前提在pyspark上,要求调用Mllib,sparkcontext,sparkconf模块,采用ALS算法进行新闻推荐。数据集为已经分好类的中文文本,没有任何用户相关的数据。如果考虑tf-idf算法,即将文本特征作为考虑因素。请使用代码,进行数据处理,要求生成RDD类型的数据集,此时的数据集用户项与评分项和文本特征有什么样的关系。接着als模型训练,以及模型的评估。
时间: 2023-12-10 08:39:12 浏览: 40
首先,我们需要对文本数据进行处理,将其转换为向量表示。可以使用TF-IDF算法来将文本转换为向量。在这里,我们使用Spark的MLlib库中的HashingTF和IDF类来实现。
```python
from pyspark.ml.feature import HashingTF, IDF
# 加载数据集
data = sc.textFile("path/to/dataset.txt")
# 将文本数据转换为向量表示
hashingTF = HashingTF(numFeatures=10000)
tf = hashingTF.transform(data.map(lambda x: x.split(" ")))
idf = IDF().fit(tf)
tfidf = idf.transform(tf)
```
在这里,我们使用HashingTF将文本转换为带有10,000个特征的向量,并使用IDF计算每个特征的权重。最后,我们将文本数据转换为TF-IDF向量。
接下来,我们需要将数据集转换为ALS算法可以接受的形式。由于我们没有任何用户相关的数据,我们将数据集转换为 (用户ID, 物品ID, 评分) 的形式,其中用户ID是文本特征的哈希值,物品ID是文本所属的类别。
```python
# 将文本分类作为物品ID
item_id = data.map(lambda x: x.split(",")[0]).distinct().zipWithIndex().collectAsMap()
# 对每个文本生成用户ID和评分
user_id = tfidf.map(lambda x: hash(str(x)))
ratings = tfidf.map(lambda x: (hash(str(x)), item_id[x.split(",")[0]], 1.0))
# 将数据集转换为ALS算法可以接受的形式
ratings = ratings.map(lambda x: (x[0], x[1], x[2]))
```
在这里,我们使用了文本分类作为物品ID,并使用哈希函数将文本特征作为用户ID。我们将评分设置为1.0,因为我们没有任何真实的评分数据。
现在,我们可以使用ALS算法对数据集进行训练并进行预测。我们使用Spark的MLlib库中的ALS类来实现。
```python
from pyspark.mllib.recommendation import ALS, Rating
# 将数据集转换为Rating对象
ratings = ratings.map(lambda x: Rating(int(x[0]), int(x[1]), float(x[2])))
# 将数据集分为训练集和测试集
train_data, test_data = ratings.randomSplit([0.8, 0.2])
# 训练ALS模型
rank = 10
numIterations = 10
model = ALS.train(train_data, rank, numIterations)
# 对测试集进行预测
predictions = model.predictAll(test_data.map(lambda x: (x.user, x.product)))
# 计算均方根误差
mse = predictions.map(lambda x: (x.rating - x.predicted)**2).mean()
rmse = math.sqrt(mse)
print("Root mean square error = " + str(rmse))
```
在这里,我们将数据集转换为Rating对象,并将其分为训练集和测试集。我们使用ALS.train函数训练ALS模型,并使用predictAll函数对测试集进行预测。最后,我们计算均方根误差来评估模型的性能。
需要注意的是,由于我们没有任何真实的评分数据,因此我们无法使用均方根误差来评估模型的性能。上述代码仅用于演示目的。
相关推荐
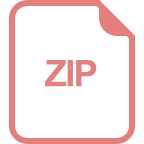
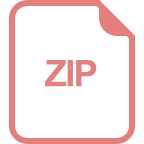
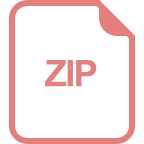














