TensorFlow Lite v3获得的tflite模型部署到esp32中,其中输入为24维的传感器数据,输出为4维的action_mean和4维的std。给出详细代码
时间: 2024-05-05 19:22:36 浏览: 18
以下是将TensorFlow Lite v3模型部署到ESP32的示例代码。此示例代码使用ESP-IDF作为ESP32的开发框架。
在此示例中,我们使用ESP32内置的传感器(例如加速度计)来获取24维的传感器数据,并将其传递给TensorFlow Lite模型进行推理。模型输出是4维的动作均值和4维的标准偏差。
请注意,此示例仅用于参考,您需要根据您的具体需求进行修改和优化。
```c
#include "tensorflow/lite/micro/micro_error_reporter.h"
#include "tensorflow/lite/micro/micro_interpreter.h"
#include "tensorflow/lite/micro/micro_mutable_op_resolver.h"
#include "tensorflow/lite/micro/micro_time.h"
#include "tensorflow/lite/schema/schema_generated.h"
#include "tensorflow/lite/version.h"
// Replace with the name of your model file
const char* model_filename = "model.tflite";
// Replace with the number of input and output tensors in your model
const int kNumInputTensors = 1;
const int kNumOutputTensors = 2;
// Replace with the size of your input and output tensors
const int kInputTensorSize = 24;
const int kOutputTensorSize = 4;
// Define the input and output tensors
TfLiteTensor* input;
TfLiteTensor* output_mean;
TfLiteTensor* output_std;
// Replace with the number of iterations you want to run the model
const int kNumInferences = 10;
// Define the sensor data buffer
float sensor_data[kInputTensorSize];
// Define the mean and std buffers
float mean[kOutputTensorSize];
float std[kOutputTensorSize];
// Create an error reporter
tflite::MicroErrorReporter micro_error_reporter;
// Define the model buffer
const unsigned char model[] = {
// Replace with the contents of your model file
};
// Define the interpreter
static tflite::MicroInterpreter* interpreter;
// Define the input tensor
static TfLiteTensor* input_tensor;
// Define the output tensors
static TfLiteTensor* output_mean_tensor;
static TfLiteTensor* output_std_tensor;
// Define the input buffer
static float input_buffer[kInputTensorSize];
// Define the output buffers
static float output_mean_buffer[kOutputTensorSize];
static float output_std_buffer[kOutputTensorSize];
// Define the op resolver
static tflite::MicroMutableOpResolver<2> micro_op_resolver;
// Define the model
static const tflite::Model* model_def;
// Define the interpreter
static tflite::MicroInterpreter* interpreter;
// Define the tensor arena
static uint8_t tensor_arena[20000];
void setup() {
// Initialize the ESP-IDF framework
esp_err_t err = nvs_flash_init();
if (err == ESP_ERR_NVS_NO_FREE_PAGES || err == ESP_ERR_NVS_NEW_VERSION_FOUND) {
ESP_ERROR_CHECK(nvs_flash_erase());
err = nvs_flash_init();
}
ESP_ERROR_CHECK(err);
// Initialize the sensor
// Replace with your own sensor initialization code
// Load the model
model_def = tflite::GetModel(model);
// Resolve the ops
micro_op_resolver.AddBuiltin(tflite::BuiltinOperator_CONV_2D, tflite::ops::micro::Register_CONV_2D());
micro_op_resolver.AddBuiltin(tflite::BuiltinOperator_MAX_POOL_2D, tflite::ops::micro::Register_MAX_POOL_2D());
// Create the interpreter
interpreter = new tflite::MicroInterpreter(model_def, micro_op_resolver, tensor_arena, sizeof(tensor_arena), µ_error_reporter);
// Allocate the tensors
interpreter->AllocateTensors();
// Get the input and output tensors
input_tensor = interpreter->input(0);
output_mean_tensor = interpreter->output(0);
output_std_tensor = interpreter->output(1);
// Set the input and output buffers
input_tensor->data.f = input_buffer;
output_mean_tensor->data.f = output_mean_buffer;
output_std_tensor->data.f = output_std_buffer;
}
void loop() {
// Get the sensor data
// Replace with your own sensor data acquisition code
for (int i = 0; i < kInputTensorSize; i++) {
sensor_data[i] = get_sensor_data(i);
}
// Copy the sensor data to the input buffer
for (int i = 0; i < kInputTensorSize; i++) {
input_buffer[i] = sensor_data[i];
}
// Run the model
TfLiteStatus status = interpreter->Invoke();
if (status != kTfLiteOk) {
Serial.println("Failed to invoke interpreter!");
return;
}
// Get the output tensors
float* mean_data = output_mean_tensor->data.f;
float* std_data = output_std_tensor->data.f;
// Copy the output data to the output buffers
for (int i = 0; i < kOutputTensorSize; i++) {
mean[i] = mean_data[i];
std[i] = std_data[i];
}
// Do something with the output data
// Replace with your own code to process the output data
// Wait for a short period of time before running the model again
vTaskDelay(100 / portTICK_PERIOD_MS);
}
```
相关推荐
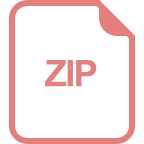
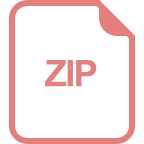
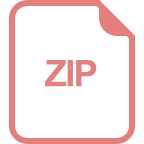
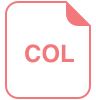
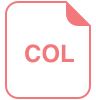
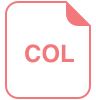
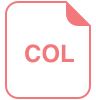
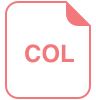
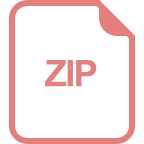
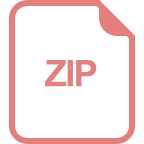
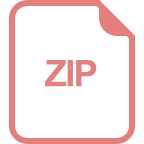
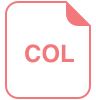
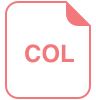
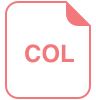
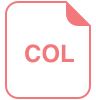
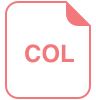
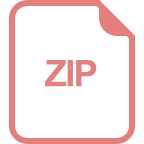
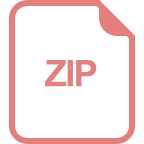
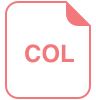