mc dropout
时间: 2024-03-11 08:41:30 浏览: 21
MC Dropout是一种基于蒙特卡洛方法的正则化技术,用于在神经网络训练过程中减少过拟合现象。它通过在训练过程中随机丢弃一部分神经元的输出来引入随机性,从而增加模型的鲁棒性和泛化能力。
具体来说,MC Dropout在每个训练样本的前向传播过程中,以一定的概率(通常为0.5)随机丢弃一部分神经元的输出。这样做的效果是,每次前向传播时都会得到不同的输出,相当于从模型的不同子集中采样。在训练过程中,通过多次前向传播和反向传播的迭代,MC Dropout可以使模型学习到更多的不同子集之间的共享特征,从而提高模型的泛化能力。
在测试阶段,为了得到更可靠的预测结果,通常会进行多次前向传播,并对多次预测结果进行平均或投票。这样可以减少模型预测的不确定性,并提高模型的鲁棒性。
总结来说,MC Dropout通过随机丢弃神经元的输出,在训练过程中引入噪声,从而增加模型的泛化能力和鲁棒性。
相关问题
MC dropout
MC dropout是一种使用dropout来训练模型并在预测时估计模型的不确定度的方法。在MC dropout中,模型的参数可以看成是服从一个伯努利分布,通过在预测时多次进行预测并取平均值来得到最终的预测结果。通过计算预测结果的平均值,可以得到模型的方差,从而估计深度学习的不确定度。MC dropout也被称为贝叶斯神经网络。
bert dropout
BERT Dropout是一种在BERT模型中应用的正则化技术,用于防止过拟合和提高模型的泛化能力。在BERT中,Dropout被应用于Transformer的多头自注意力机制和前馈神经网络中。
具体来说,BERT Dropout通过在训练过程中随机将一部分神经元的输出置为0来实现。这样做的好处是,它可以迫使模型不依赖于某些特定的输入特征,从而增加模型对不同输入的鲁棒性。
在BERT中,通常会在Transformer的每个子层中应用Dropout。具体来说,对于每个子层的输出,都会独立地随机选择一些神经元,并将它们的输出置为0。然后,在进行下一层的计算之前,会将剩余的神经元的输出进行缩放,以保持总体输出的期望值不变。
通过使用BERT Dropout,可以有效地减少模型的过拟合风险,并提高模型在未见过的数据上的性能。
相关推荐
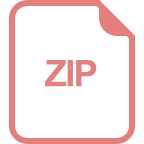
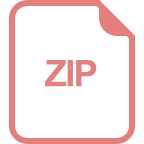












