if use_bottleneck == True: self.bottleneck = nn.Sequential( nn.Linear(n_hiddens[-1], bottleneck_width), nn.Linear(bottleneck_width, bottleneck_width), nn.BatchNorm1d(bottleneck_width), nn.ReLU(), nn.Dropout(), ) self.bottleneck[0].weight.data.normal_(0, 0.005) self.bottleneck[0].bias.data.fill_(0.1) self.bottleneck[1].weight.data.normal_(0, 0.005) self.bottleneck[1].bias.data.fill_(0.1) self.fc = nn.Linear(bottleneck_width, n_output) torch.nn.init.xavier_normal_(self.fc.weight) else: self.fc_out = nn.Linear(n_hiddens[-1], self.n_output)
时间: 2024-02-14 20:28:35 浏览: 88
这段代码是在模型中添加bottleneck层和全连接层。如果use_bottleneck为True,则会创建一个包含线性层、批归一化层、激活函数层和Dropout层的Sequential模块,并将其赋值给self.bottleneck。同时,还会创建一个线性层self.fc用于最终的预测。
在创建bottleneck层时,使用nn.Linear函数定义了两个线性层,输入维度为n_hiddens[-1],输出维度为bottleneck_width。然后,使用nn.BatchNorm1d对输出进行批归一化,使用nn.ReLU作为激活函数,使用nn.Dropout进行随机失活。
接下来,通过.data属性设置权重和偏置的初始值。权重初始化为服从均值为0、标准差为0.005的正态分布,偏置初始化为常数0.1。
如果use_bottleneck为False,则直接创建一个线性层self.fc_out,输入维度为n_hiddens[-1],输出维度为n_output。
无论使用bottleneck还是直接使用全连接层,最后都会进行权重初始化。对于使用bottleneck的模型,使用torch.nn.init.xavier_normal_函数对self.fc的权重进行Xavier正态分布初始化。
相关问题
解释这段代码if use_bottleneck == True: self.bottleneck = nn.Sequential( nn.Linear(n_hiddens[-1], bottleneck_width), nn.Linear(bottleneck_width, bottleneck_width), nn.BatchNorm1d(bottleneck_width), nn.ReLU(), nn.Dropout(), ) self.bottleneck[0].weight.data.normal_(0, 0.005) self.bottleneck[0].bias.data.fill_(0.1) self.bottleneck[1].weight.data.normal_(0, 0.005) self.bottleneck[1].bias.data.fill_(0.1) self.fc = nn.Linear(bottleneck_width, n_output) torch.nn.init.xavier_normal_(self.fc.weight) else: self.fc_out = nn.Linear(n_hiddens[-1], self.n_output)
这段代码是一个条件语句,用于根据use_bottleneck的值来创建模型中的不同层。
如果use_bottleneck为True,代码块中的内容将被执行。首先,创建一个nn.Sequential的模块self.bottleneck,该模块按顺序包含了几个层:
- nn.Linear(n_hiddens[-1], bottleneck_width):创建一个线性层,输入维度为n_hiddens[-1],输出维度为bottleneck_width。
- nn.Linear(bottleneck_width, bottleneck_width):创建另一个线性层,输入和输出维度均为bottleneck_width。
- nn.BatchNorm1d(bottleneck_width):创建一个批归一化层,应用于bottleneck_width维度的输入。
- nn.ReLU():创建一个ReLU激活函数层。
- nn.Dropout():创建一个随机失活层。
然后,使用.data属性为self.bottleneck中的第一个线性层设置权重和偏置的初始值。权重初始化为服从均值为0、标准差为0.005的正态分布,偏置初始化为常数0.1。同样地,对于第二个线性层,也进行相同的操作。
接下来,创建一个线性层self.fc,输入维度为bottleneck_width,输出维度为n_output。使用torch.nn.init.xavier_normal_函数对self.fc的权重进行Xavier正态分布初始化。
如果use_bottleneck为False,则执行else语句块。在这个块中,创建一个线性层self.fc_out,输入维度为n_hiddens[-1],输出维度为self.n_output。
这段代码实现了根据use_bottleneck的值选择不同的层结构,从而构建不同的模型。
