MC-BERT表征文本的代码
时间: 2024-04-14 22:08:21 浏览: 191
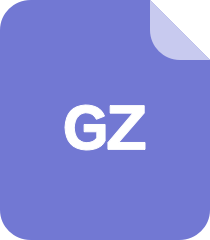
一行代码使用BERT生成句向量,BERT做文本分类、文本相似度计算

以下是使用PyTorch实现MC-BERT模型的示例代码:
```python
import torch
import torch.nn as nn
from transformers import BertModel, BertTokenizer
class MCBert(nn.Module):
def __init__(self, bert_config):
super(MCBert, self).__init__()
self.bert = BertModel.from_pretrained('bert-base-uncased', config=bert_config)
self.dropout = nn.Dropout(bert_config.hidden_dropout_prob)
self.classifier = nn.Linear(bert_config.hidden_size, 1)
def forward(self, input_ids, token_type_ids=None, attention_mask=None):
outputs = self.bert(input_ids, token_type_ids=token_type_ids, attention_mask=attention_mask)
pooled_output = outputs[1]
pooled_output = self.dropout(pooled_output)
logits = self.classifier(pooled_output)
return logits
# 加载预训练模型和词汇表
tokenizer = BertTokenizer.from_pretrained('bert-base-uncased')
config = BertConfig.from_pretrained('bert-base-uncased')
model = MCBert(config)
# 输入文本
text = "Hello, how are you doing today?"
# 将文本转换为输入特征
input_ids = tokenizer.encode(text, add_special_tokens=True)
attention_mask = [1] * len(input_ids)
# 将输入特征传入模型
logits = model(torch.tensor([input_ids]), attention_mask=torch.tensor([attention_mask]))
# 打印输出
print(logits)
```
在上面的示例代码中,我们首先定义了一个MC-BERT模型,包括一个BERT模型、一个dropout层和一个线性分类器。然后,我们加载了BERT的预训练模型和词汇表,并使用tokenizer将输入文本转换为输入特征。最后,我们将输入特征传入模型,并打印输出。
阅读全文
相关推荐
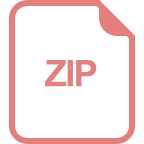
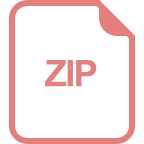

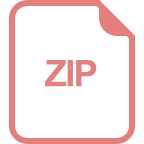
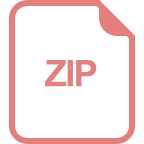
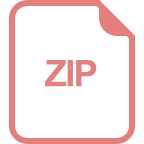
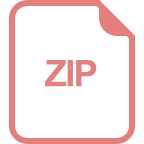
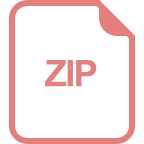
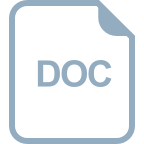
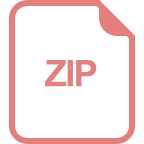
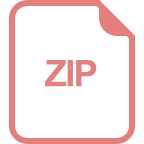
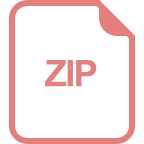
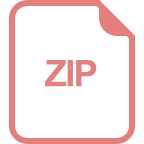
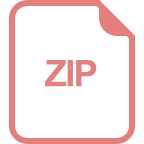
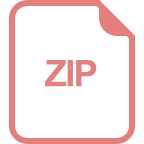
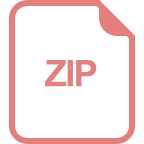
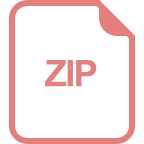