生成代码:用jieba分词进行文本预处理,使用自定义词典
时间: 2024-05-25 21:17:42 浏览: 16
import jieba
# 加载自定义词典
jieba.load_userdict('custom_dict.txt')
# 文本预处理
def text_preprocessing(text):
# 分词
seg_list = jieba.cut(text)
# 去除停用词等无用词汇
filtered_words = []
for word in seg_list:
if word.strip() and word not in stopwords_list:
filtered_words.append(word)
# 返回处理后的文本
return ' '.join(filtered_words)
相关问题
生成代码:用jieba分词进行文本预处理
以下是用 Python 语言和 jieba 库进行文本预处理的示例代码:
```python
import jieba
# 读取文本
with open('example.txt', 'r', encoding='utf-8') as f:
text = f.read()
# 分词
words = jieba.cut(text)
# 去除停用词
stop_words = ['的', '了', '在', '是', '我', '你', '他', '她', '它', '我们', '你们', '他们', '她们', '它们']
filtered_words = [word for word in words if word not in stop_words]
# 输出结果
print(filtered_words)
```
这段代码的主要步骤如下:
1. 读取文本(假设文件名为 example.txt)。
2. 用 jieba.cut() 函数对文本进行分词。
3. 去除停用词,这里只是举例了一些常见的停用词,具体应该根据实际情况进行选择和扩充。
4. 输出处理后的结果。
需要注意的是,jieba.cut() 函数的返回值是一个生成器对象,需要用 for 循环或者转换成列表等形式才能进行后续处理。另外,jieba 默认使用精确模式进行分词,也可以设置为其他模式或自定义词典等。
数据预处理中使用中文文本分词技术的操作步骤
中文文本分词是自然语言处理中的一个重要环节,可以将一段连续的中文文本切分成一个个有意义的词语,为后续的文本处理提供基础。下面是使用jieba分词工具进行中文文本分词的操作步骤:
1. 安装jieba分词工具
可以使用pip命令进行安装:`pip install jieba`
2. 导入jieba分词工具
在Python代码中导入jieba分词工具:`import jieba`
3. 加载停用词
停用词是指在文本分析中不需要进行处理的词语,如“的”、“是”等常用词语,可以在分词前进行过滤。可以先将停用词保存在一个文件中,然后在代码中读取并进行加载。
4. 加载自定义词典
jieba分词工具提供了用户自定义词典的功能,可以通过加载自定义词典来增加分词的准确性。可以将自定义词典保存在一个文件中,然后在代码中读取并进行加载。
5. 对文本进行分词
使用jieba分词工具的`jieba.cut()`函数对文本进行分词,函数返回一个生成器对象,可以通过遍历生成器对象获取分词结果。可以使用不同的分词模式进行分词,如精确模式、全模式、搜索引擎模式等。
6. 进行词性标注
jieba分词工具还提供了词性标注的功能,可以对分词结果进行词性标注,如名词、动词、形容词等。
7. 进行去重、去停用词等处理
对于分词结果,可以进行去重、去停用词等处理,以获取更加准确的分词结果。
以上是使用jieba分词工具进行中文文本分词的基本操作步骤,需要根据具体的任务需求进行相应的参数设置和处理。
相关推荐
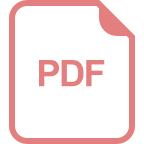
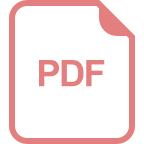
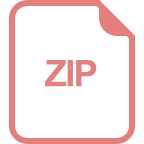
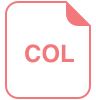
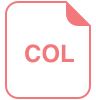
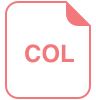
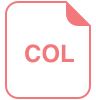
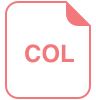