class EnhancedResidual(nn.Module): def init(self,in_c,out_c,fm_sz,net_type = 'ta'): super(EnhancedResidual,self).init() self.net_type = net_type self.conv1 = nn.Sequential( nn.Conv2d(in_channels = in_c,out_channels = in_c,kernel_size = 3,padding = 1), nn.BatchNorm2d(in_c), nn.ReLU(), ) self.conv2 = nn.Sequential( nn.Conv2d(in_channels = in_c,out_channels = out_c,kernel_size = 3,padding = 1), nn.BatchNorm2d(out_c), nn.ReLU(), ) self.botneck = nn.Conv2d(in_channels = in_c,out_channels = out_c,kernel_size = 1) self.pool = nn.MaxPool2d(kernel_size = 2,stride = 2) if net_type == 'ta': self.spa = SpatialAttention() self.ca = ChannelAttention(in_planes = in_c,ratio = in_c) self.sa = MultiHeadSelfAttention(in_c = in_c,out_c = in_c // 4,head_n = 4,fm_sz = fm_sz) elif net_type == 'sa': self.sa = MultiHeadSelfAttention(in_c = in_c,out_c = out_c // 4,head_n = 4,fm_sz = fm_sz) elif net_type == 'cbam': self.spa = SpatialAttention() self.ca = ChannelAttention(in_planes = in_c,ratio = in_c) def forward(self,x): x0 = self.botneck(x) x = self.conv1(x) if self.net_type == 'sa': x = self.sa(x) #x = self.conv2(x) elif self.net_type == 'cbam': x = self.ca(x) * x x = self.spa(x) * x x = self.conv2(x) elif self.net_type == 'ta': x = self.ca(x) * x x = self.spa(x) * x x = self.sa(x) x = self.conv2(x) x = x + x0 x = self.pool(x) return x 改写为tensorflow形式
import tensorflow as tf
class EnhancedResidual(tf.keras.layers.Layer):
def __init__(self, in_c, out_c, fm_sz, net_type='ta', **kwargs):
super(EnhancedResidual, self).__init__(**kwargs)
self.net_type = net_type
self.conv1 = tf.keras.Sequential([
tf.keras.layers.Conv2D(filters=in_c, kernel_size=3, padding='same'),
tf.keras.layers.BatchNormalization(),
tf.keras.layers.ReLU()
])
self.conv2 = tf.keras.Sequential([
tf.keras.layers.Conv2D(filters=out_c, kernel_size=3, padding='same'),
tf.keras.layers.BatchNormalization(),
tf.keras.layers.ReLU(),
])
self.botneck = tf.keras.layers.Conv2D(filters=out_c, kernel_size=1)
self.pool = tf.keras.layers.MaxPool2D(pool_size=2, strides=2)
if net_type == 'ta':
self.spa = SpatialAttention()
self.ca = ChannelAttention(in_planes=in_c, ratio=in_c)
self.sa = MultiHeadSelfAttention(in_c=in_c, out_c=in_c // 4, head_n=4, fm_sz=fm_sz)
elif net_type == 'sa':
self.sa = MultiHeadSelfAttention(in_c=in_c, out_c=out_c // 4, head_n=4, fm_sz=fm_sz)
elif net_type == 'cbam':
self.spa = SpatialAttention()
self.ca = ChannelAttention(in_planes=in_c, ratio=in_c)
def call(self, x):
x0 = self.botneck(x)
x = self.conv1(x)
if self.net_type == 'sa':
x = self.sa(x)
# x = self.conv2(x)
elif self.net_type == 'cbam':
x = self.ca(x) * x
x = self.spa(x) * x
x = self.conv2(x)
elif self.net_type == 'ta':
x = self.ca(x) * x
x = self.spa(x) * x
x = self.sa(x)
x = self.conv2(x)
x = x + x0
x = self.pool(x)
return x
阅读全文
相关推荐
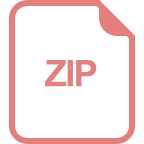
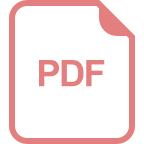
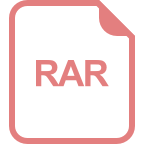














