tf.keras.layers.Dense(int(channel / r))(squeeze)
时间: 2024-05-19 11:12:01 浏览: 10
这行代码是使用 Keras 中的 Dense 层对输入进行全连接操作,输出维度为 channel / r。其中,channel 是输入张量的通道数,r 是一个超参数,用于控制输出维度的缩小比例。这一行代码可以在模型中使用 Squeeze-and-Excitation 模块,用于自适应地调整不同通道的权重。具体来说,它将输入张量进行全局平均池化,然后通过两个全连接层生成一个向量,最后通过 softmax 函数将这个向量转换为权重系数。这些权重系数用于加权输入张量的不同通道,以提高模型的表达能力。
相关问题
# 最佳权重保存路径 BEST_MODEL_PATH = './best_model.h5'
好的,你可以将最佳权重保存路径添加到代码中,如下所示:
```python
import numpy as np
import tensorflow as tf
import os
# 加载数据集
with open('poems.txt', 'r', encoding='utf-8') as f:
data = f.read()
# 构建词典
vocab = sorted(set(data))
char2idx = {char: idx for idx, char in enumerate(vocab)}
idx2char = np.array(vocab)
# 将文本数据转换为数字
text_as_int = np.array([char2idx[c] for c in data])
# 定义训练数据和标签
seq_length = 100
examples_per_epoch = len(data) // (seq_length + 1)
char_dataset = tf.data.Dataset.from_tensor_slices(text_as_int)
sequences = char_dataset.batch(seq_length + 1, drop_remainder=True)
def split_input_target(chunk):
input_text = chunk[:-1]
target_text = chunk[1:]
return input_text, target_text
dataset = sequences.map(split_input_target)
BATCH_SIZE = 128
BUFFER_SIZE = 10000
dataset = dataset.shuffle(BUFFER_SIZE).batch(BATCH_SIZE, drop_remainder=True)
# 构建模型
vocab_size = len(vocab)
embedding_dim = 256
rnn_units = 1024
def build_model(vocab_size, embedding_dim, rnn_units, batch_size):
model = tf.keras.Sequential([
tf.keras.layers.Embedding(vocab_size, embedding_dim,
batch_input_shape=[batch_size, None]),
tf.keras.layers.GRU(rnn_units,
return_sequences=True,
stateful=True,
recurrent_initializer='glorot_uniform'),
tf.keras.layers.Dense(vocab_size)
])
return model
model = build_model(
vocab_size=len(vocab),
embedding_dim=embedding_dim,
rnn_units=rnn_units,
batch_size=BATCH_SIZE)
# 定义损失函数
def loss(labels, logits):
return tf.keras.losses.sparse_categorical_crossentropy(labels, logits, from_logits=True)
# 编译模型
model.compile(optimizer='adam', loss=loss)
# 定义检查点
checkpoint_dir = './training_checkpoints'
checkpoint_prefix = os.path.join(checkpoint_dir, "ckpt_{epoch}")
checkpoint_callback=tf.keras.callbacks.ModelCheckpoint(
filepath=checkpoint_prefix,
save_weights_only=True)
# 定义最佳权重检查点
BEST_MODEL_PATH = './best_model.h5'
best_checkpoint = tf.keras.callbacks.ModelCheckpoint(BEST_MODEL_PATH,
monitor='val_loss',
save_best_only=True,
mode='min',
save_weights_only=True)
# 训练模型
EPOCHS = 50
history = model.fit(dataset, epochs=EPOCHS, callbacks=[checkpoint_callback, best_checkpoint])
# 生成诗歌
def generate_text(model, start_string):
num_generate = 100
input_eval = [char2idx[s] for s in start_string]
input_eval = tf.expand_dims(input_eval, 0)
text_generated = []
temperature = 1.0
model.reset_states()
for i in range(num_generate):
predictions = model(input_eval)
predictions = tf.squeeze(predictions, 0)
predictions = predictions / temperature
predicted_id = tf.random.categorical(predictions, num_samples=1)[-1,0].numpy()
input_eval = tf.expand_dims([predicted_id], 0)
text_generated.append(idx2char[predicted_id])
return (start_string + ''.join(text_generated))
# 加载检查点
model = build_model(vocab_size, embedding_dim, rnn_units, batch_size=1)
model.load_weights(BEST_MODEL_PATH)
model.build(tf.TensorShape([1, None]))
# 生成一首诗
print(generate_text(model, start_string=u"山中"))
```
现在,模型将保存最佳的权重到文件 `best_model.h5`。
SqueezeNet在tensorflow下的python代码实现一个实例
下面是使用TensorFlow实现SqueezeNet的一个简单示例代码:
```python
import tensorflow as tf
def fire_module(x, squeeze=16, expand=64):
# Squeeze layer
s = tf.keras.layers.Conv2D(squeeze, (1, 1), activation='relu')(x)
# Expand layer
e1 = tf.keras.layers.Conv2D(expand, (1, 1), activation='relu')(s)
e3 = tf.keras.layers.Conv2D(expand, (3, 3), padding='same', activation='relu')(s)
# Concatenate expand layers
output = tf.keras.layers.concatenate([e1, e3], axis=3)
return output
def SqueezeNet(input_shape=(224, 224, 3), classes=1000):
input = tf.keras.layers.Input(shape=input_shape)
x = tf.keras.layers.Conv2D(64, (3, 3), strides=(2, 2), padding='valid', activation='relu')(input)
x = tf.keras.layers.MaxPooling2D(pool_size=(3, 3), strides=(2, 2))(x)
x = fire_module(x, squeeze=16, expand=64)
x = fire_module(x, squeeze=16, expand=64)
x = tf.keras.layers.MaxPooling2D(pool_size=(3, 3), strides=(2, 2))(x)
x = fire_module(x, squeeze=32, expand=128)
x = fire_module(x, squeeze=32, expand=128)
x = tf.keras.layers.MaxPooling2D(pool_size=(3, 3), strides=(2, 2))(x)
x = fire_module(x, squeeze=48, expand=192)
x = fire_module(x, squeeze=48, expand=192)
x = fire_module(x, squeeze=64, expand=256)
x = fire_module(x, squeeze=64, expand=256)
x = tf.keras.layers.Dropout(0.5)(x)
x = tf.keras.layers.Conv2D(classes, (1, 1), padding='valid')(x)
x = tf.keras.layers.Flatten()(x)
output = tf.keras.layers.Dense(classes, activation='softmax')(x)
model = tf.keras.models.Model(input, output, name='SqueezeNet')
return model
```
同样,这里也定义了`fire_module`函数,然后通过堆叠多个“Fire模块”和池化层来构建整个SqueezeNet模型。最后,添加一个Dropout层和全连接层来输出分类结果。
相关推荐
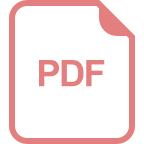
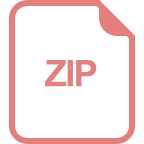
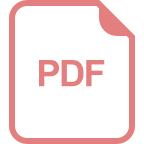












