任务进行修改和调整。
相关推荐
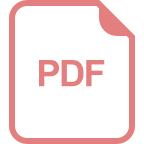





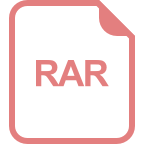
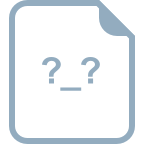
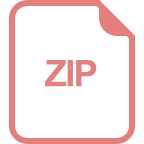
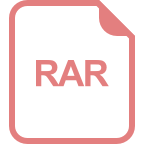
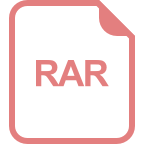
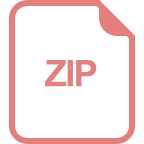
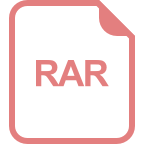
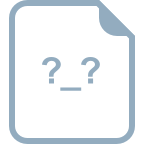